A Neural-Network-based Forward Model to Improve Air Quality Estimation from Spaceborne Polarimeters
2022 Systems and Information Engineering Design Symposium (SIEDS)(2022)
摘要
A growing awareness of the adverse effects of high concentrations of aerosol pollutants on human health [1] motivates the need to accurately measure and forecast the amount of PM2.5 in the air; that is the particulate matter of aerosol particles with size 2.5 microns or less in diameter [2]. Quantifying concentrations of aerosols, particularly near the surface, is foundational to the understanding of the sources, evolution, and transport of PM2.5 and will help to support environmental justice for communities across America and the world. Moreover, developing improved algorithms to accurately invert or retrieve surface-level PM2.5 from satellite remote sensing is critical to improve neighborhood-scale estimates of air quality [3]. In particular, past and future satellite polarimeter and lidar measurements will be key to understanding surface-level PM2.5 conditions in real-time across the globe. A current solution to the retrieval of accurate aerosol properties from satellite polarimeter measurements has been developed by NASA for the Plankton, Aerosols, Clouds and Ecosystems mission (PACE) mission in the form of the Micro-physical Aerosol Properties from Polarimetry (PACE-MAPP) algorithm [4]. However, because solving the vector radiative transfer is numerically intensive, and solving the non-linear inverse problem requires an iterative approach that for multiple channels involves hundreds of vector radiative transfer calls, this approach delivers products at a rate that has latencies too large for and is prohibitively inefficient for the large-scale datasets that will be needed to resolve PM2.5 at neighborhood-scale resolutions of less than 1 km by 1 km. PACE-MAPP solves this problem by developing a neural network framework to replace the complex and time-consuming vector radiative transfer calculations at each iteration. In this study, we apply the PACE-MAPP framework to polarimetry data gathered from the POLDER instrument (PO-Larization and Directionality of the Earth's Reflectances) [5] onboard PARASOL, a satellite that flew from 2006 to 2013 as a part of efforts to understand the effects of clouds and aerosols on the Earth's climate [6] [7], and demonstrate for the first time ever that a neural-network-based approach using coupled atmosphere-ocean vector radiative transfer can be applied to retrieve aerosol properties from satellite polarimeter data, and to take the first step toward evaluating the algorithm's performance at producing air quality products such as PM2.5. We further demonstrate the feasibility of deploying neural networks to solve the numerical inefficiencies that plague satellite polarimeter retrievals while maintaining high accuracy, and expect to cut the speed of acquisition by a factor of 1000.
更多查看译文
关键词
neural-network-based forward model,air quality estimation,spaceborne polarimeters,aerosol pollutants,aerosol particles,satellite remote sensing,neighborhood-scale estimates,future satellite polarimeter,lidar measurements,understanding surface-level,accurate aerosol properties,satellite polarimeter measurements,Microphysical Aerosol Properties,Polarimetry algorithm,nonlinear inverse problem,vector radiative transfer calls,neighborhood-scale resolutions,neural network framework,PACE-MAPP framework,5] onboard PARASOL,neural-network-based approach,coupled atmosphere-ocean vector radiative transfer,satellite polarimeter data,air quality products,neural networks,plague satellite polarimeter retrievals
AI 理解论文
溯源树
样例
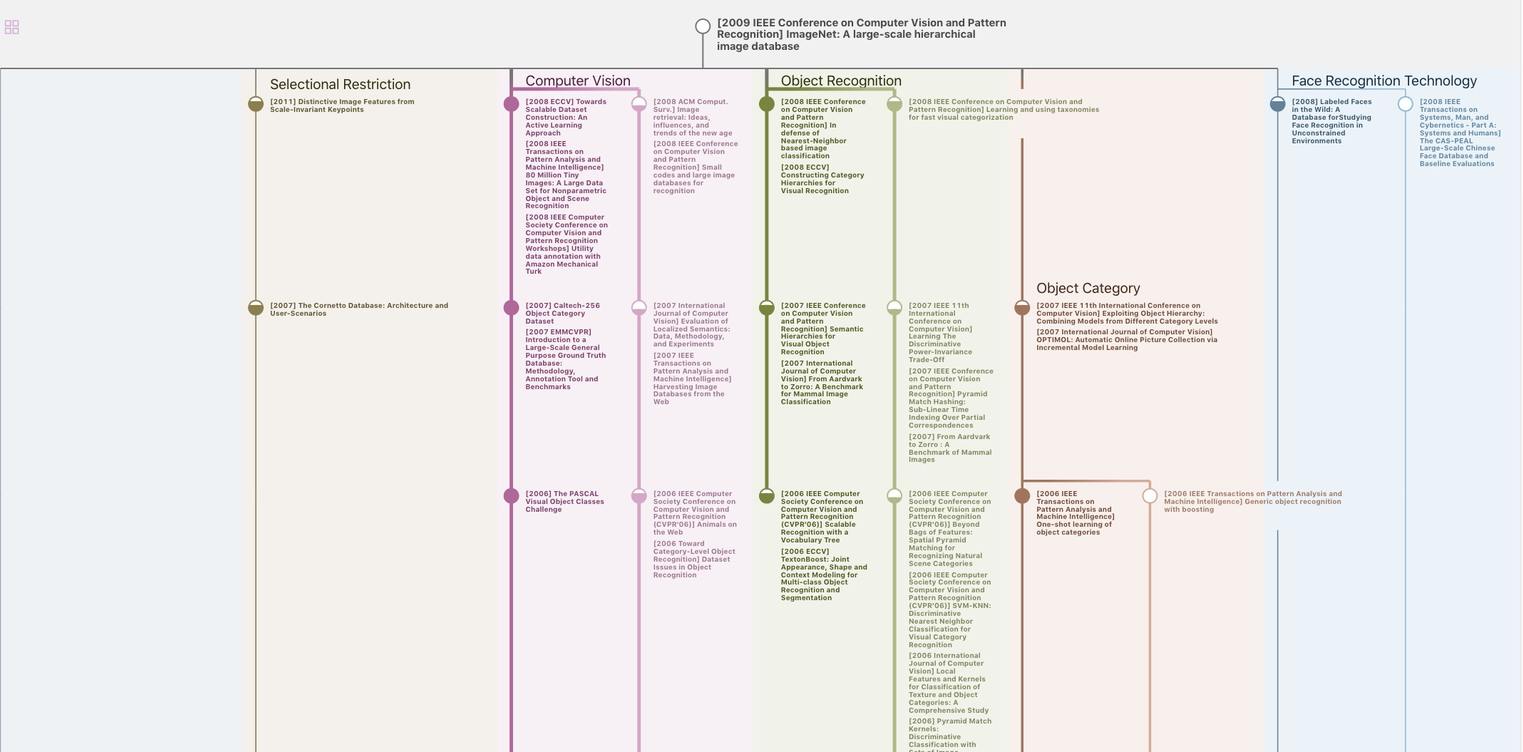
生成溯源树,研究论文发展脉络
Chat Paper
正在生成论文摘要