Ensembles of fractal descriptors with multiple deep learned features for classification of histological images
2022 29th International Conference on Systems, Signals and Image Processing (IWSSIP)(2022)
摘要
In this paper, we propose an approach to study the ensemble of handcrafted and deep learned features, as well as possible templates for associating them for the classification of histological images. The handcrafted features were calculated with fractal techniques and the deep learned features were extracted from multiple convolutional neural network architectures. The most relevant features from each ensemble, selected with a ranking algorithm, were analyzed by a heterogeneous ensemble of classifiers to avoid overfitting scenarios. The proposed method was applied in the context of histological images of breast cancer, colorectal cancer and liver tissue. The highest accuracies were values from 93.10% to 99.25%. These results allowed defining some standard templates for techniques on different kinds of histological images, for instance, the fractal descriptors when ensembled with deep features via transfer learning can provide the best results. The insights presented here are a relevant contribution to specialists interested in the field of histological images and developing techniques to support the detection and diagnostics of scientifically relevant diseases.
更多查看译文
关键词
deep features,fractal descriptors,feature ensemble,classifier ensemble,H&E images
AI 理解论文
溯源树
样例
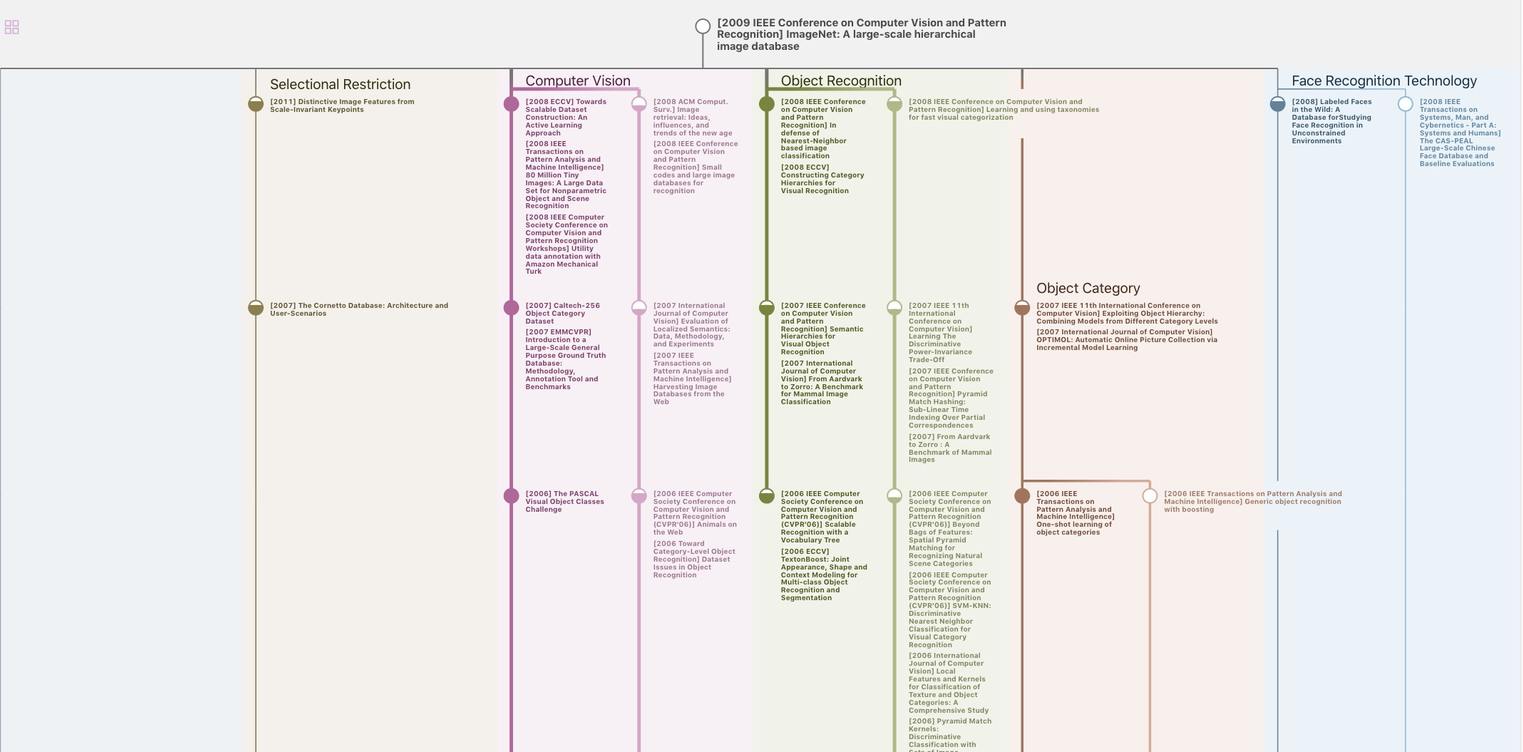
生成溯源树,研究论文发展脉络
Chat Paper
正在生成论文摘要