Assessing a soil-removed semi-empirical model for estimating leaf chlorophyll content
Remote Sensing of Environment(2022)
摘要
Leaf chlorophyll content (LCC) is an important indicator of foliar nitrogen status and photosynthetic capacity. Compared to physical models, the generality of empirical models based on vegetation indices is often questioned when they are used to estimate LCC due to the influence from canopy structure, such as leaf area index (LAI). A recent study developed the LAI-insensitive chlorophyll index (LICI) and established a semi-empirical LICI-based LCC quantification model, which inherits both the robustness of physical models and the simplicity of empirical models. However, it is unclear whether such a simple model is as accurate and generic as physical models. Here, we adopted an innovative approach to disentangle the confounding effects of LAI and LCC on LICI and found that LICI was strongly correlated to LCC but only marginally sensitive to LAI. Moreover, we also found that LICI was sensitive to the soil background and thus proposed a spectral separation of soil and vegetation (3SV) algorithm, which is automatic and does not require prior information of soil background. After implementing the 3SV algorithm to remove the contributed reflectance of soil, we then obtained the contributed reflectance of vegetation (CRv). Model simulations showed that the soil background effect on the CRv-derived LICI was largely eliminated and hence this index was viewed to be soil-removed. As a result, the accuracy and generality of the soil-removed LICI-based model for LCC estimation was evaluated using comprehensive datasets from multiple vegetation types, years, sites, and observation platforms and compared to that of a MatrixVI-based physical model and a MERIS terrestrial chlorophyll index (MTCI)-based semi-empirical model. The root-mean-square error (RMSE) for LCC estimated by the soil-removed LICI-based model was 6.22–6.87 μg/cm2 for the crop datasets and 10.68 μg/cm2 for the multi-ecosystem dataset when the equivalent wet soil fraction was <0.7. Although further efforts are required to mitigate the effects of soil on the LICI-based model over sparse vegetation, this research is highly beneficial for extending its potential applications to the globe and advancing the development of an operational LCC monitoring system in the emerging satellite hyperspectral era.
更多查看译文
关键词
Leaf chlorophyll content,Physical model,Semi-empirical model,LICI,Soil effect
AI 理解论文
溯源树
样例
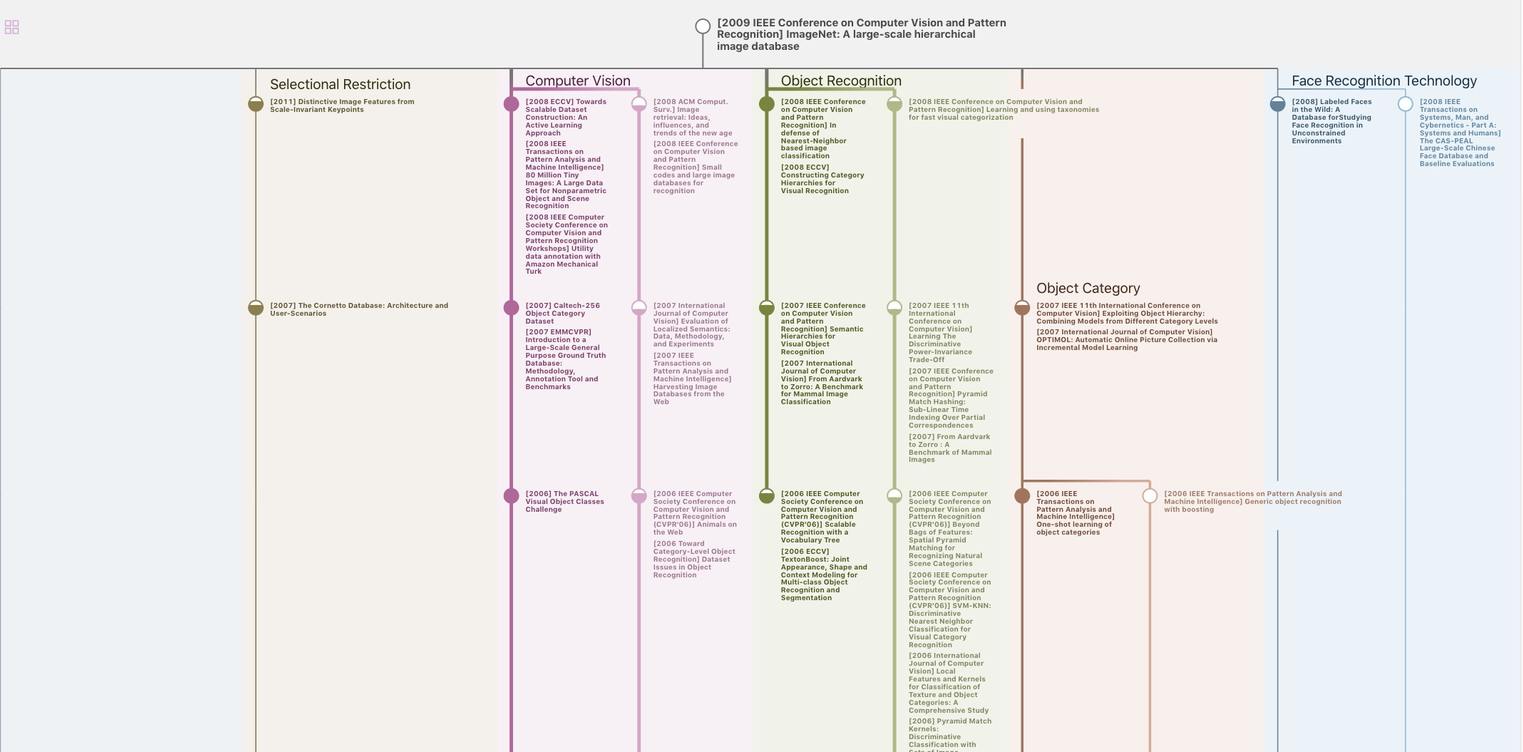
生成溯源树,研究论文发展脉络
Chat Paper
正在生成论文摘要