A Transfer Learning Image Classification Method Using Self-Supervised Information
2022 8th International Conference on Virtual Reality (ICVR)(2022)
摘要
Research on intelligent diagnosis and treatment is a major frontier issue in the current era of medical big data. For the global health crisis COVID-19, the radiological imaging techniques CT can provide useful and important information thus widely preferred due to its merit and three-dimensional view of the lung. However, to classify the CT-slices to assist in diagnosis, due to the annotation by radiologists is a highly subjective task, tedious and time-consuming work often influenced by individual bias and clinical experiences. Moreover, the current image classification methods cannot work well on the massive real-time totally unlabeled CT scans. To address these challenges, we proposed a transfer learning method using self-supervised information to classify the unlabeled CT images, using an auxiliary task of segmentation to improve classification efficiency. We classified the totally unlabeled CT scans from Huoshenshan Hospital into ordinary, severe and critical cases, and the accuracy rate reached 86%. The experimental results show that the use of small-sample semi-supervised transfer learning algorithm can be used in insufficient CT images. Our framework can improve the learning ability and achieve a higher performance. Extensive experiments on real CT volumes demonstrate that the proposed method outperforms most current models and advances the state-of-the-art performance.
更多查看译文
关键词
transfer learning,self-supervised learning,classification,semi-supervised
AI 理解论文
溯源树
样例
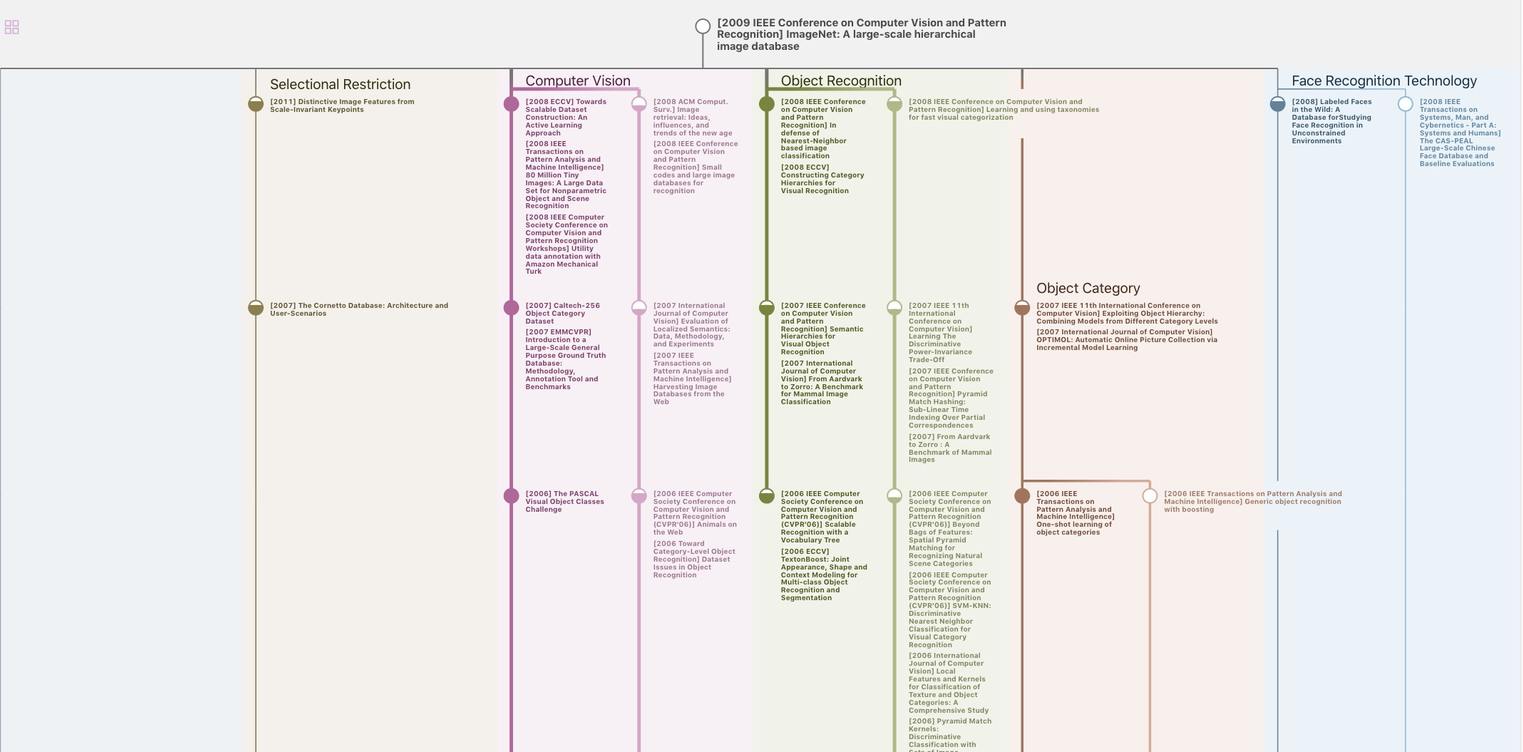
生成溯源树,研究论文发展脉络
Chat Paper
正在生成论文摘要