On the Effectiveness of Images in Multi-modal Text Classification: An Annotation Study
ACM Transactions on Asian and Low-Resource Language Information Processing(2022)
摘要
Combining different input modalities beyond text is a key challenge for natural language processing (NLP). Previous work has been inconclusive as to the true utility of images as a supplementary information source for text classification tasks, motivating this large-scale human study of labelling performance given text-only, images-only, or both text and images. To this end, we create a new dataset accompanied with a novel annotation method — Japanese Entity Labeling with Dynamic Annotation (JELDA) — to deepen our understanding of the effectiveness of images for multi-modal text classification. By performing careful comparative analysis of human performance and the performance of state-of-the-art (SOTA) multi-modal text classification models, we gain valuable insights into differences between human and model performance, and the conditions under which images are beneficial for text classification.
更多查看译文
关键词
Datasets,neural networks,natural language processing,text classification,multi-modality
AI 理解论文
溯源树
样例
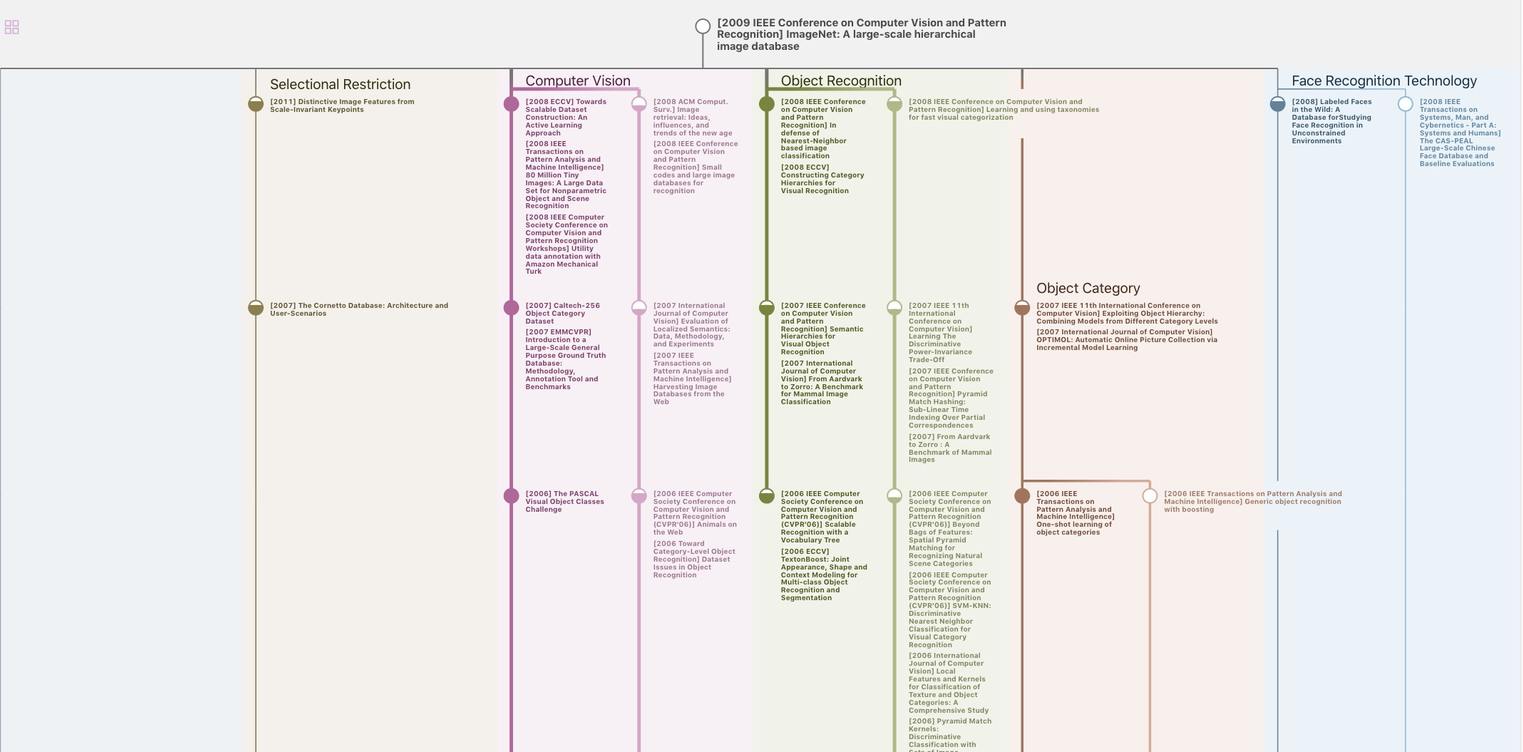
生成溯源树,研究论文发展脉络
Chat Paper
正在生成论文摘要