Nonparametric and Regularized Dynamical Wasserstein Barycenters for Sequential Observations
IEEE TRANSACTIONS ON SIGNAL PROCESSING(2023)
Abstract
We consider probabilistic models for sequential observations which exhibit gradual transitions among a finite number of states. We are particularly motivated by applications such as human activity analysis where observed accelerometer time series contains segments representing distinct activities, which we call pure states, as well as periods characterized by continuous transition among these pure states. To capture this transitory behavior, the dynamical Wasserstein barycenter (DWB) model of (Cheng et al., 2021) associates with each pure state a data-generating distribution and models the continuous transitions among these states as a Wasserstein barycenter of these distributions with dynamically evolving weights. Focusing on the univariate case where Wasserstein distances and barycenters can be computed in closed form, we extend (Cheng et al., 2021) specifically relaxing the parameterization of the pure states as Gaussian distributions. We highlight issues related to the uniqueness in identifying the model parameters as well as uncertainties induced when estimating a dynamically evolving distribution from a limited number of samples. To ameliorate non-uniqueness, we introduce regularization that imposes temporal smoothness on the dynamics of the barycentric weights. A quantile-based approximation of the pure state distributions yields a finite dimensional estimation problem which we numerically solve using cyclic descent alternating between updates to the pure-state quantile functions and the barycentric weights. We demonstrate the utility of the proposed algorithm in segmenting both simulated and real world human activity time series.
MoreTranslated text
Key words
Wasserstein barycenter,displacement interpolation,dynamical model,sequential data,time series analysis,sliding window,non-parametric,quantile function,human activity analysis
PDF
View via Publisher
AI Read Science
AI Summary
AI Summary is the key point extracted automatically understanding the full text of the paper, including the background, methods, results, conclusions, icons and other key content, so that you can get the outline of the paper at a glance.
Example
Background
Key content
Introduction
Methods
Results
Related work
Fund
Key content
- Pretraining has recently greatly promoted the development of natural language processing (NLP)
- We show that M6 outperforms the baselines in multimodal downstream tasks, and the large M6 with 10 parameters can reach a better performance
- We propose a method called M6 that is able to process information of multiple modalities and perform both single-modal and cross-modal understanding and generation
- The model is scaled to large model with 10 billion parameters with sophisticated deployment, and the 10 -parameter M6-large is the largest pretrained model in Chinese
- Experimental results show that our proposed M6 outperforms the baseline in a number of downstream tasks concerning both single modality and multiple modalities We will continue the pretraining of extremely large models by increasing data to explore the limit of its performance
Try using models to generate summary,it takes about 60s
Must-Reading Tree
Example
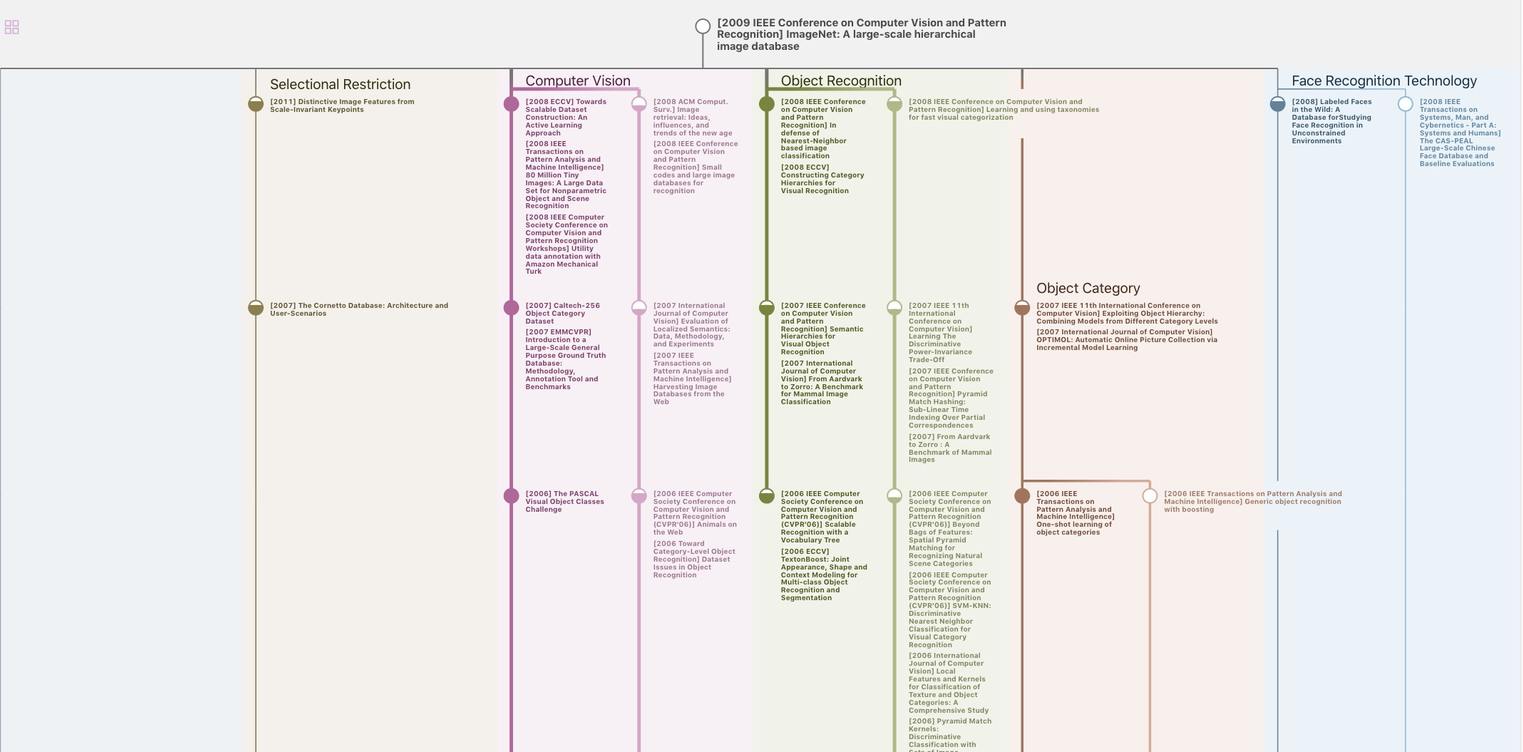
Generate MRT to find the research sequence of this paper
Related Papers
Modeling Changes in Molecular Dynamics Time Series As Wasserstein Barycentric Interpolations
2023 International Conference on Sampling Theory and Applications (SampTA) 2023
被引用0
Data Disclaimer
The page data are from open Internet sources, cooperative publishers and automatic analysis results through AI technology. We do not make any commitments and guarantees for the validity, accuracy, correctness, reliability, completeness and timeliness of the page data. If you have any questions, please contact us by email: report@aminer.cn
Chat Paper
GPU is busy, summary generation fails
Rerequest