Quantification of the Loading Coaxiality for the Uniaxial Material Test System with a Cylindrical Specimen via Optimized Neural Network
Journal of Physics: Conference Series(2022)
摘要
Abstract Material testing systems are crucial for evaluating the mechanical characteristics of materials. The material testing system’s accuracy determines the mechanical property of the material. Loading coaxiality is a crucial factor determining the precision of uniaxial tensile testing machines. Because of solid boundary nonlinearity in calculating loading coaxiality of the uniaxial test system, current methods can only be used for qualitative evaluation rather than quantification. Using the finite element method and the deep learning technique, a mapping relationship has been established between the strain measurements on the rod specimen and the alignment deviations of the material uniaxial testing system to quantify the loading coaxiality of the material testing system. The regularized genetic algorithm was further used to optimize the deep learning model’s hyperparameters, including learning rate, batch size, and network structure. Results showed that the deep learning model developed in this study could be used to quantify the alignment deviation of the uniaxial system based on strain distribution on the specimen. Besides providing a source for error analysis for material testing research, quantitative computation of alignment deviation can also guide the design, manufacture, and assembly of material uniaxial test systems.
更多查看译文
AI 理解论文
溯源树
样例
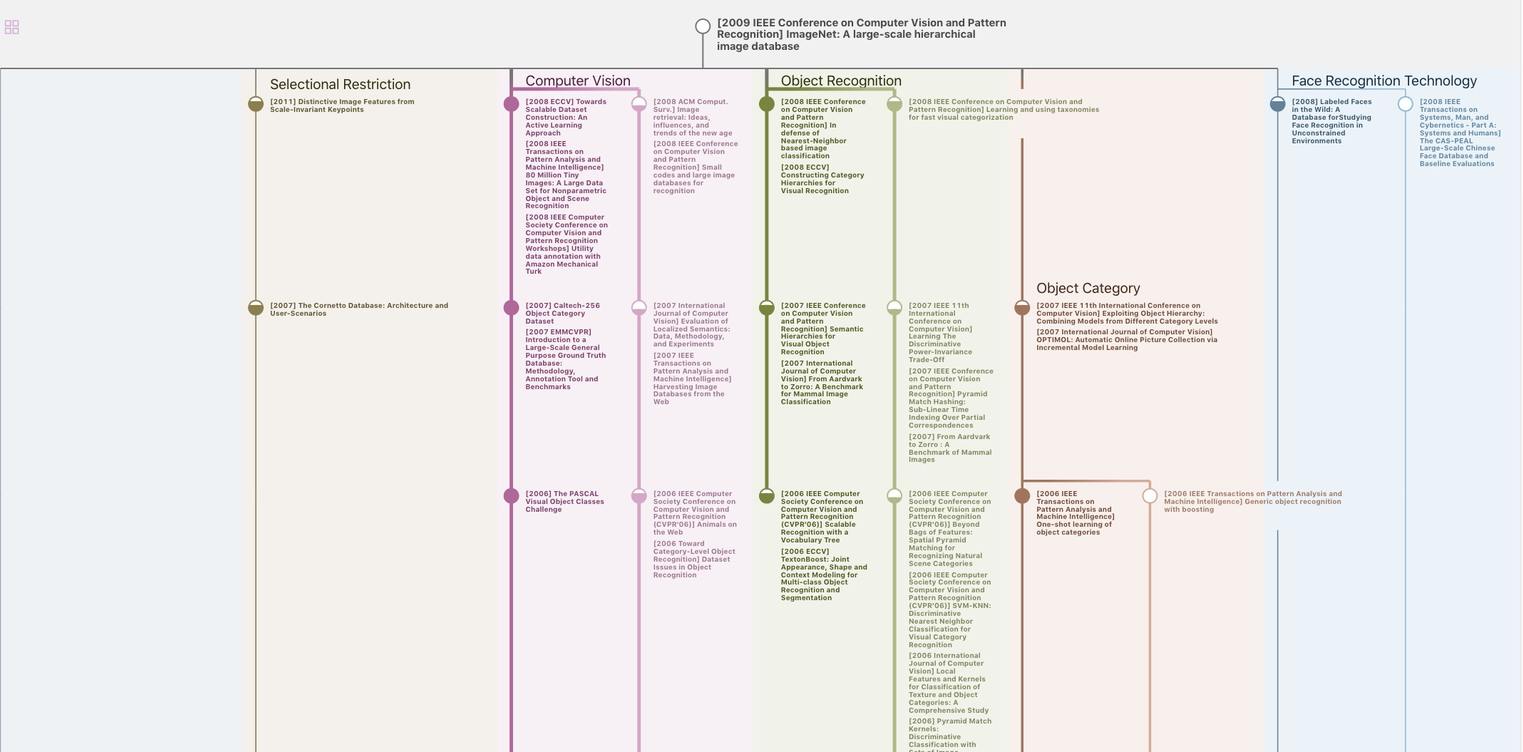
生成溯源树,研究论文发展脉络
Chat Paper
正在生成论文摘要