Variational convolutional neural networks classifiers
Physica A: Statistical Mechanics and its Applications(2022)
摘要
Convolutional neural networks have been shown to extract features better than traditional algorithms in the fields such as image classification, object detection, and speech recognition. In parallel, a variational quantum algorithm incorporating parameterized quantum circuits has higher performance on near-term quantum processors. In this paper, we propose a classification algorithm called variational convolutional neural networks (VCNN), allowing for efficient training and implementation on near-term quantum devices. The VCNN algorithm combines the multi-scale entanglement renormalization ansatz. We deploy the VCNN algorithm on the TensorFlow Quantum platform with the numerical simulator backends using the MNIST and Fashion MNIST datasets. Experimental results show that the average accuracy of VCNN on classification tasks can reach up to 96.41%. Our algorithm has higher learning accuracy and fewer training epochs than quantum neural network algorithms. Moreover, we conclude that circuit-based models have excellent resilience to noise by numerical simulations.
更多查看译文
关键词
Quantum computing,Quantum machine learning,Convolutional neural networks,Parameterized quantum circuits
AI 理解论文
溯源树
样例
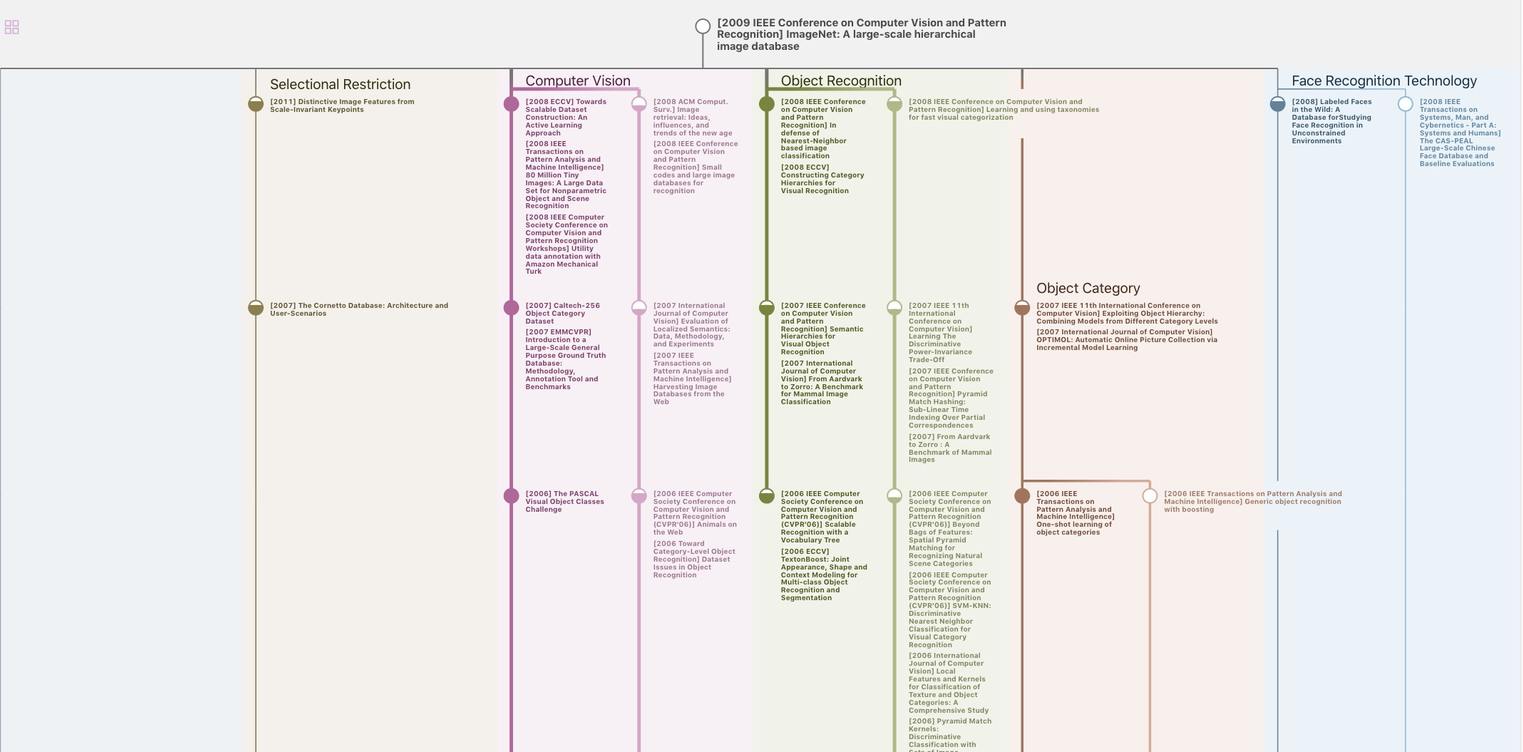
生成溯源树,研究论文发展脉络
Chat Paper
正在生成论文摘要