Knowledge Distillation based Contextual Relevance Matching for E-commerce Product Search
arxiv(2022)
摘要
Online relevance matching is an essential task of e-commerce product search to boost the utility of search engines and ensure a smooth user experience. Previous work adopts either classical relevance matching models or Transformer-style models to address it. However, they ignore the inherent bipartite graph structures that are ubiquitous in e-commerce product search logs and are too inefficient to deploy online. In this paper, we design an efficient knowledge distillation framework for e-commerce relevance matching to integrate the respective advantages of Transformer-style models and classical relevance matching models. Especially for the core student model of the framework, we propose a novel method using $k$-order relevance modeling. The experimental results on large-scale real-world data (the size is 6$\sim$174 million) show that the proposed method significantly improves the prediction accuracy in terms of human relevance judgment. We deploy our method to the anonymous online search platform. The A/B testing results show that our method significantly improves 5.7% of UV-value under price sort mode.
更多查看译文
关键词
contextual relevance matching,distillation,search,knowledge,e-commerce
AI 理解论文
溯源树
样例
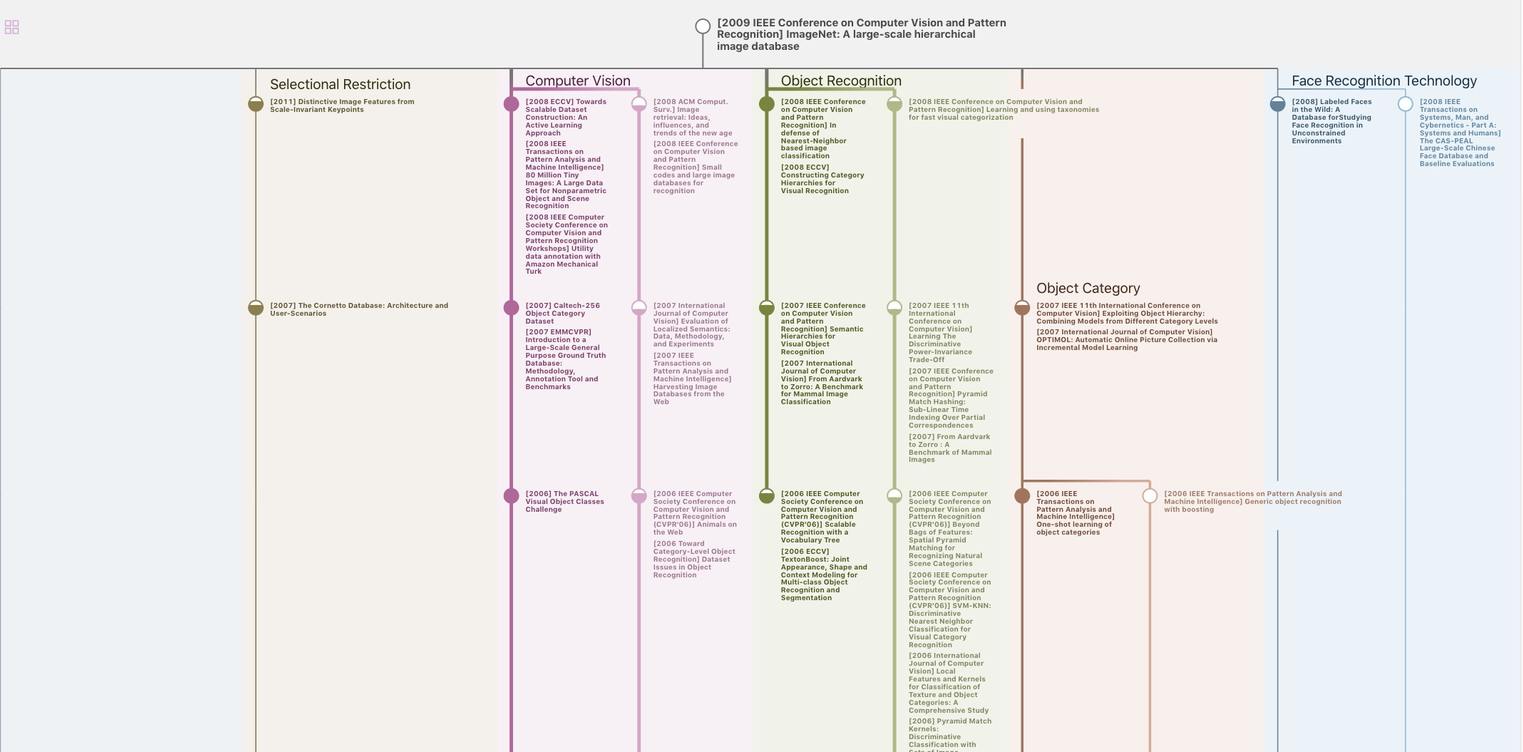
生成溯源树,研究论文发展脉络
Chat Paper
正在生成论文摘要