Image colorization using deep convolutional auto-encoder with multi-skip connections
Soft Computing(2022)
摘要
The colorization of grayscale images is a challenging task in image processing. Recently, deep learning has shown remarkable performance in image colorization. However, the detail loss and color distortion are still serious problem for most existing methods, and some useful features may be lost in the processes of various convolutional layers because of the vanishing gradient problem. Therefore, there is still a considerable space to reach the roof of image colorization. In this work, we propose a deep convolutional auto-encoder with special multi-skip connections for image colorization in YUV color space, and the specific contributions or designs of this work are shown as the following five points. First, a given gray image is used as the Y channel to input a deep learning model to predict U and V channel. Second, the adopted encoder-decoder consists of a main path and two branch paths, and the branch path has two skip connection ways that include one shortcut in each three layers and one shortcut in each six layers. Third, the convolutional kernel size is set as 2*2 that is a special consideration in the path of one shortcut in each six layers. Fourth, a composite loss function is proposed based on the mean square error and gradient that is defined to calculate the errors between the ground truth and the predicted result. Finally, we also discuss the reasonable network parameters, such as the way of shortcut connection, the convolutional kernel size of shortcut connection, and loss function parameters. Experiments on different image datasets show that the proposed image colorization model is effective, and the scores of the PNSR, RMSE, SSIM, and Pearson correlation coefficient are, respectively, to 27.0595, 0.1311, 0.561, and 0.9771.
更多查看译文
关键词
Auto-encoder,Convolutional neural network,Deep learning,Image processing,Image colorization,Residual neural network
AI 理解论文
溯源树
样例
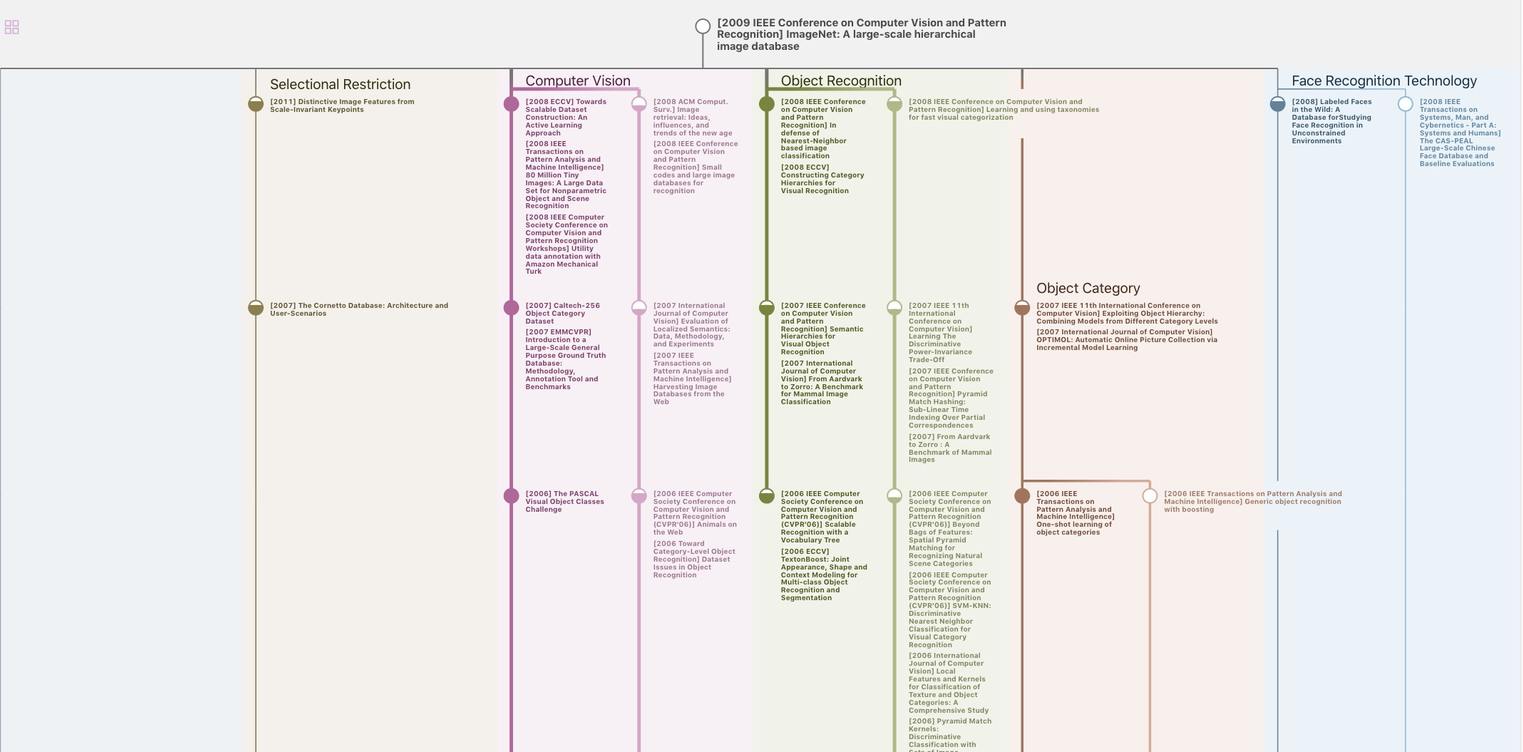
生成溯源树,研究论文发展脉络
Chat Paper
正在生成论文摘要