LOPR: Latent Occupancy PRediction using Generative Models
arxiv(2022)
摘要
Environment prediction frameworks are essential for autonomous vehicles to facilitate safe maneuvers in a dynamic environment. Previous approaches have used occupancy grid maps as a bird's eye-view representation of the scene and optimized the prediction architectures directly in pixel space. Although these methods have had some success in spatiotemporal prediction, they are, at times, hindered by unrealistic and incorrect predictions. We postulate that the quality and realism of the forecasted occupancy grids can be improved with the use of generative models. We propose a framework that decomposes occupancy grid prediction into task-independent low-dimensional representation learning and task-dependent prediction in the latent space. We demonstrate that our approach achieves state-of-the-art performance on the real-world autonomous driving dataset, NuScenes.
更多查看译文
关键词
latent occupancy prediction,generative models
AI 理解论文
溯源树
样例
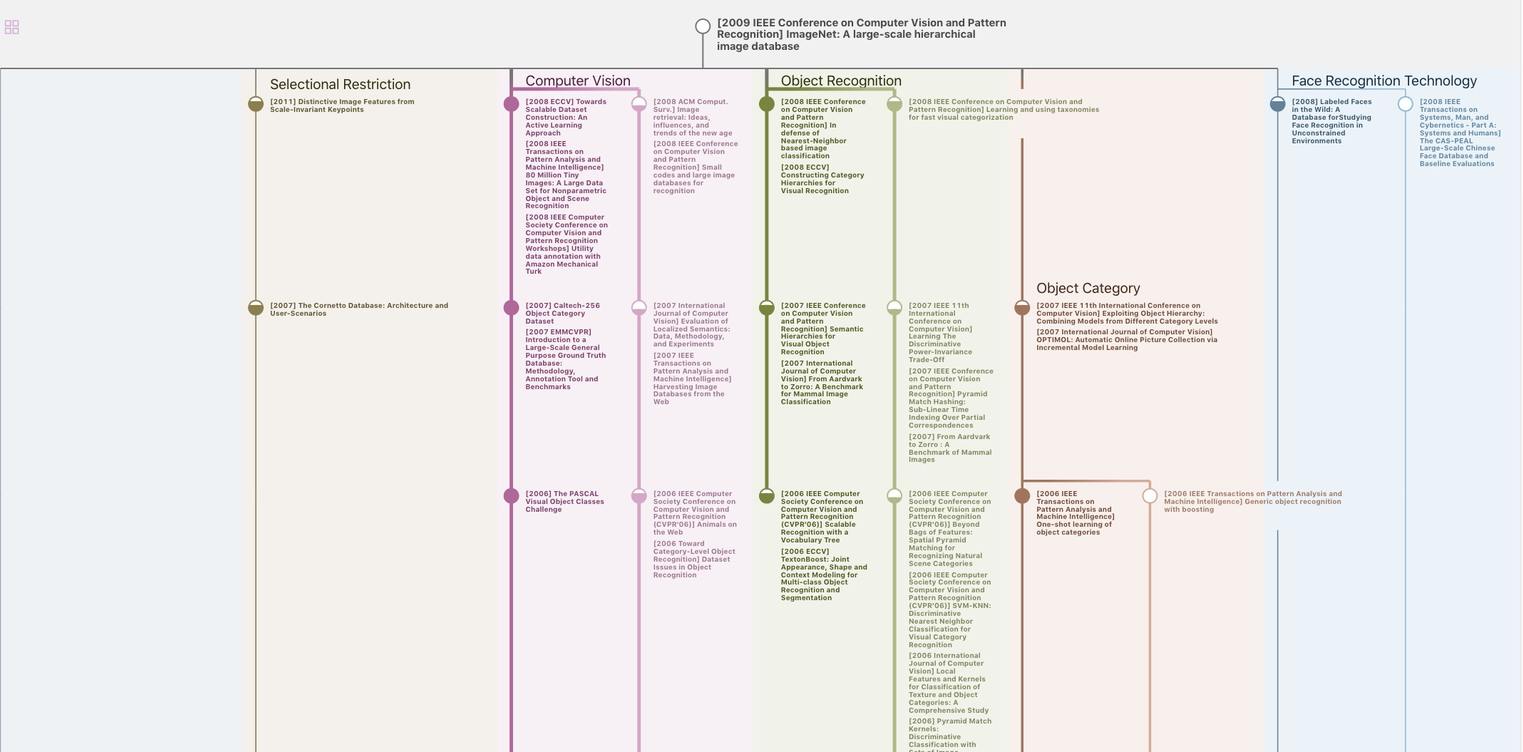
生成溯源树,研究论文发展脉络
Chat Paper
正在生成论文摘要