Automatic Dense Tissue Segmentation in Digital Mammography Images Based on Fully Convolutional Network and Intensity-Based Clustering
2022 IEEE COLOMBIAN CONFERENCE ON APPLICATIONS OF COMPUTATIONAL INTELLIGENCE (COLCACI 2022)(2022)
摘要
Mammographic breast percent density (PD) is one of the strongest risk factors associated with the development of breast cancer. As a result, the accurate estimation of PD from screening mammograms is an important problem for breast cancer risk assessment. Nevertheless, automatic segmentation of the dense fibroglandular tissue (FGT) is a difficult task due to the complexity of morphological characteristics and heterogeneity of the breast. In this work, we present a hybrid algorithm based on convolutional neural networks (CNN) and intensity-based clustering used for the fully-automated segmentation of dense tissue in mammograms. We utilize a dataset of 582 mammograms with expert reader’s manually segmented dense tissue areas as a reference. The PD estimates obtained with the proposed method yield a median PD error of 7.7% with no statistically significant differences with respect to the expert. The proposed method is also compared to a clinically validated algorithm
更多查看译文
关键词
percent density,fibroglandular tissue,digital mammography,convolutional neural network,breast cancer,risk
AI 理解论文
溯源树
样例
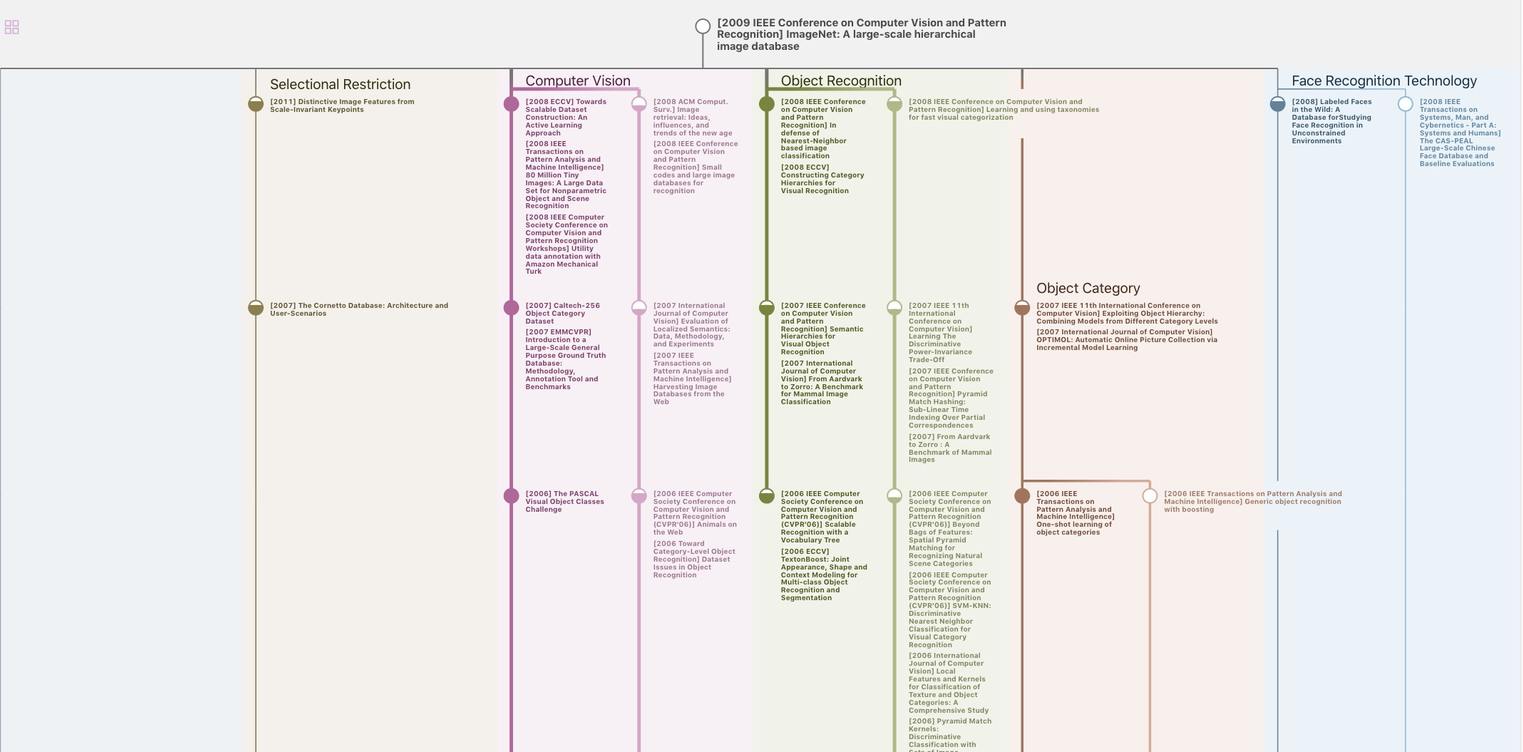
生成溯源树,研究论文发展脉络
Chat Paper
正在生成论文摘要