Reuse of Client Models in Federated Learning
2022 IEEE INTERNATIONAL CONFERENCE ON SMART COMPUTING (SMARTCOMP 2022)(2022)
摘要
Federated Learning (FL) has attracted a lot of attention from both academia and industry. In FL, user data no more need to be transmitted to the data center and each client device trains the deep model using its personal data so as to protect user privacy from being revealed. There exists two kinds of FL architecture, cloud based and edge based. Researchers have proposed the client-edge-cloud hierarchical FL system combining their advantage together to take the full advantage and avoid the defects. To improve the utilization ratio of local model parameters and data, so that enhance every single edge model accuracy, and ultimately achieve a more outstanding global model, we propose an algorithm Client Model Multiple Access (CMMA). CMMA allows clients associate with a set of edge servers, and uploads its training results to all servers in the set. That said, the local model of one client is reused by multiple edge servers. Such reuse can improve model performance particularly when the number of clients is small. Empirical experiments demonstrate the superiority of our scheme in different datasets, CNN models and data distributions. The results have not just shown the general suppress CMMA against hierarchical FL, but also validate the advantage of CMMA guaranteeing global model accuracy in unstable network or short of clients dilemma.
更多查看译文
关键词
Federated Learning,Mobile Edge Computing,Edge Learning,Deep Learning,Distributed Learning
AI 理解论文
溯源树
样例
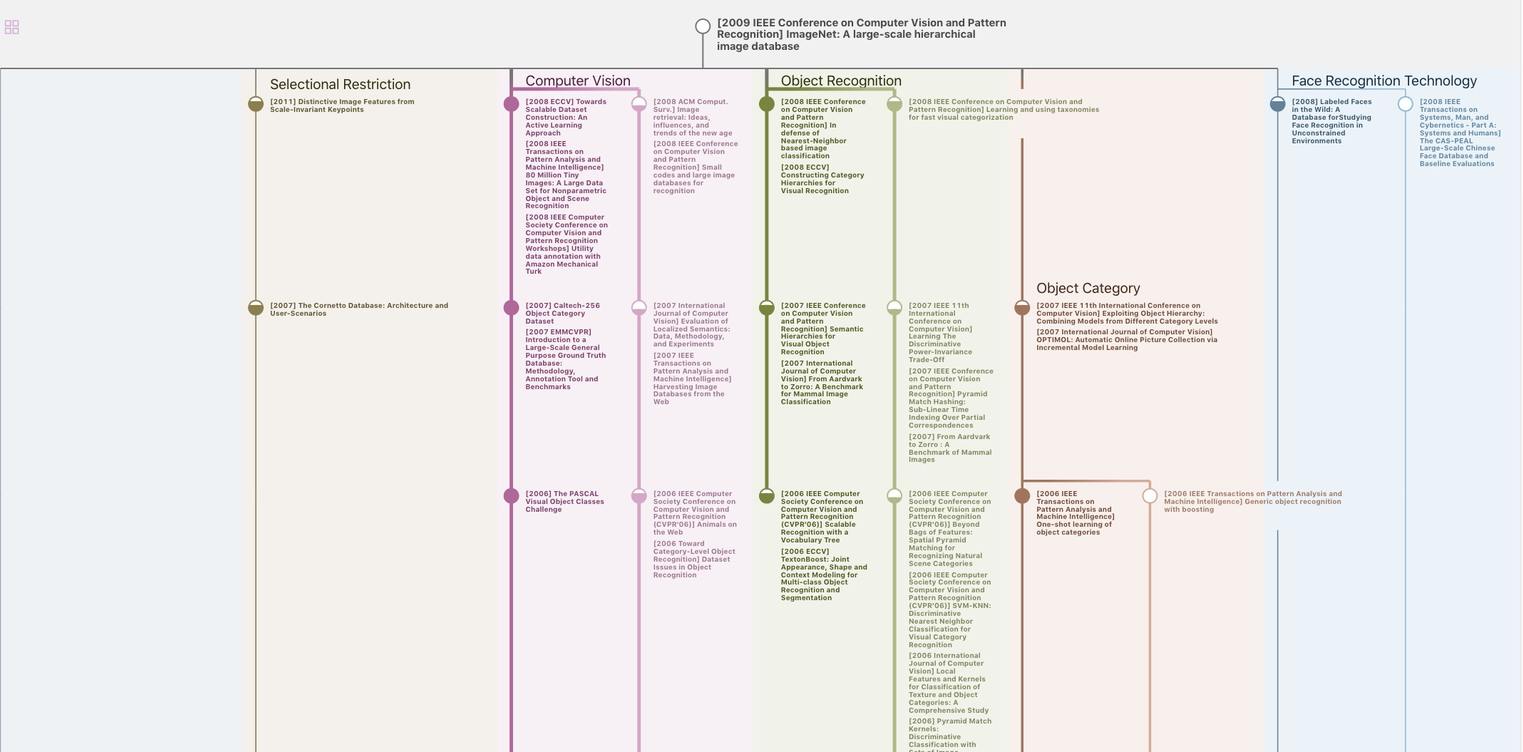
生成溯源树,研究论文发展脉络
Chat Paper
正在生成论文摘要