A 40-nm 646.6TOPS/W Sparsity-Scaling DNN Processor for On-Device Training.
2022 IEEE Symposium on VLSI Technology and Circuits (VLSI Technology and Circuits)(2022)
摘要
This work presents the first deep-neural-network (DNN) processor that supports sparsity-scaling training (SST). SST enables a sparsity of 92.4-to-97.8% with a <1.84% accuracy loss on commonly used neural networks (including ResNet and VGG). Compact 8-bit block floating-point (BFP8) is employed and external memory access (EMA) is minimized by bidirectional data compression. The chip delivers the maximum energy efficiency of 646.6TOPS/W, achieving 3.7× and 4.9× improvements in energy and area efficiencies, respectively, when compared to the state-of-the-art designs.
更多查看译文
关键词
bidirectional data compression,maximum energy efficiency,area efficiencies,on-device training,deep-neural-network processor,DNN,sparsity-scaling training,external memory access,sparsity-scaling DNN processor,EMA,SST,compact block floating-point,word length 8 bit,size 40 nm
AI 理解论文
溯源树
样例
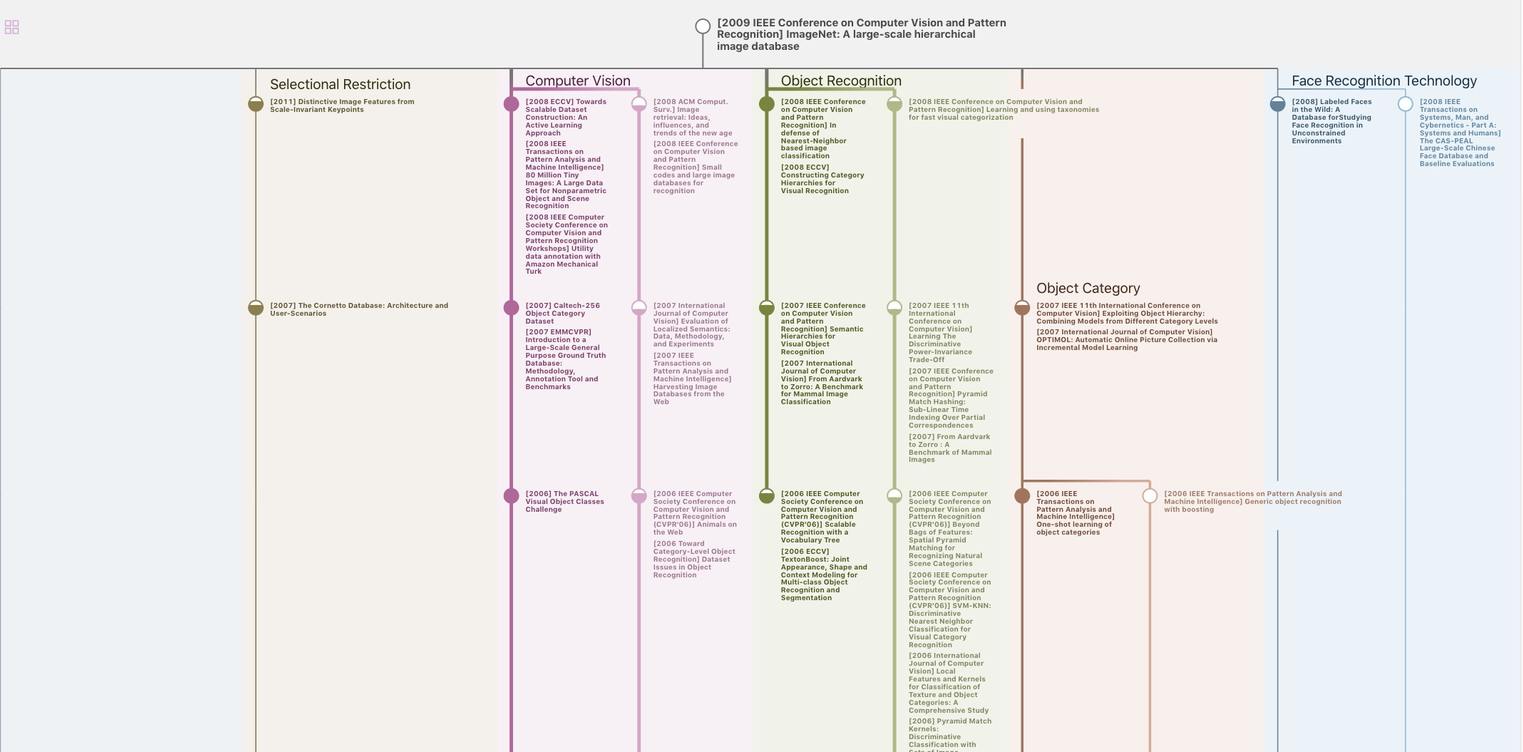
生成溯源树,研究论文发展脉络
Chat Paper
正在生成论文摘要