HMGCL: Heterogeneous multigraph contrastive learning for LBSN friend recommendation
World Wide Web(2022)
摘要
Friend recommendation from user trajectory is a vital real-world application of location-based social networks (LBSN) services. Previous statistical analysis indicated that social network relationships could explain 10% to 30% of human movement, especially long-distance travel. Therefore, it is necessary to recognize patterns from human mobility to assist the friend recommendation. However, previous works either modelled friendships and check-in records by simple graphs with only one connection between any two nodes or ignored a large amount of vital spatio-temporal information and semantic information in raw LBSN data. To overcome the limitation of the simple graph commonly seen in previous works, we leverage heterogeneous multigraph to model LBSN data and define various semantic connections between nodes. Against this background, we propose a Heterogeneous Multigraph Contrastive Learning (HMGCL) model to capture spatio-temporal characteristics of human trajectories for user node embedding learning. Extensive experiments show that our method outperforms the state-of-the-art approaches in six real-world city datasets.
更多查看译文
关键词
Heterogeneou multigraph,Location-based social networks,Contrastive learning,Friend recommendation
AI 理解论文
溯源树
样例
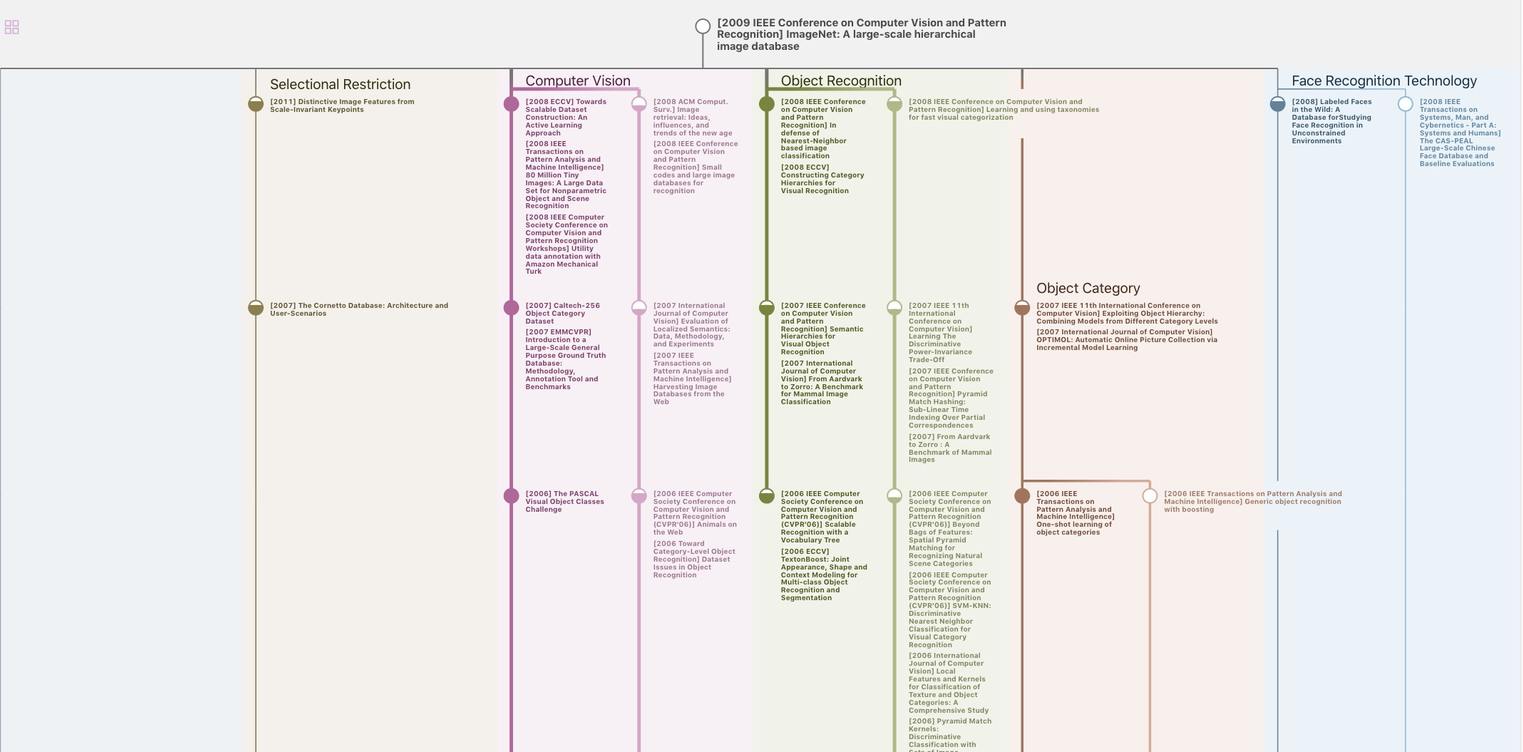
生成溯源树,研究论文发展脉络
Chat Paper
正在生成论文摘要