Robust image hashing for content identification through contrastive self-supervised learning
Neural Networks(2022)
摘要
Content identification systems are an essential technology for many applications. These systems identify query multimedia items using a database of known identities. A hash-based system uses a perceptual hashing function that generates a hash value invariant against a set of expected manipulations in an image, later compared to perform identification. Usually, this set of manipulations is well-known, and the researcher creates the perceptual hashing function that best adapts to the set. However, a new manipulation may break the hashing function, requiring to create a new one, which may be costly and time-consuming. Therefore, we propose to let the hashing function learn an invariant feature space automatically. For this, we exploit the recent advances in self-supervised learning, where a model uses unlabeled data to generate a feature representation by solving a metric learning-based pretext task that enforces the robust image hashing properties for content identification systems. To achieve model transferability on unseen data, our pretext task enforces the feature vector invariance against the manipulation set, and through random sampling on the unlabeled training set, we present the model a wide variety of perceptual information to work on. As exhaustive experimentation shows, this method achieves excellent robustness against a comprehensive set of manipulations, even difficult ones such as horizontal flip and rotation, with excellent identification performance. Also, the trained model is highly discriminative against the presence of near-duplicate images. Furthermore, this method does not need re-training or fine-tuning on a new dataset to achieve the observed performance, indicating an excellent generalization capacity.
更多查看译文
关键词
content identification,robust image,self-supervised
AI 理解论文
溯源树
样例
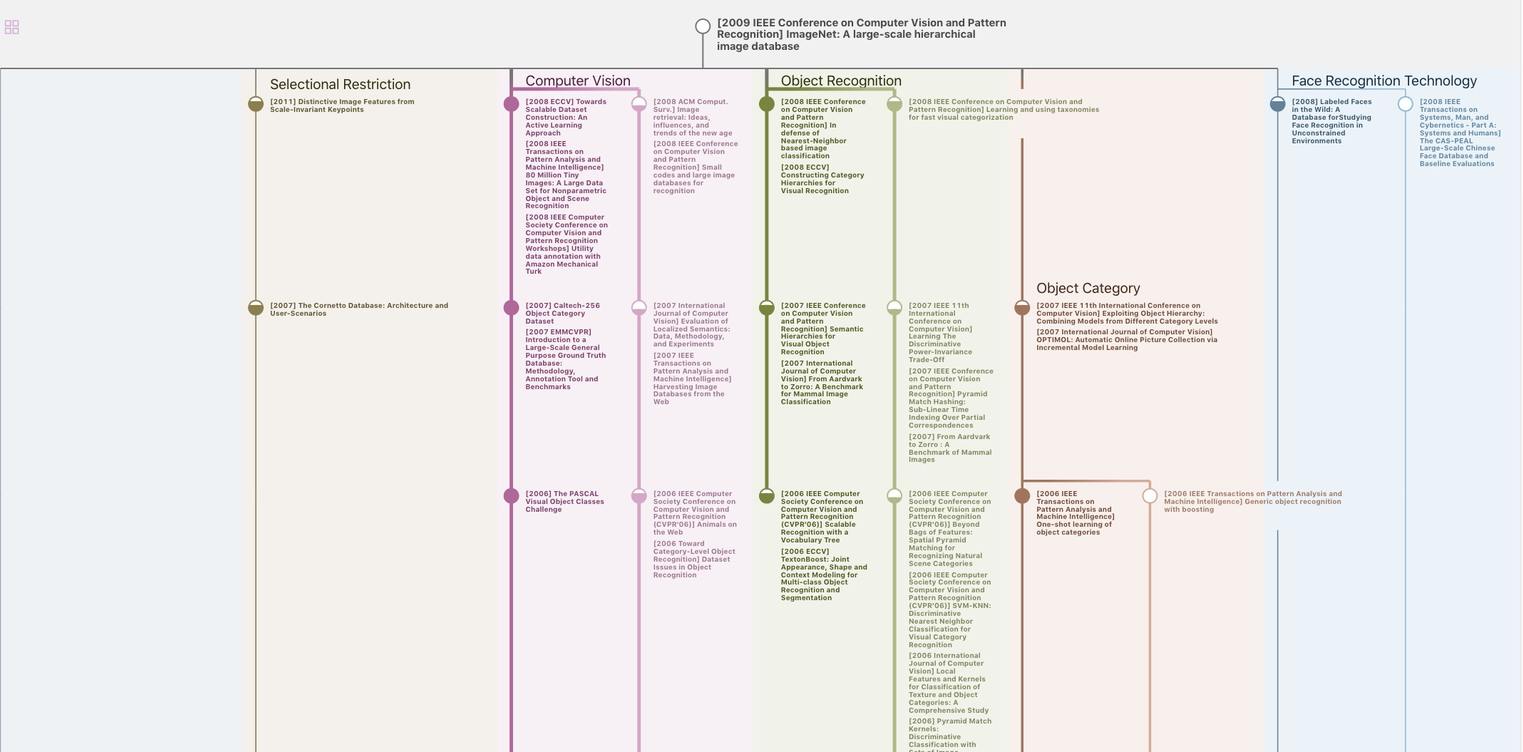
生成溯源树,研究论文发展脉络
Chat Paper
正在生成论文摘要