Kernelized dynamic convolution routing in spatial and channel interaction for attentive concrete defect recognition
SIGNAL PROCESSING-IMAGE COMMUNICATION(2022)
摘要
Image/video based defect recognition is a crucial task in automating visual inspection of concrete structures. Although some progress has been made to automatically recognize defects in concrete structural images, significant challenges still exist. In this work, we propose a deep convolutional neural network architecture that embeds novel spatial-channel interaction based concurrent attention (SCA) for multi-target, multi-class defect recognition. SCA module stems from novel kernel salient feature (KSF) encoder that captures higher-order features with robust discriminative representation of concrete defects. KSF encoder incorporates kernelized convolution followed by dynamic routing operation to be used as the primary building block. The proposed CSDNet architecture is able to apportion higher weightage in defective regions while suppressing large background area (healthy region), thereby improving recognition performance of overlapping defects in concrete structures. Experimental results and ablation study on four large benchmark datasets show consistent superiority of our proposed network as compared to current state-of-the-art methodologies. This end-to-end trainable architecture can be augmented with unmanned aerial vehicles (UAVs) to monitor health of massive infrastructures to leverage high concrete defect recognition performance.
更多查看译文
关键词
Kernel salient feature encoder,Spatial-channel attention,Concrete structural defect,Convolutional neural network,Multi-target multi-class classification
AI 理解论文
溯源树
样例
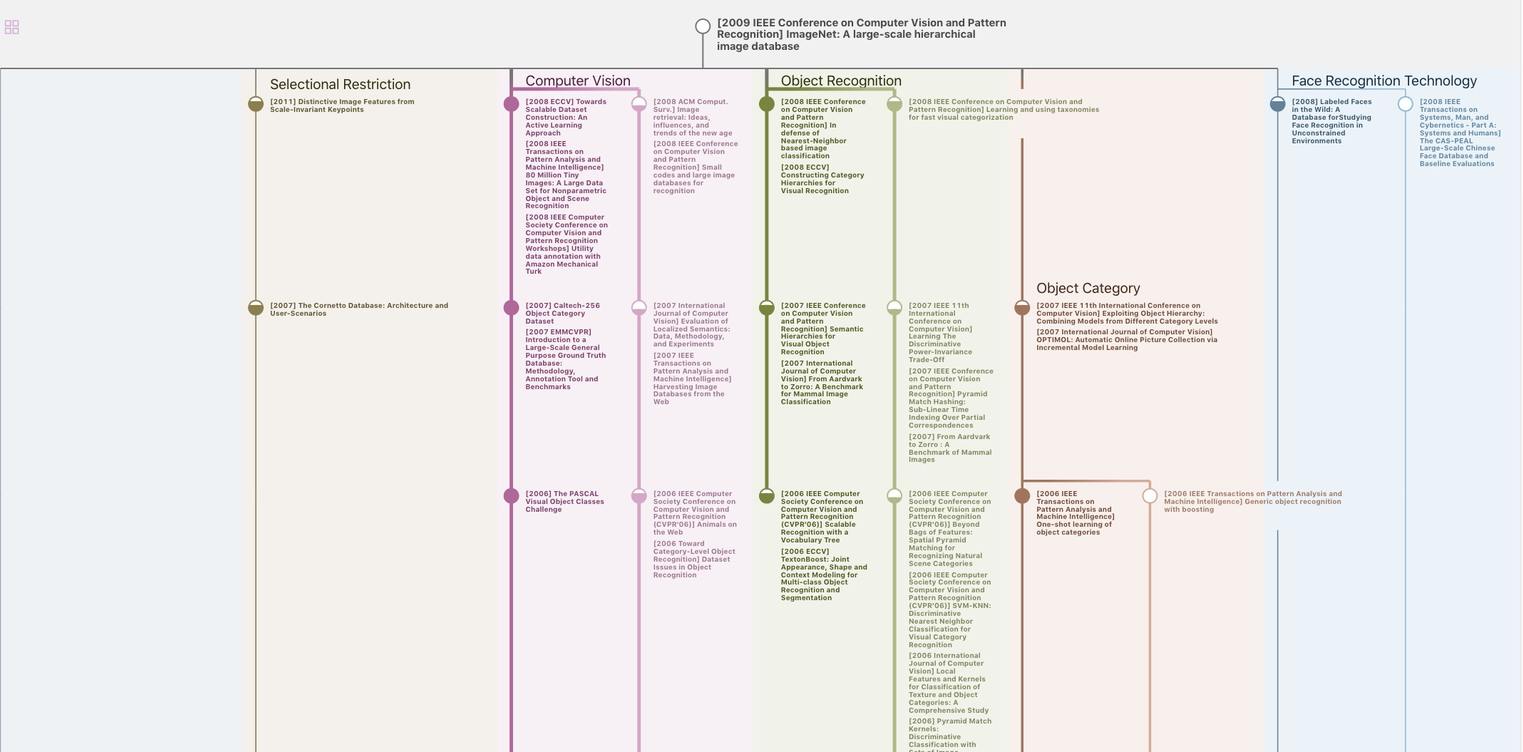
生成溯源树,研究论文发展脉络
Chat Paper
正在生成论文摘要