The Dynamics of Sharpness-Aware Minimization: Bouncing Across Ravines and Drifting Towards Wide Minima
JOURNAL OF MACHINE LEARNING RESEARCH(2023)
摘要
We consider Sharpness-Aware Minimization (SAM), a gradient-based optimization method for deep networks that has exhibited performance improvements on image and language prediction problems. We show that when SAM is applied with a convex quadratic objective, for most random initializations it converges to a cycle that oscillates between either side of the minimum in the direction with the largest curvature, and we provide bounds on the rate of convergence. In the non-quadratic case, we show that such oscillations effectively perform gradient descent, with a smaller step-size, on the spectral norm of the Hessian. In such cases, SAM's update may be regarded as a third derivative-the derivative of the Hessian in the leading eigenvector direction-that encourages drift toward wider minima.
更多查看译文
关键词
Non-convex optimization,wide minima,sharpness-aware minimization.
AI 理解论文
溯源树
样例
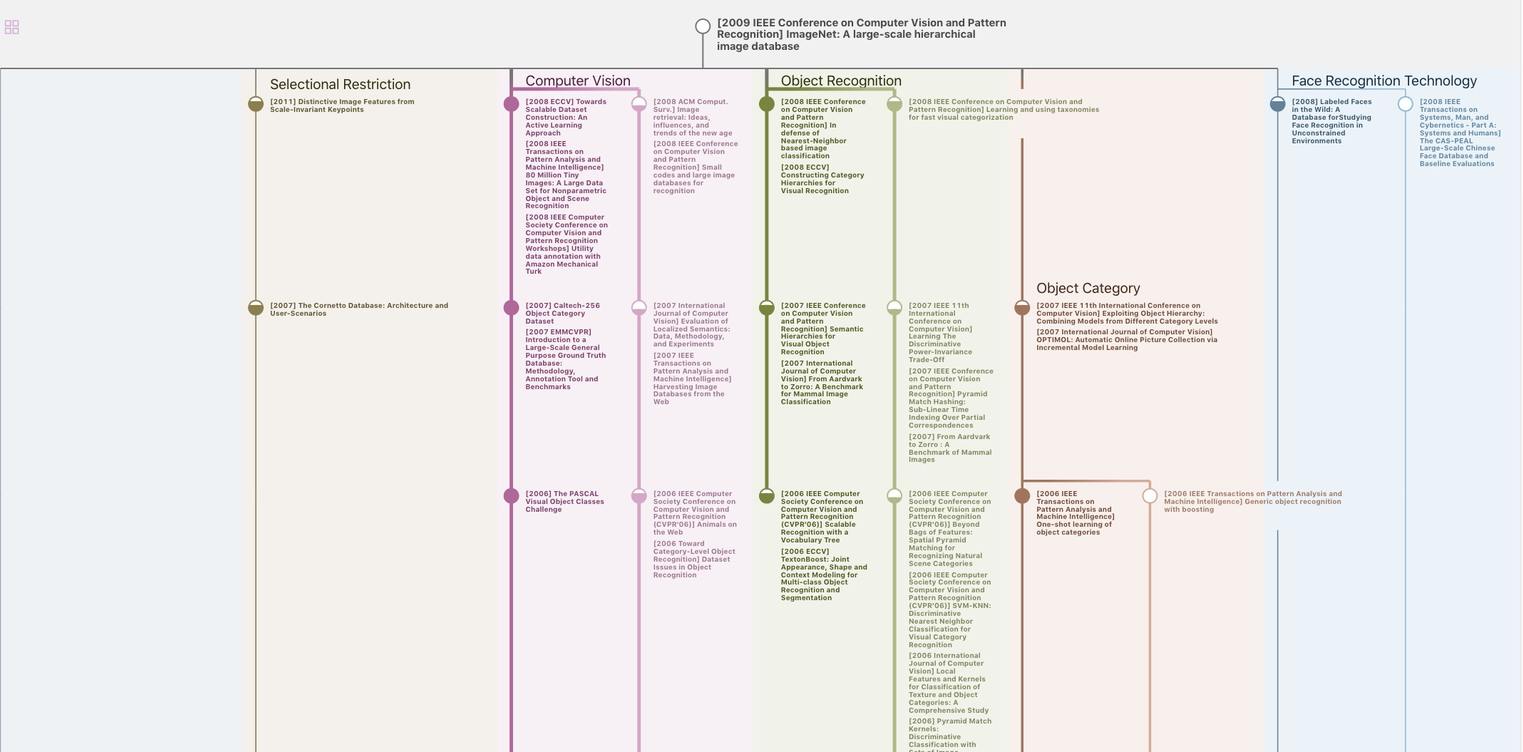
生成溯源树,研究论文发展脉络
Chat Paper
正在生成论文摘要