An event driven approximate bio-electrical model generating surface electromyography RMS features
2022 35th International Conference on VLSI Design and 2022 21st International Conference on Embedded Systems (VLSID)(2022)
摘要
Surface electromyography (sEMG) is an important diagnostic tool used in the evaluation of neuro-muscular diseases. Electromyography signals are generated in muscle cells and when probed at the surface of the limbs are referred to as sEMG. Existing devices have proven the utility of correlating sEMG signals with finger movements in the upper limb. Multiple limb movements are required to attain a desired action and characterizing the same through sEMG signals requires large and variant datasets, which is considered highly exhaustive process. Hence an sEMG derived feature generating model which is in close agreement with the experimental results is needed to populate datasets and evolve a better classifier model, and subsequently characterize various limb actions. The paper proposes a linear bio-electrical model to artificially synthesize RMS feature of sEMG signals for individual four finger flexion actions, which is never attempted in the past. A spatial optimization algorithm was applied iteratively to achieve the best match between the artificially synthesized RMS feature to the experimentally acquired and normalized RMS peaks extracted around the forearm for four individual finger flexion actions. The grid model incorporates different resistance profiles representing muscle fibres, tissues, bones, and skin to arrive at the four localized muscle cells group potential (MGP) points mapping four finger movements. The model with additional gaussian distribution of the noise and error at the MGP points and at output positions respectively was simulated successfully to synthesize realistic RMS features for the positioned electrodes around the model. The machine learning (ML) classifier model developed from the synthesized RMS features showed similar accuracy as that of experimental acquired data for four finger flexion actions. The model generating artificial RMS feature for finger flexion actions is a novel and significant contribution towards circuits design, simulation, and development of wearable bionic devices.
更多查看译文
关键词
Electromyography,EMG,electrical model,bioelectrical model,resistive model,RMS,features,electo-muscular activity
AI 理解论文
溯源树
样例
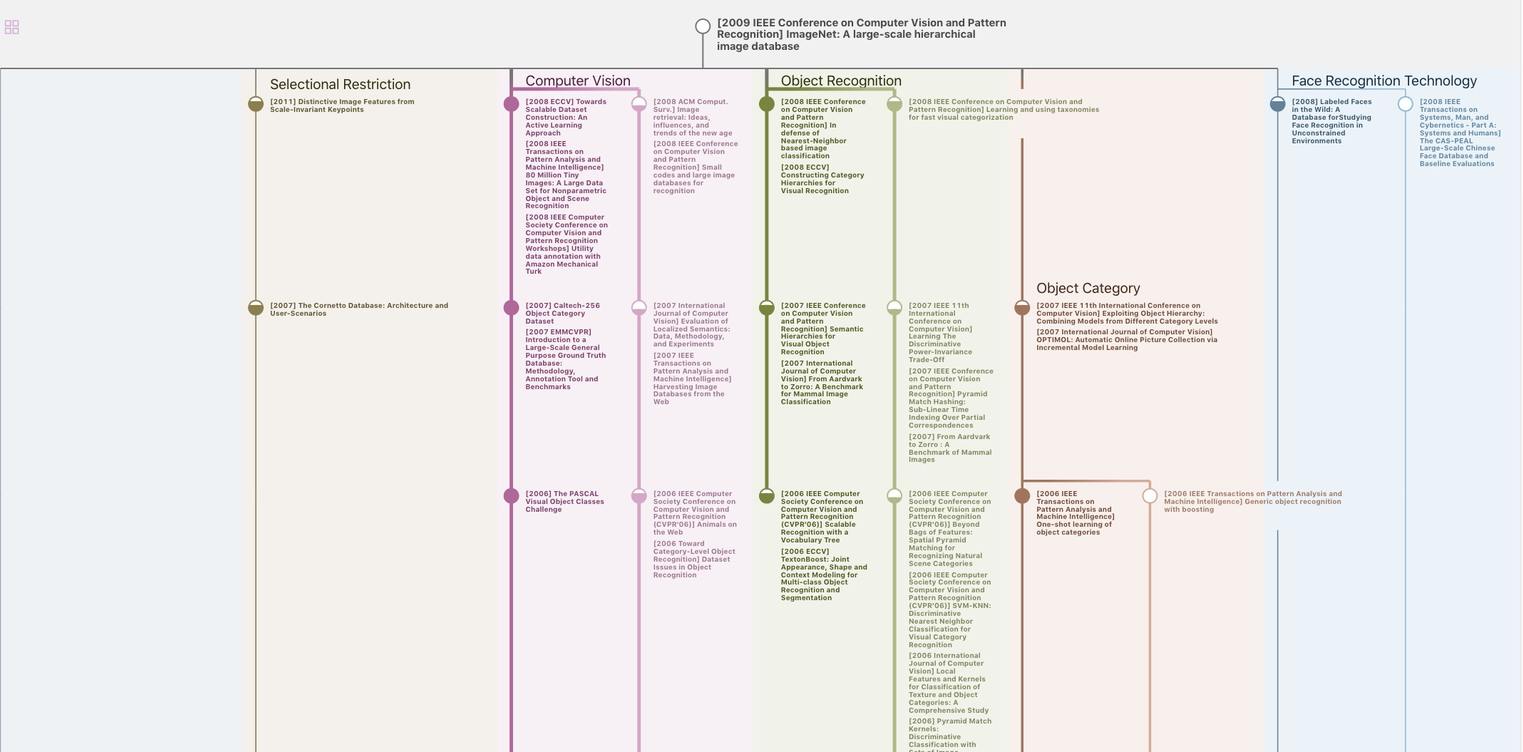
生成溯源树,研究论文发展脉络
Chat Paper
正在生成论文摘要