A novel depth search based light weight CAR network for the segmentation of brain tumour from MR images
Neuroscience Informatics(2022)
摘要
In this modern era, brain tumour is one of the dreadful diseases that occur due to the growth of abnormal cells or by the accumulation of dead cells in the brain. If these abnormalities are not detected in the early stages, they lead to severe conditions and may cause death to the patients. With the advancement of medical imaging, Magnetic Resonance Images (MRI) are developed to analyze the patients manually. However, this manual screening is prone to errors. To overcome this, a novel depth search-based network termed light weight channel attention and residual network (LWCAR-Net) is proposed by integrating with a novel depth search block (DSB) and a CAR module. The depth search block extracts the pertinent features by performing a series of convolution operations enabling the network to restore low-level information at every stage. On other hand, CAR module in decoding path refines the feature maps to increase the representation and generalization abilities of the network. This allows the network to locate the brain tumor pixels from MRI images more precisely. The performance of the depth search based LWCAR-Net is estimated by testing on different globally available datasets like BraTs 2020 and Kaggle LGG dataset. This method achieved a sensitivity of 95%, specificity of 99%, the accuracy of 99.97%, and dice coefficient of 95% respectively. Furthermore, the proposed model outperformed the existing state-of-the-art models like U-Net++, SegNet, etc by achieving an AUC of 98% in segmenting the brain tumour cells.
更多查看译文
关键词
Brain tumour,Magnetic Resonance Images (MRI),Light weight channel attention and residual network (LWCAR-Net),Depth Search Block (DSB),BraTs dataset
AI 理解论文
溯源树
样例
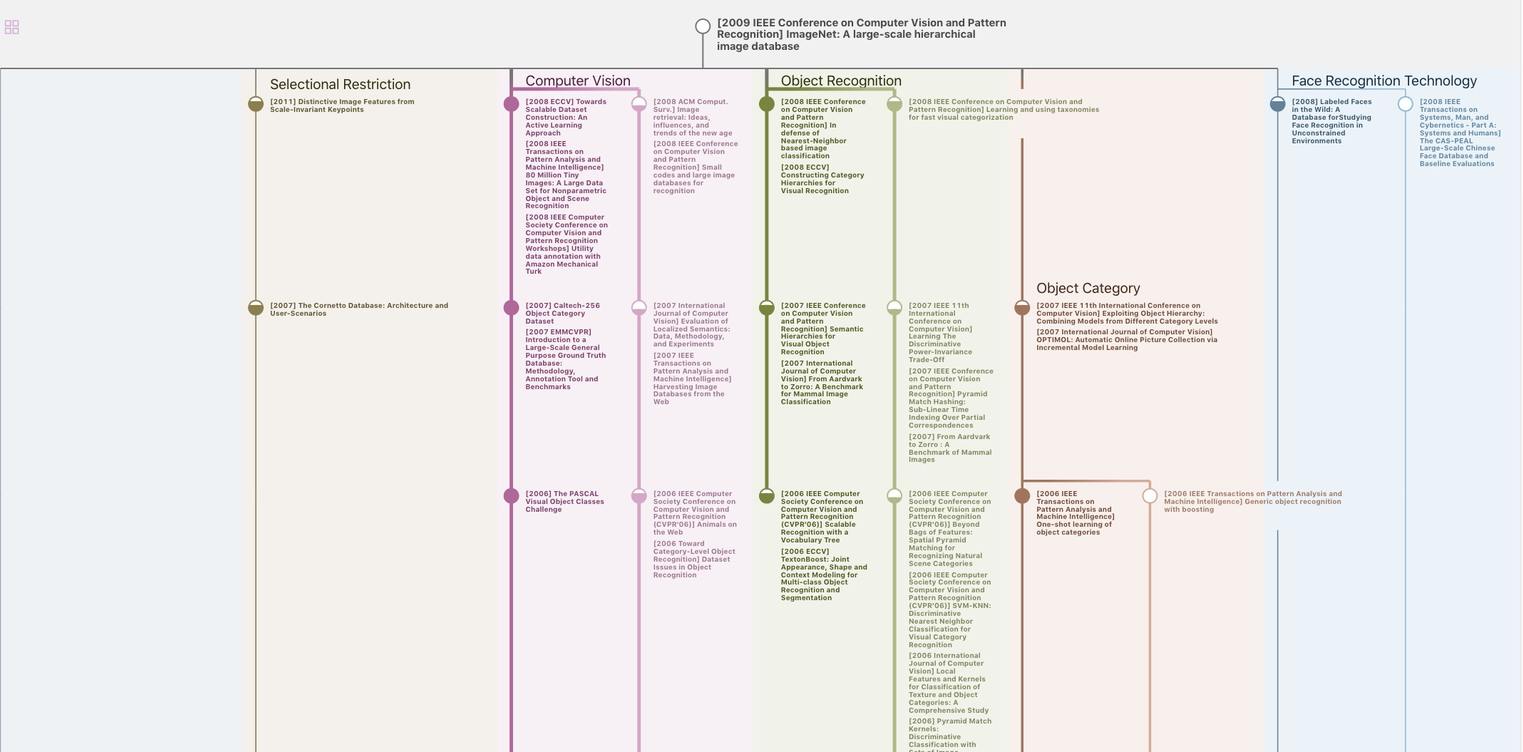
生成溯源树,研究论文发展脉络
Chat Paper
正在生成论文摘要