An Attention-Based Backend Allowing Efficient Fine-Tuning of Transformer Models for Speaker Verification
2022 IEEE Spoken Language Technology Workshop (SLT)(2023)
摘要
In recent years, self-supervised learning paradigm has received extensive attention due to its great success in various down-stream tasks. However, the fine-tuning strategies for adapting those pre-trained models to speaker verification task have yet to be fully explored. In this paper, we analyze several feature extraction approaches built on top of a pre-trained model, as well as regularization and a learning rate scheduler to stabilize the fine-tuning process and further boost performance: multi-head factorized attentive pooling is proposed to factorize the comparison of speaker representations into multiple phonetic clusters. We regularize towards the parameters of the pre-trained model and we set different learning rates for each layer of the pre-trained model during fine-tuning. The experimental results show our method can significantly shorten the training time to 4 hours and achieve SOTA performance: 0.59%, 0.79% and 1.77% EER on Vox1-O, Vox1-E and Vox1-H, respectively.
1
1
Code is available at https://github.com/JunyiPeng00/IEEE-SLT22-Pretrained-Model-for-SV.
更多查看译文
关键词
Pre-trained model,fine-tuning strategy,speaker verification,attentive pooling
AI 理解论文
溯源树
样例
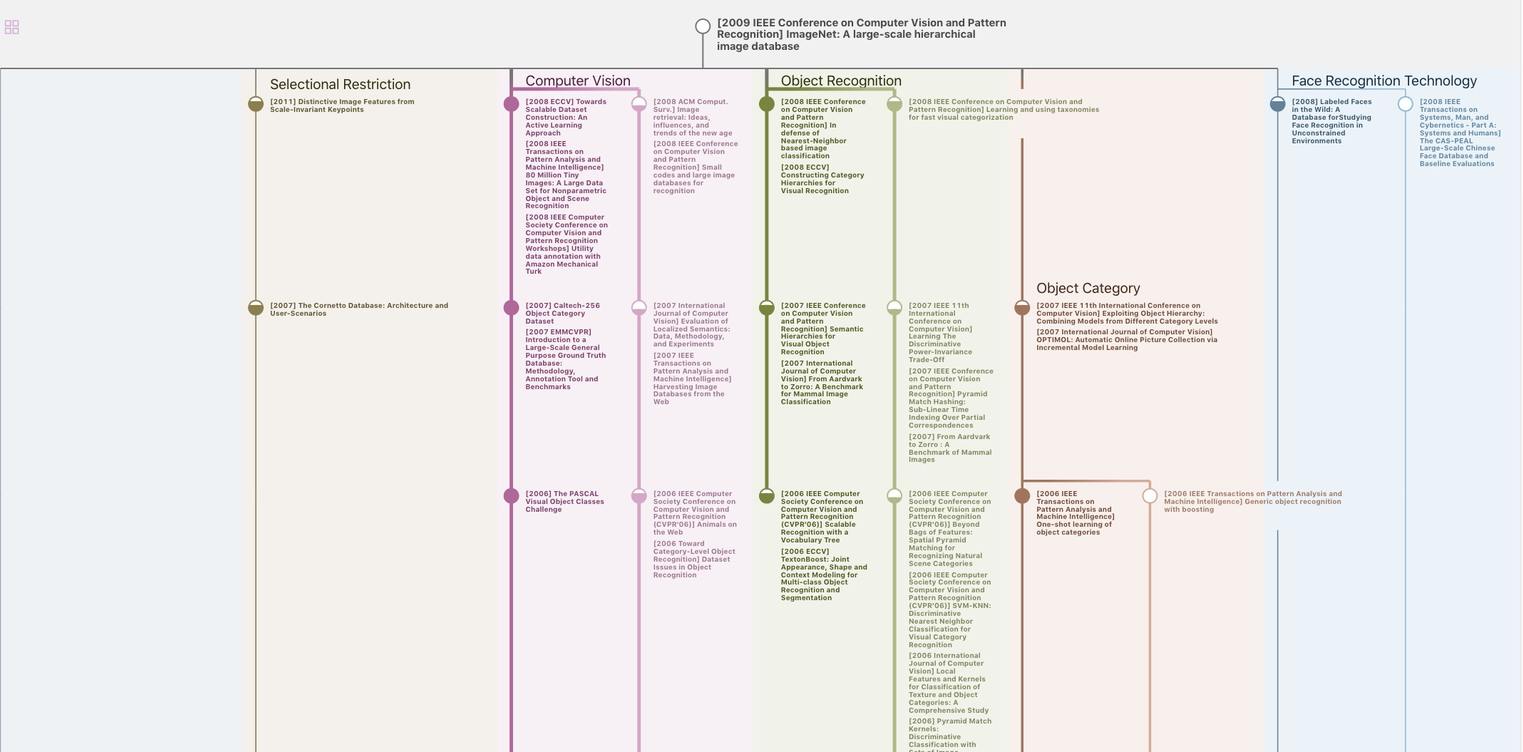
生成溯源树,研究论文发展脉络
Chat Paper
正在生成论文摘要