Estimation of minority modes of transportation based on machine learning approach
Procedia Computer Science(2022)
摘要
The modal choice, the third step of the historical four-stage model (FSM), determines the flows for each mode of transportation. It is generally stimated from the discrete choice models, which require re-building trips conditions for all alternatives, even those marginal. This paper proposes a new alternative based on machine learning methods and resampling techniques to estimate the modal choice by considering the extreme minority mode of transportation, such as intermodal combinations (e.g., car + public transit, bike + public transit). We have proposed two learning algorithms: the decision tree (DT) and the multinomial logistic regression (MNLR). Afer resampling the dataset, the DT presents better predictions (70% of global accuracy and f1-score closes to 70% for all modes of transportation) than the MNLR (35% of global accuracy).
更多查看译文
关键词
Modal choice,machine learning,discrete choice,intermodality
AI 理解论文
溯源树
样例
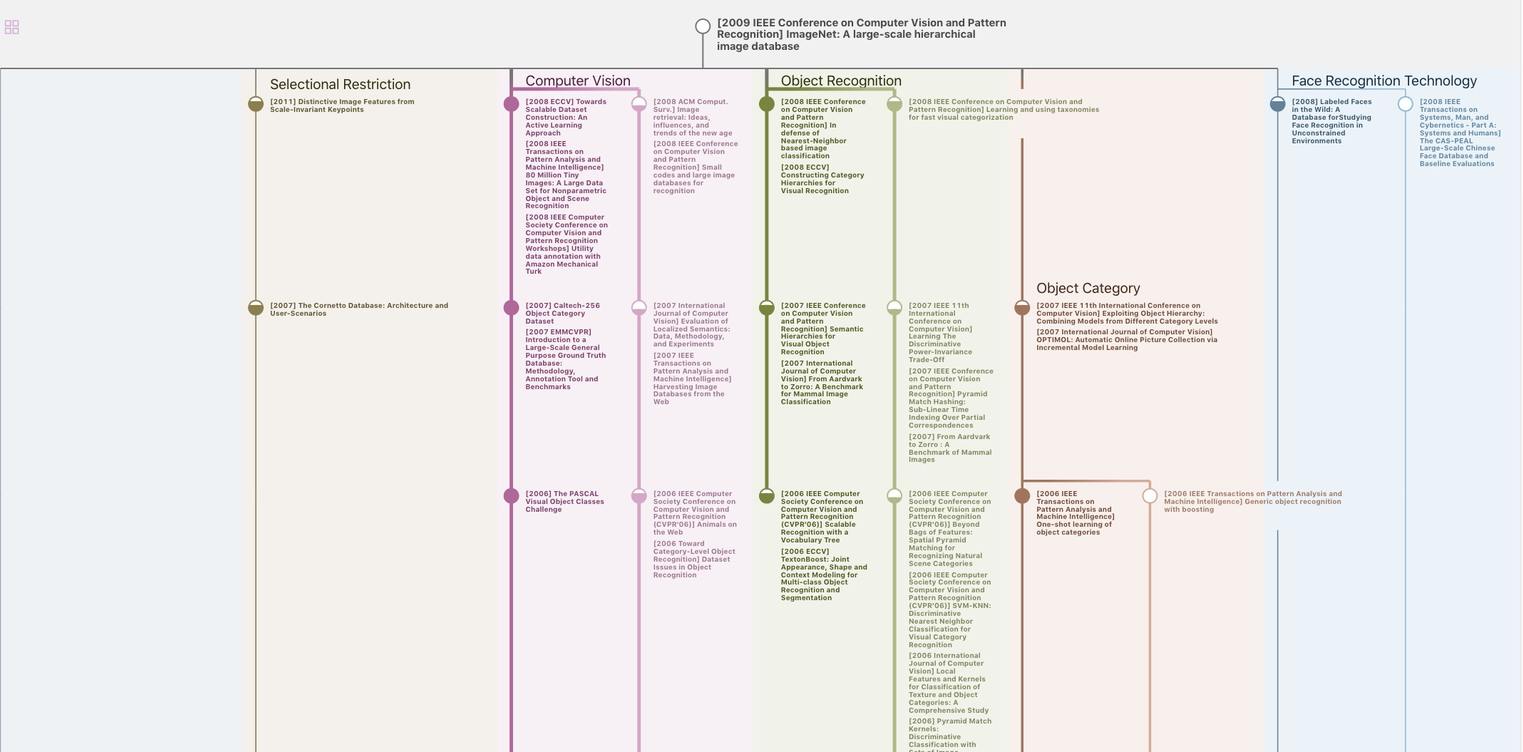
生成溯源树,研究论文发展脉络
Chat Paper
正在生成论文摘要