EAF-SR: an enhanced autoencoder framework for social recommendation
Multimedia Tools and Applications(2022)
摘要
In the recommendation systems, the user feedback data (e.g., user-item rating and the social information) is usually sparse, discrete and full of noise. However, most of existing methods use it to make recommendations directly, which leads to the reduction of their recommendation accuracy and quality ultimately. To address this problem, this paper proposes an Enhanced Autoencoder Framework with knowledge distillation (EAF-SR) for learning robust information from user feedback data, where it consists of three parts: Pre-training, knowledge distillation layers and Re-training. Specifically, Pre-training is proposed to generate the soft targets by using the stacked denoising autoencoder (SDAE) from user feedbacks and trust information, which aims to reduce data noise. Then, knowledge distillation layers are developed to learn the robust information from the generated soft targets. Finally, Re-training network combined with Pre-training is designed to make recommendations for each user. Extensive experiments done on three real-world datasets (e.g., Flixster, Epinions and Douban) show that the proposed EAF-SR is superior to the state-of-the-art approaches.
更多查看译文
关键词
Deep learning,Recommender system,Stacked denoising autoencoder,Knowledge distillation,Social recommendation
AI 理解论文
溯源树
样例
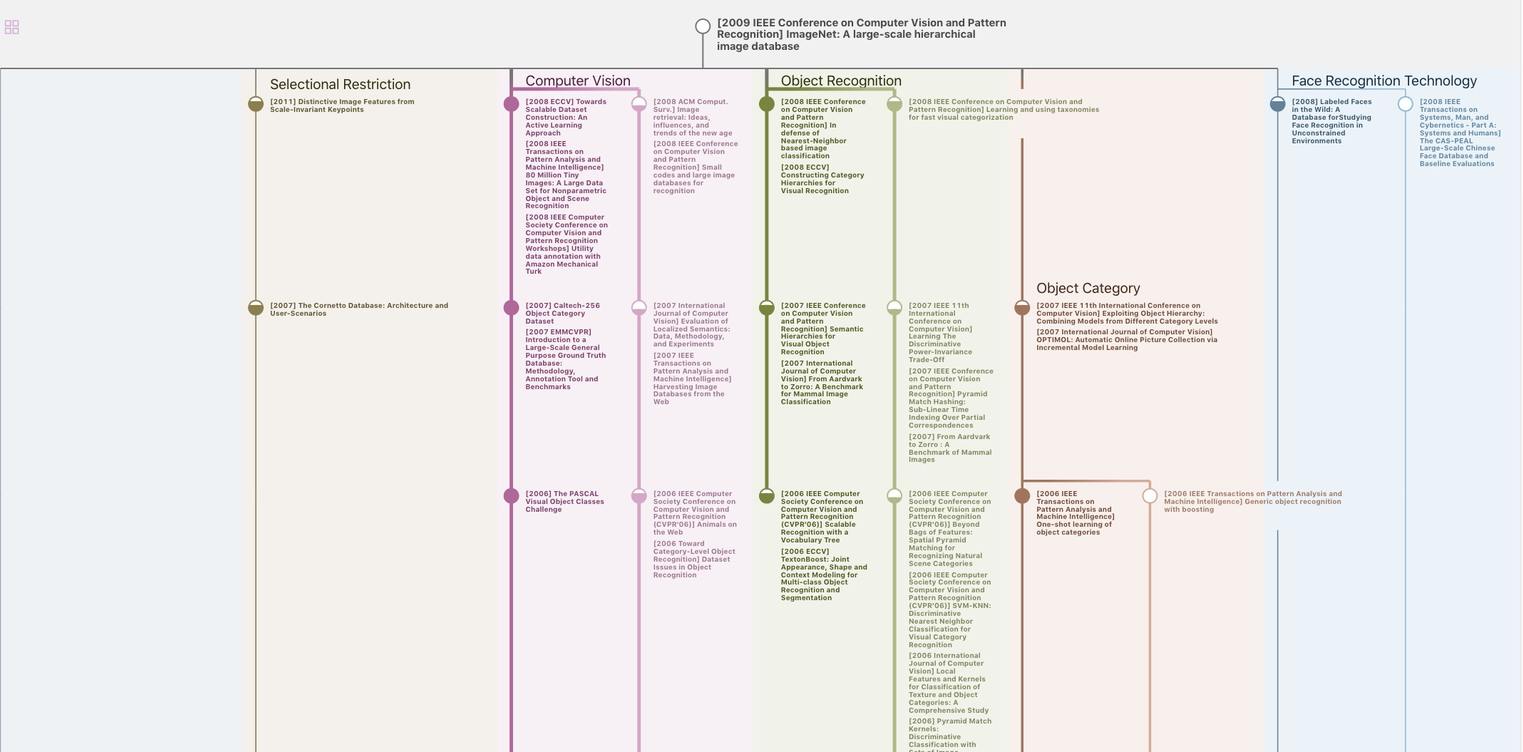
生成溯源树,研究论文发展脉络
Chat Paper
正在生成论文摘要