Env-Aware Anomaly Detection: Ignore Style Changes, Stay True to Content!
arxiv(2022)
摘要
We introduce a formalization and benchmark for the unsupervised anomaly detection task in the distribution-shift scenario. Our work builds upon the iWildCam dataset, and, to the best of our knowledge, we are the first to propose such an approach for visual data. We empirically validate that environment-aware methods perform better in such cases when compared with the basic Empirical Risk Minimization (ERM). We next propose an extension for generating positive samples for contrastive methods that considers the environment labels when training, improving the ERM baseline score by 8.7%.
更多查看译文
关键词
ignore style changes,detection,env-aware
AI 理解论文
溯源树
样例
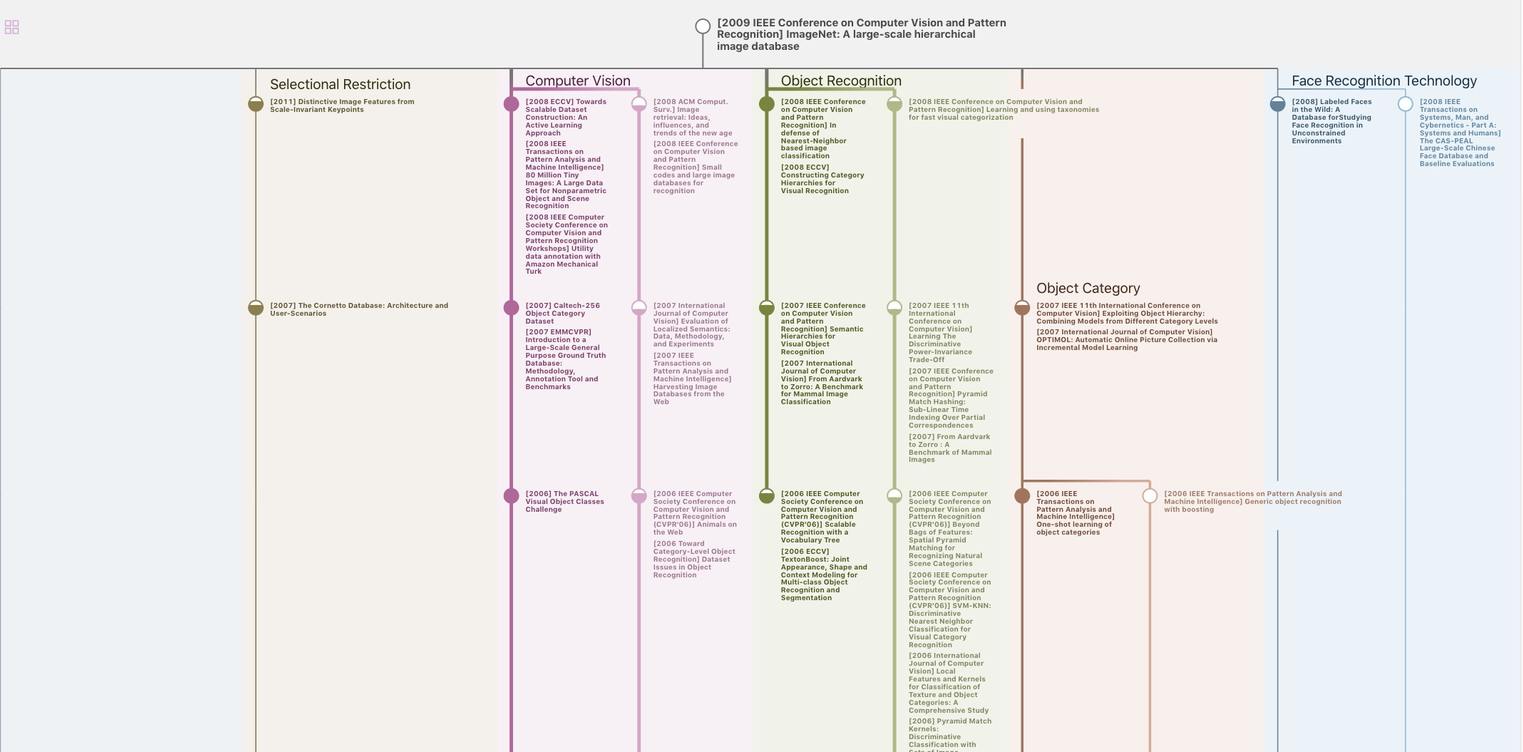
生成溯源树,研究论文发展脉络
Chat Paper
正在生成论文摘要