Data-driven Physics-Informed Interpolation Evolution Combining Historical-Predicted Knowledge for Remaining Oil Distribution Prediction
Journal of petroleum science & engineering(2022)
Abstract
The remaining oil distribution plays an important role in enhanced oil recovery (EOR), which directly guides the development of an oil reservoir in the middle and later stages. However, it is still challenging to accurately and efficiently characterize the distribution of remaining oil due to the complex reservoir geology. We propose a data-driven physics-informed interpolation evolution algorithm combining historical-predicted knowledge (DPIE-HPK) for the prediction of remaining oil distribution totally based on reservoir monitoring data. As a key step towards the remaining oil distribution, the production rates can be predicted as well. A physics-informed data supplement (PIDS) process is also presented to assist the DPIE-HPK algorithm. Both historical physics information and future information are used in the DPIE-HPK framework. The historical physical information is preprocessed by the PIDS, and future information is predicted by Long Short-Term Memory (LSTM) deep learning models. As a crucial interpolation evolution method, the Kriging method is also integrated into the framework to evolve the unknown spatial information. We test the DPIE-HPK framework for the prediction of the remaining oil distribution of a typical tight carbonate oil reservoir located in the Middle East. It is concluded that the DPIE-HPK framework is a fast, accurate and efficient intelligence tool for predicting the remaining oil distribution.
MoreTranslated text
Key words
Remaining oil distribution,EOR,DPIE-HPK,PIDS,LSTM,Kriging
AI Read Science
Must-Reading Tree
Example
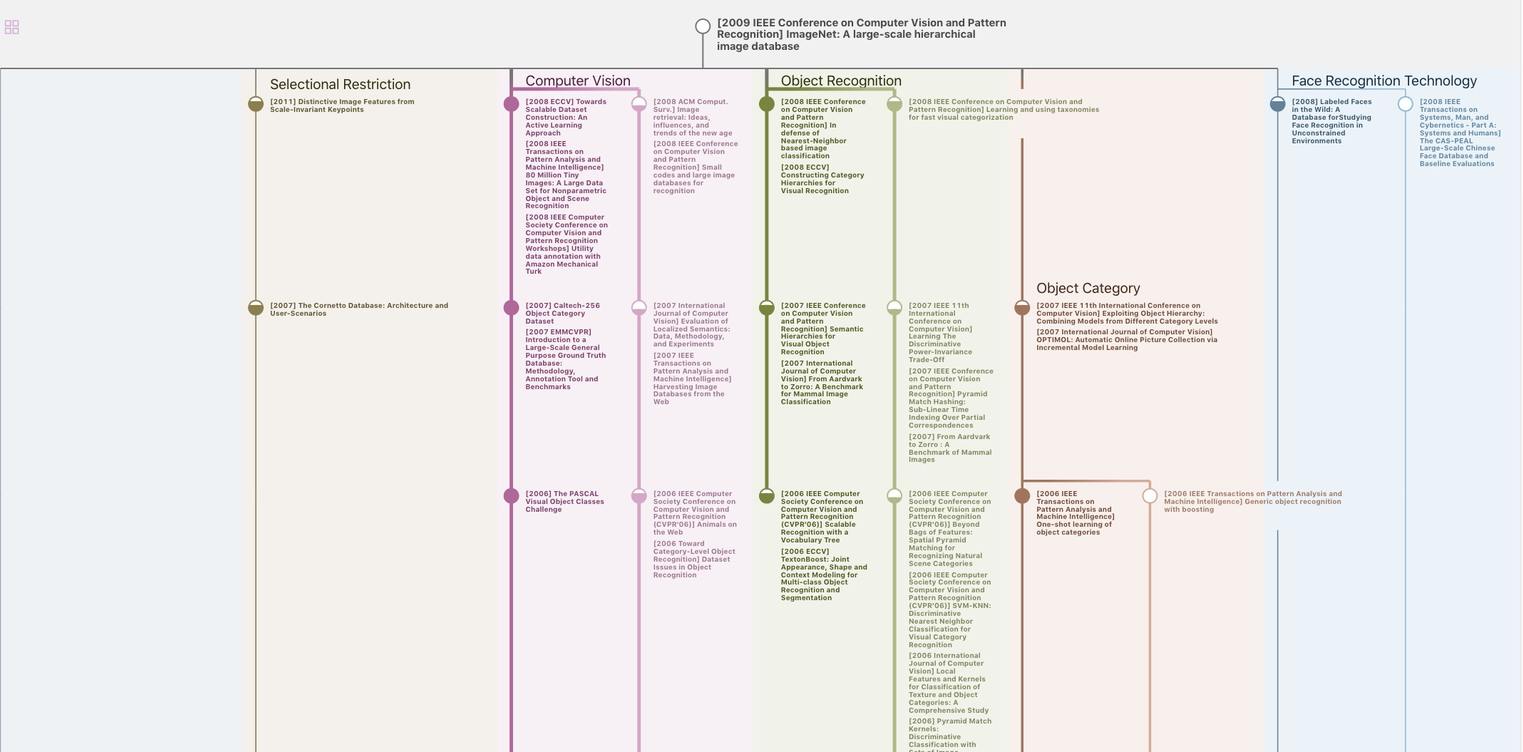
Generate MRT to find the research sequence of this paper
Chat Paper
Summary is being generated by the instructions you defined