New Machine Learning Techniques for Simulation-Based Inference: InferoStatic Nets, Kernel Score Estimation, and Kernel Likelihood Ratio Estimation
arxiv(2023)
摘要
We propose an intuitive, machine-learning approach to multiparameter inference, dubbed the InferoStatic Networks (ISN) method, to model the score and likelihood ratio estimators in cases when the probability density can be sampled but not computed directly. The ISN uses a backend neural network that models a scalar function called the inferostatic potential $\varphi$. In addition, we introduce new strategies, respectively called Kernel Score Estimation (KSE) and Kernel Likelihood Ratio Estimation (KLRE), to learn the score and the likelihood ratio functions from simulated data. We illustrate the new techniques with some toy examples and compare to existing approaches in the literature. We mention en passant some new loss functions that optimally incorporate latent information from simulations into the training procedure.
更多查看译文
关键词
kernel score estimation,inferostatic nets,inference,learning,simulation-based
AI 理解论文
溯源树
样例
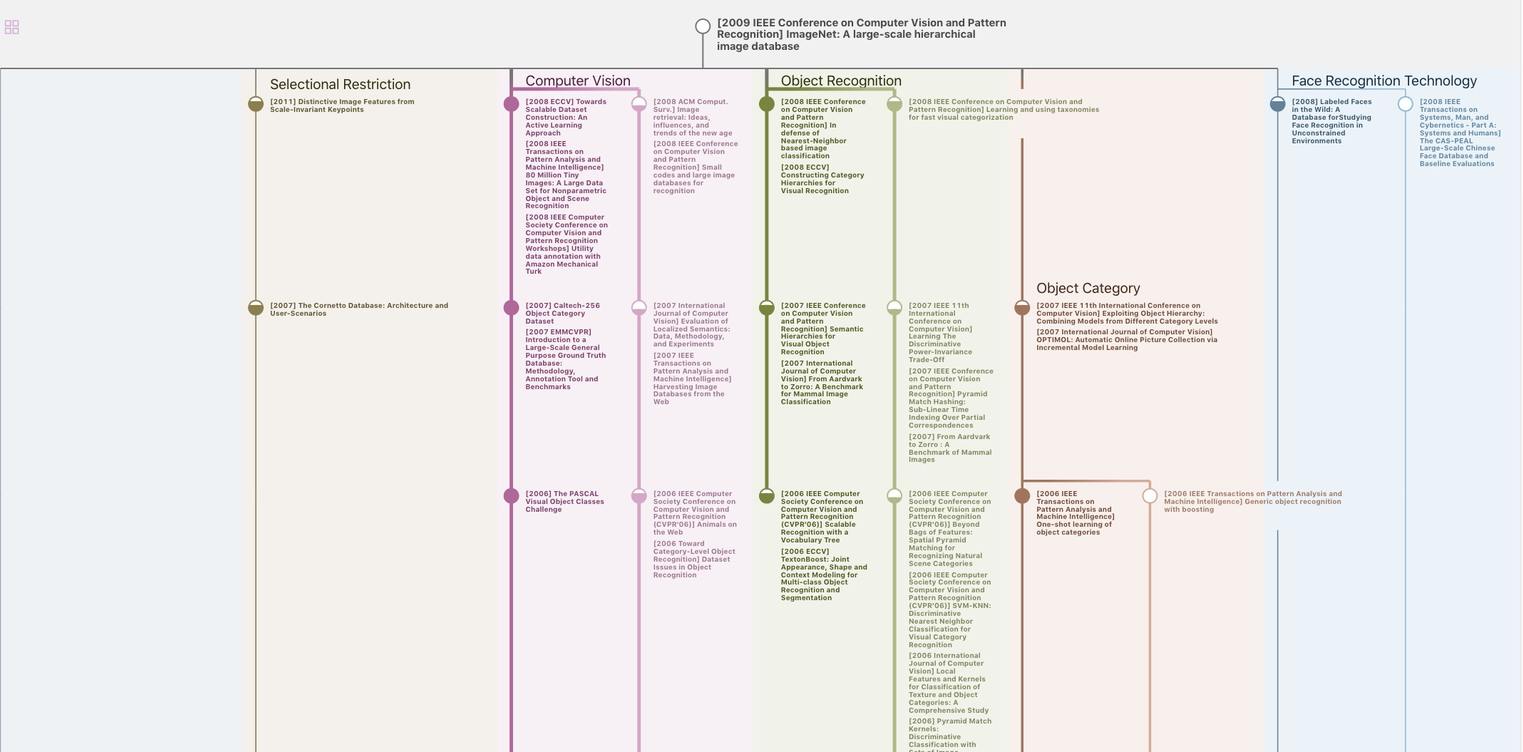
生成溯源树,研究论文发展脉络
Chat Paper
正在生成论文摘要