Improving Quantum Classifier Performance in NISQ Computers by Voting Strategy from Ensemble Learning
arxiv(2022)
摘要
Due to the immense potential of quantum computers and the significant computing overhead required in machine learning applications, the variational quantum classifier (VQC) has received a lot of interest recently for image classification. The performance of VQC is jeopardized by the noise in Noisy Intermediate-Scale Quantum (NISQ) computers, which is a significant hurdle. It is crucial to remember that large error rates occur in quantum algorithms due to quantum decoherence and imprecision of quantum gates. Previous studies have looked towards using ensemble learning in conventional computing to reduce quantum noise. We also point out that the simple average aggregation in classical ensemble learning may not work well for NISQ computers due to the unbalanced confidence distribution in VQC. Therefore, in this study, we suggest that ensemble quantum classifiers be optimized with plurality voting. On the MNIST dataset and IBM quantum computers, experiments are carried out. The results show that the suggested method can outperform state-of-the-art on two- and four-class classifications by up to 16.0% and 6.1% , respectively.
更多查看译文
关键词
quantum classifier performance,nisq computers,ensemble learning,voting
AI 理解论文
溯源树
样例
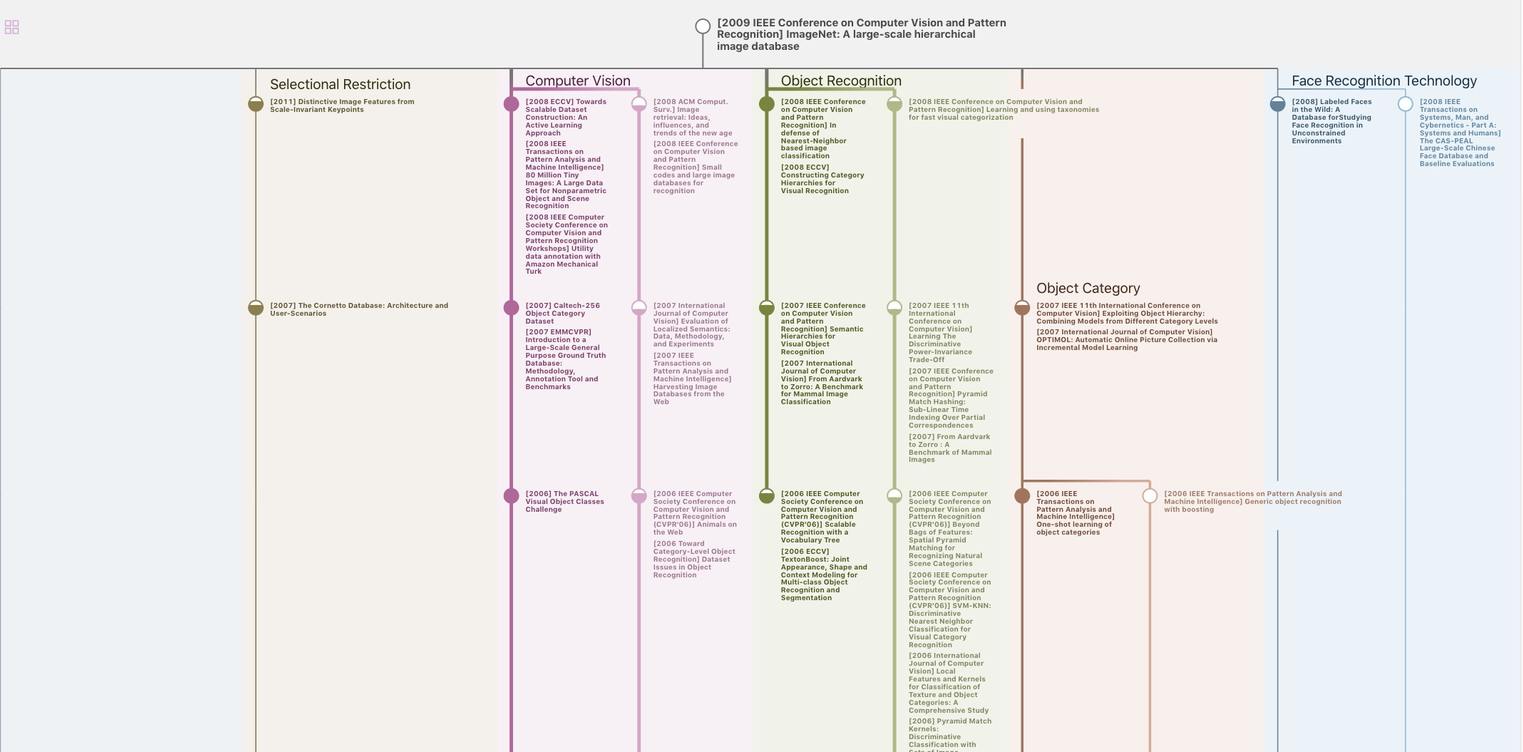
生成溯源树,研究论文发展脉络
Chat Paper
正在生成论文摘要