Analyzing historical diagnosis code data from NIH N3C and RECOVER Programs using deep learning to determine risk factors for Long Covid
arxiv(2022)
摘要
Post-acute sequelae of SARS-CoV-2 infection (PASC) or Long COVID is an emerging medical condition that has been observed in several patients with a positive diagnosis for COVID-19. Historical Electronic Health Records (EHR) like diagnosis codes, lab results and clinical notes have been analyzed using deep learning and have been used to predict future clinical events. In this paper, we propose an interpretable deep learning approach to analyze historical diagnosis code data from the National COVID Cohort Collective (N3C) to find the risk factors contributing to developing Long COVID. Using our deep learning approach, we are able to predict if a patient is suffering from Long COVID from a temporally ordered list of diagnosis codes up to 45 days post the first COVID positive test or diagnosis for each patient, with an accuracy of 70.48\%. We are then able to examine the trained model using Gradient-weighted Class Activation Mapping (GradCAM) to give each input diagnoses a score. The highest scored diagnosis were deemed to be the most important for making the correct prediction for a patient. We also propose a way to summarize these top diagnoses for each patient in our cohort and look at their temporal trends to determine which codes contribute towards a positive Long COVID diagnosis.
更多查看译文
关键词
COVID-19,EHR,deep learning,GradCAM
AI 理解论文
溯源树
样例
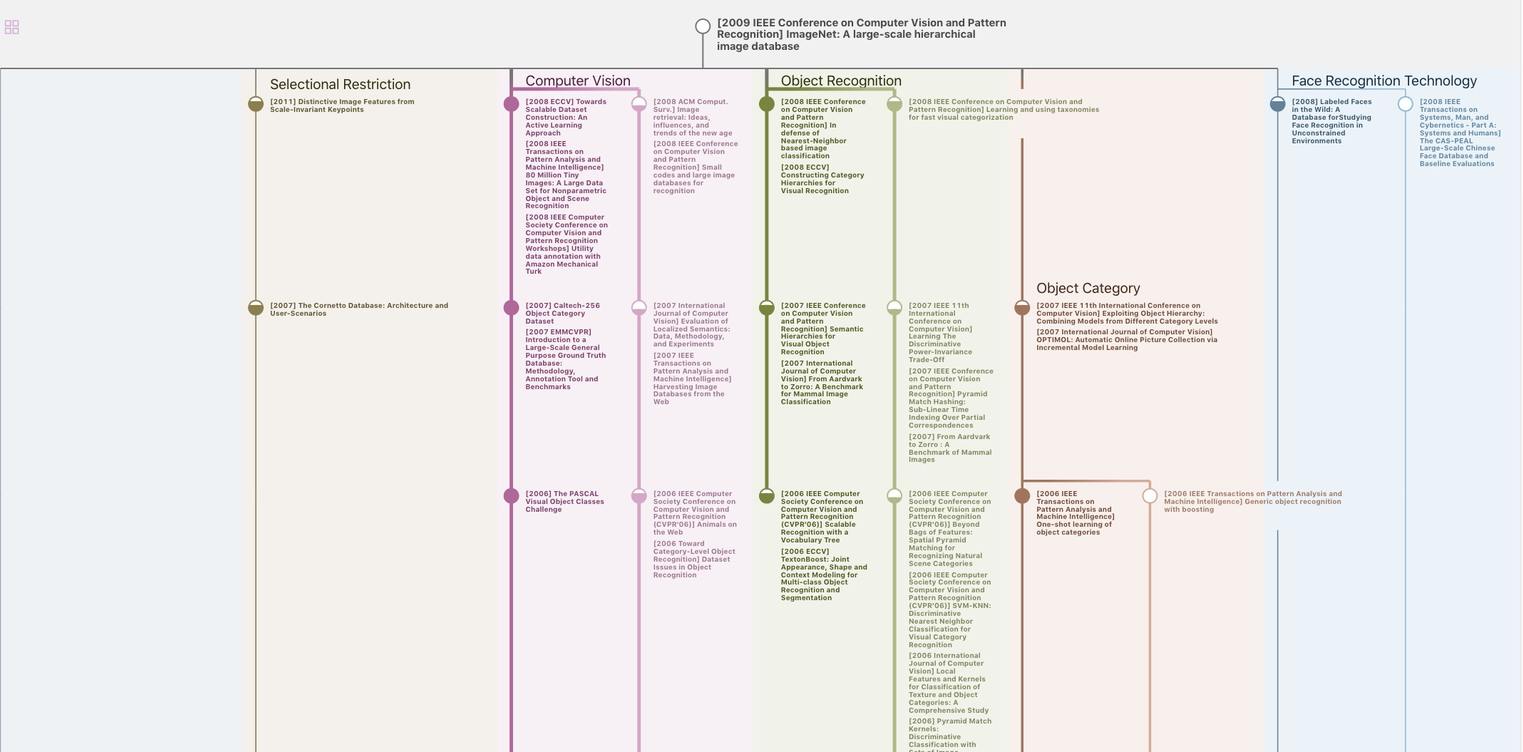
生成溯源树,研究论文发展脉络
Chat Paper
正在生成论文摘要