Predicting Episodic Video Memorability using Deep Features Fusion Strategy
International Conference on Software Engineering Research and Applications(2022)
摘要
Video memorability prediction has become an important research topic in computer vision in recent years. The movie's input is highly remembered that gains much attention with unbounded time constraints. Episodic memory is a fascinating research area that needs much attention using video processing tools and techniques. Episodic memories are long-lasting with complete detail. Movies are one of the best instances of episodic memory. This paper proposes a novel framework to fuse deep features to predict the probability of recalling episodic events. Memories are reproducible and sensitive to sophisticated set of properties rather than low-level propertiesthe proposed framework pin up the fusion of text, visual and motion features. A fuzzy-based FastText model, a supervised text extraction module, is designed to extract the annotations with their relevant classes. The colour histogram analysis is done to determine the dominant colour region that performs as a connected fragment to form episodic video sequences. A novel Faster R-CNN is designed to discover the scene objects using an informative regional proposal network formation. Here, the modified loss function sorts out the lowest overlapping regions yielding the best proposals. The 'high-level' properties are collected using Principal Component Analysis (PCA) to form episodic shots. These are fused to estimate the memorability score. The proposed framework is implemented in Mediaeval 2018 datasets. A superior spearman's rank correlation result is achieved as 0.6428 short-term and 0.4285 long-term memorability than the latest comparable methods.
更多查看译文
关键词
Video memorability prediction,episodic memory,Faster R-CNN,Fuzzy based Fast Text,Regional Proposal Network,Media Eval 2018 datasets
AI 理解论文
溯源树
样例
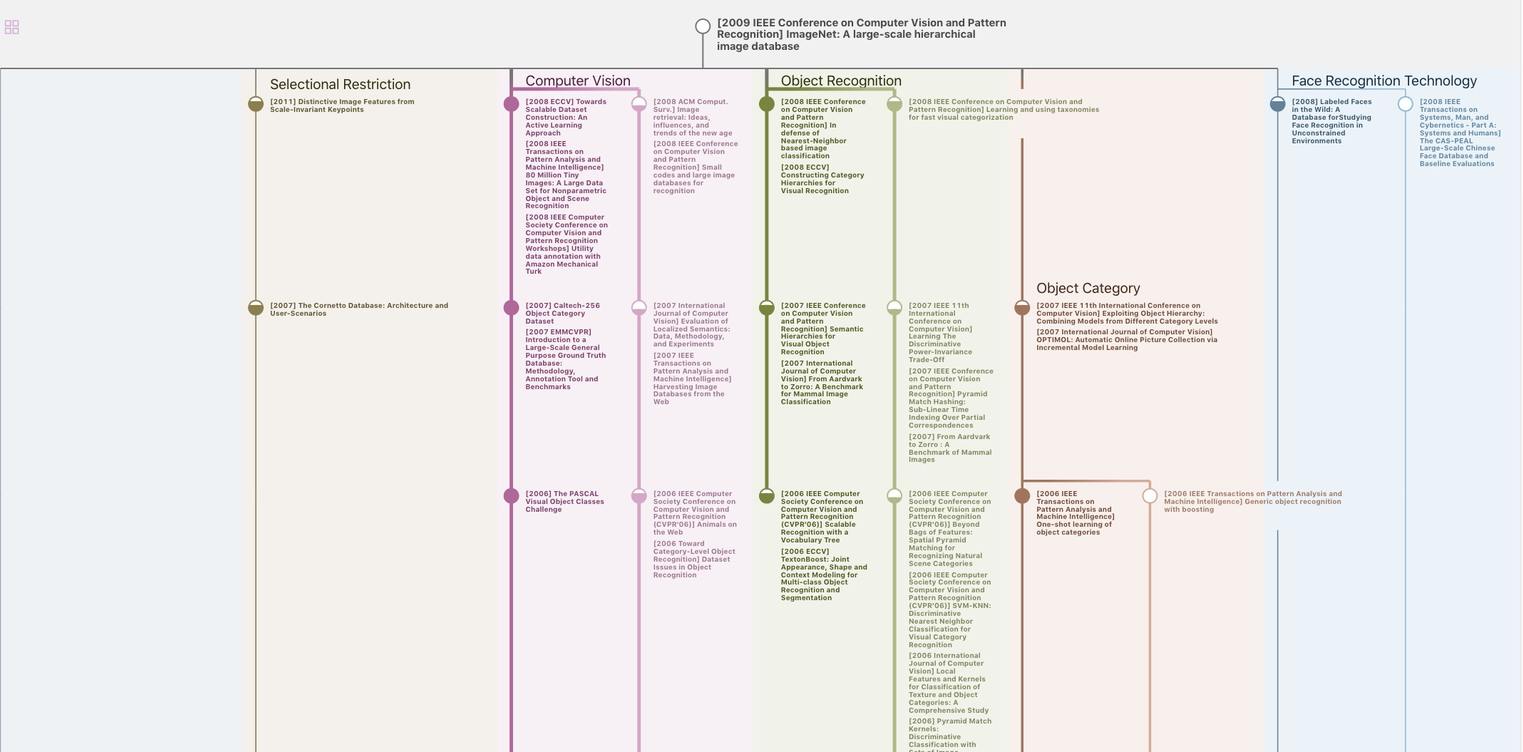
生成溯源树,研究论文发展脉络
Chat Paper
正在生成论文摘要