Learning Student Intents and Named Entities in the Education Domain
International Journal on Artificial Intelligence Tools(2022)
摘要
Detecting intents and extracting necessary contextual information (aka named entities) in input utterances are two fundamental tasks in understanding what the users say in chatbot systems. While most work in this field has been dedicated to high-resource languages in popular domains like business and home automation, little research has been done for low-resource languages, especially in a less popular domain like education. To narrow this gap, this paper presents the first study on learning to detect student intents and to recognize named entities in the education domain targeted to the Vietnamese language. Specifically, we first introduce a complete corpus consisting of 3690 utterances of students. It was manually annotated with both named entities and intent information at two levels of granularity: the fine-grained and the coarse-grained levels. We then systematically investigate different approaches to deal with the two tasks using not only independent but also joint learning architectures. The experimental results show that the joint architectures based on pre-trained language models are superior in boosting the performance of both tasks. They outperformed the conventional independent learning architectures which looked at the two tasks separately. Moreover, to further enhance the final performance, this paper proposes a technique to enrich the models with more useful linguistic features. Compared to the standard approaches, we achieve considerably better results for two tasks in both architectures. Overall, for the named entity recognition task, the best model yielded an F-1 score of 88.61%. For the intent detection task, it yielded F-1 scores of 94.36% and 91.62% at the coarse-grained and fine-grained levels, respectively.
更多查看译文
关键词
Student intents,named entities,education domain,chatbots
AI 理解论文
溯源树
样例
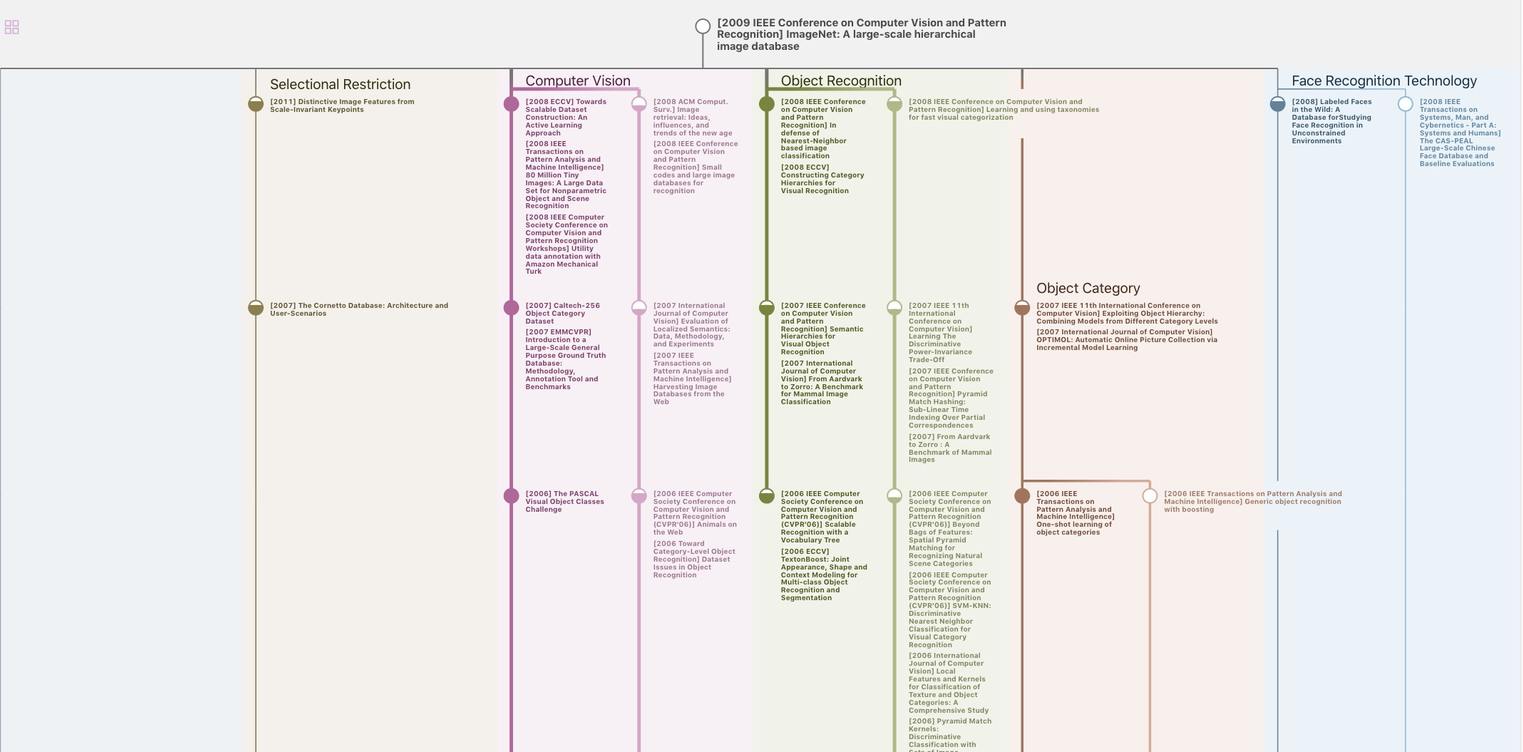
生成溯源树,研究论文发展脉络
Chat Paper
正在生成论文摘要