Localizing Anatomical Landmarks in Ocular Images Using Zoom-In Attentive Networks
OPHTHALMIC MEDICAL IMAGE ANALYSIS, OMIA 2022(2022)
摘要
Localizing anatomical landmarks are important tasks in medical image analysis. However, the landmarks to be localized often lack prominent visual features. Their locations are elusive and easily confused with the background, and thus precise localization highly depends on the context formed by their surrounding areas. In addition, the required precision is usually higher than segmentation and object detection tasks. Therefore, localization has its unique challenges different from segmentation or detection. In this paper, we propose a zoom-in attentive network (ZIAN) for anatomical landmark localization in ocular images. First, a coarse-to-fine, or "zoom-in" strategy is utilized to learn the contextualized features in different scales. Then, an attentive fusion module is adopted to aggregate multi-scale features, which consists of 1) a co-attention network with a multiple regions-of-interest (ROIs) scheme that learns complementary features from the multiple ROIs, 2) an attention-based fusion module which integrates the multi-ROIs features and non-ROI features. We evaluated ZIAN on two open challenge tasks, i.e., the fovea localization in fundus images and scleral spur localization in AS-OCT images. Experiments show that ZIAN achieves promising performances and outperforms state-of-the-art localization methods. The source code and trained models of ZIAN are available at https://github.com/leixiaofeng-astar/OMIA9-ZIAN.
更多查看译文
关键词
Fovea localization, Scleral spur localization, Self-attention
AI 理解论文
溯源树
样例
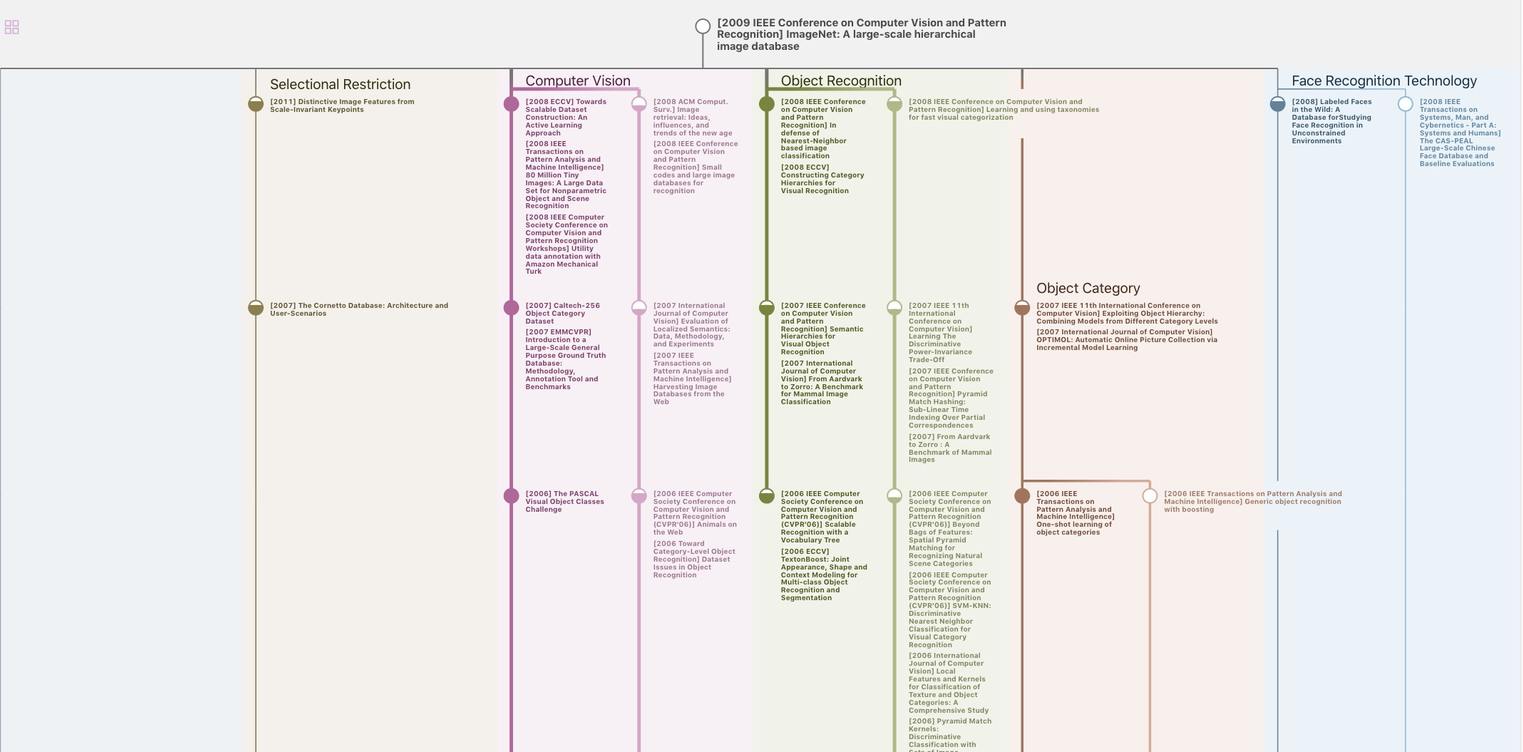
生成溯源树,研究论文发展脉络
Chat Paper
正在生成论文摘要