Commuting-pattern-oriented Optimal Sizing of Electric Vehicle Powertrain Based on Stochastic Optimization
Journal of Power Sources(2022)
关键词
Electric vehicle,Stochastic optimization,Powertrain sizing,Energy management strategy,Traffic dynamics
AI 理解论文
溯源树
样例
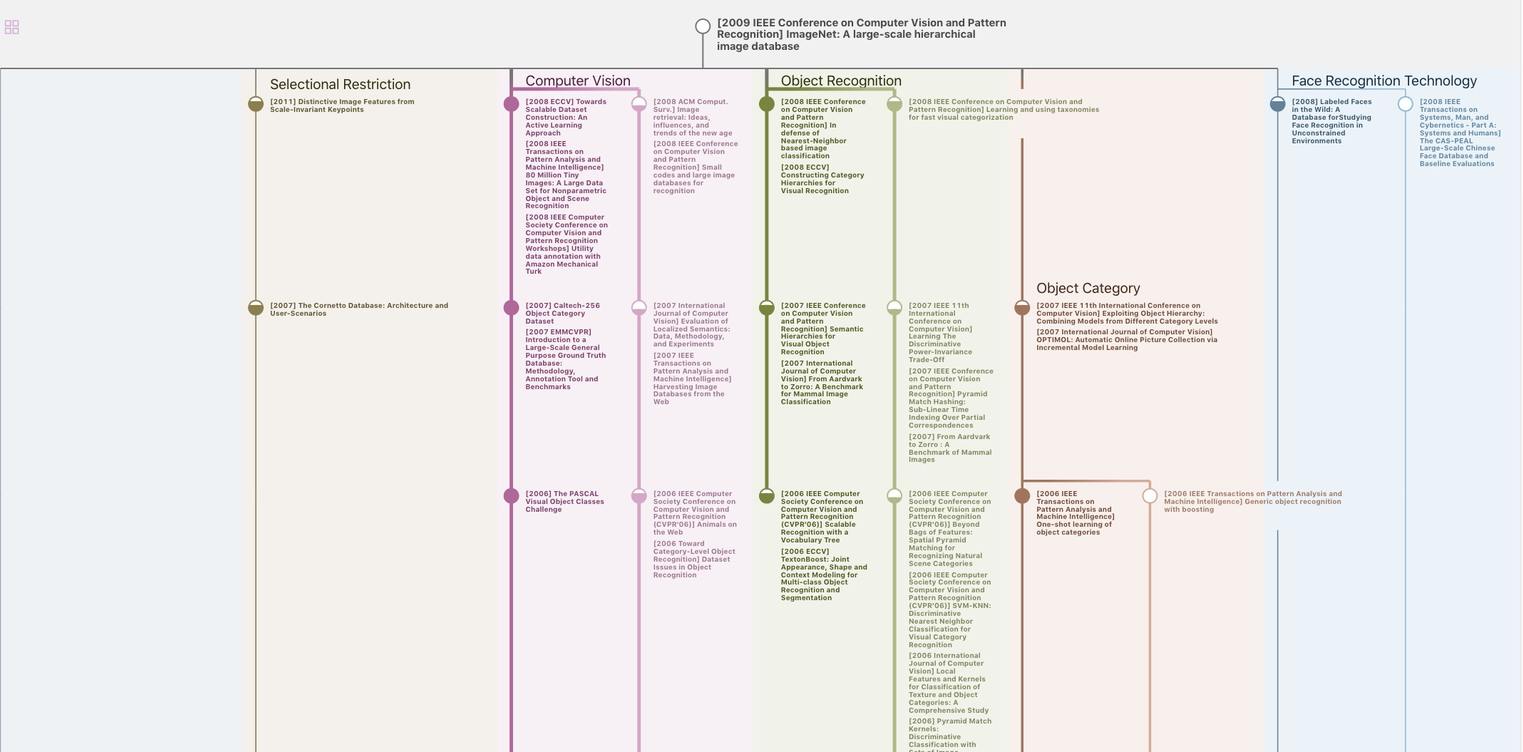
生成溯源树,研究论文发展脉络
Chat Paper
正在生成论文摘要