Smart Edge-Enabled Traffic Light Control: Improving Reward-Communication Trade-offs with Federated Reinforcement Learning
2022 IEEE International Conference on Smart Computing (SMARTCOMP)(2022)
摘要
Traffic congestion is a costly phenomenon of every-day life. Reinforcement Learning (RL) is a promising solution due to its applicability to solving complex decision-making problems in highly dynamic environments. To train smart traffic lights using RL, large amounts of data is required. Recent RL-based approaches consider training to occur on some nearby server or a remote cloud server. However, this requires that traffic lights all communicate their raw data to some central location. For large road systems, communication cost can be impractical, particularly if traffic lights collect heavy data (e.g., video, LIDAR). As such, this work pushes training to the traffic lights directly to reduce communication cost. However, completely independent learning can reduce the performance of trained models. As such, this work considers the recent advent of Federated Reinforcement Learning (FedRL) for edge-enabled traffic lights so they can learn from each other's experience by periodically aggregating locally-learned policy network parameters rather than share raw data, hence keeping communication costs low. To do this, we propose the SEAL framework which uses an intersection-agnostic representation to support FedRL across traffic lights controlling heterogeneous intersection types. We then evaluate our FedRL approach against Centralized and Decentralized RL strategies. We compare the reward-communication trade-offs of these strategies. Our results show that FedRL is able to reduce the communication costs associated with Centralized training by 36.24%; while only seeing a 2.11 % decrease in average reward (i.e., decreased traffic congestion).
更多查看译文
关键词
Smart Traffic,Traffic Light Control,Reinforcement Learning,Edge Computing,Federated Learning
AI 理解论文
溯源树
样例
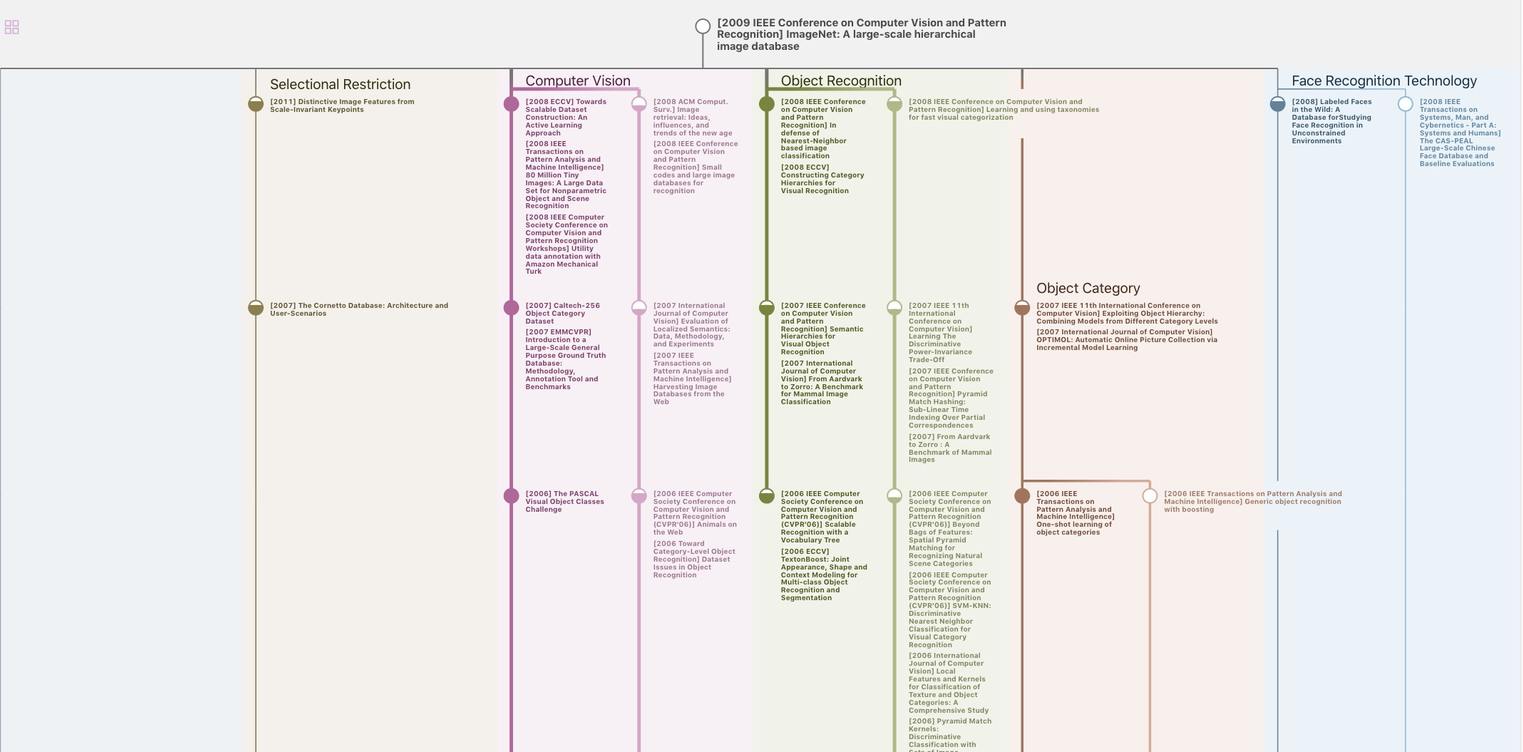
生成溯源树,研究论文发展脉络
Chat Paper
正在生成论文摘要