Transfer Learning for Event-Type Differentiation on Power Systems
2022 International Conference on Smart Grid Synchronized Measurements and Analytics (SGSMA)(2022)
摘要
Machine Learning (ML) models are continuously introduced to power systems in domains like state estimation and event identification. However, training an ML model usually requires a lot of data. For data-limited grids, we propose a transfer learning framework to transfer knowledge from a source grid with rich Phasor Measurement Unit (PMU) data for the event-type differentiation problem. The goal is challenging due to (1) different dimensionalities of the source and the target measurement spaces, (2) dissimilar data distributions, and (3) redundant PMU’s information. Thus, we project the source and the target measurement space into a latent feature space, which reduces and aligns the dimensionality of input measurements, maintains close data distributions in the latent space, and enables the transferability from the source domain to the target domain. Then, we introduce transfer learning in supervised learning by vectorizing each PMU’s measurement window as one training sample, forming the latent space. We theoretically show that our approach minimizes the upper bound of misclassification rate and experimentally demonstrates the high performance on various synthetic datasets.
更多查看译文
关键词
Event-type differentiation,power systems,transfer learning,latent feature space,dimensionality reduction
AI 理解论文
溯源树
样例
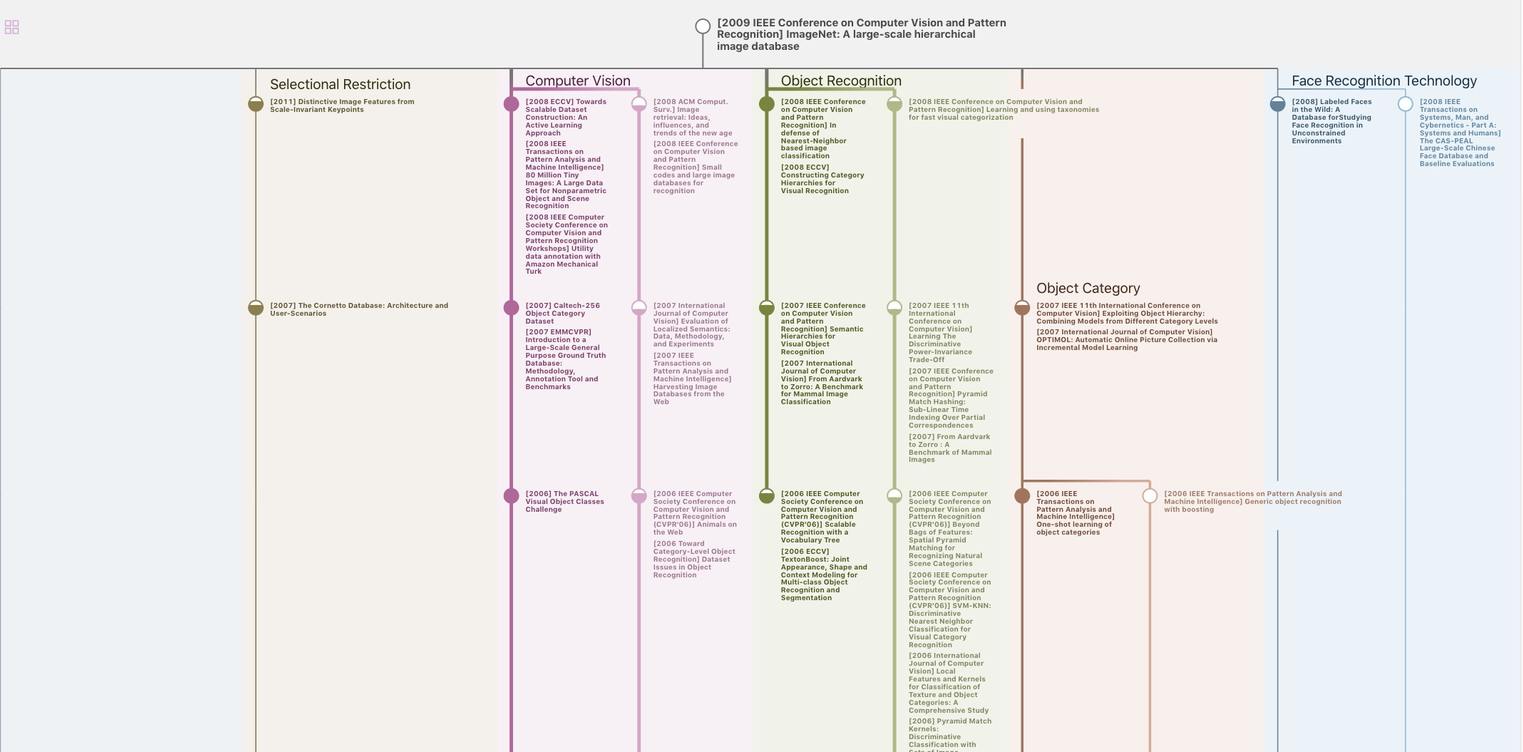
生成溯源树,研究论文发展脉络
Chat Paper
正在生成论文摘要