Prognostics of Remaining Useful Life for Lithium-Ion Batteries Based on Hybrid Approach of Linear Pattern Extraction and Nonlinear Relationship Mining
IEEE Transactions on Power Electronics(2023)
摘要
The accurate prediction of the remaining useful life (RUL) of lithium-ion batteries (LIBs) is a key, challenging research direction. In this study, a battery degradation model is built based on the LIB dataset of NASA. A data decomposition prediction method, which extracts the linear trend from the capacity degradation data and then predicts the residuals of time series with nonlinear relations, is proposed. Then, an autoregressive integrated moving average-long short-term memory (ARIMA-LSTM) combined model for predicting the RUL of LIBs is established. The linear trend of capacity degradation is predicted by the ARIMA model and the LSTM model is established to predict the nonlinear residuals separated from the capacity degradation. The summation of both obtains the final battery RUL prediction result. Compared with the LSTM model and prediction models of similar types in existing literature in recent two years, the experimental results show that the RUL prediction error of the proposed model in this article is no more than one cycle, which is smaller than that of all the models involved in the comparison. Thus, this method has higher prediction accuracy and model generalization ability in the prediction of the remaining useful life of LIBs.
更多查看译文
关键词
Predictive models,Degradation,Mathematical models,Data models,Market research,Lithium-ion batteries,Time series analysis,Autoregressive integrated moving average-long short-term memory (ARIMA-LSTM) combined model,linear and nonlinear pattern decomposition,lithium-ion batteries (LIBs),remaining useful life (RUL)
AI 理解论文
溯源树
样例
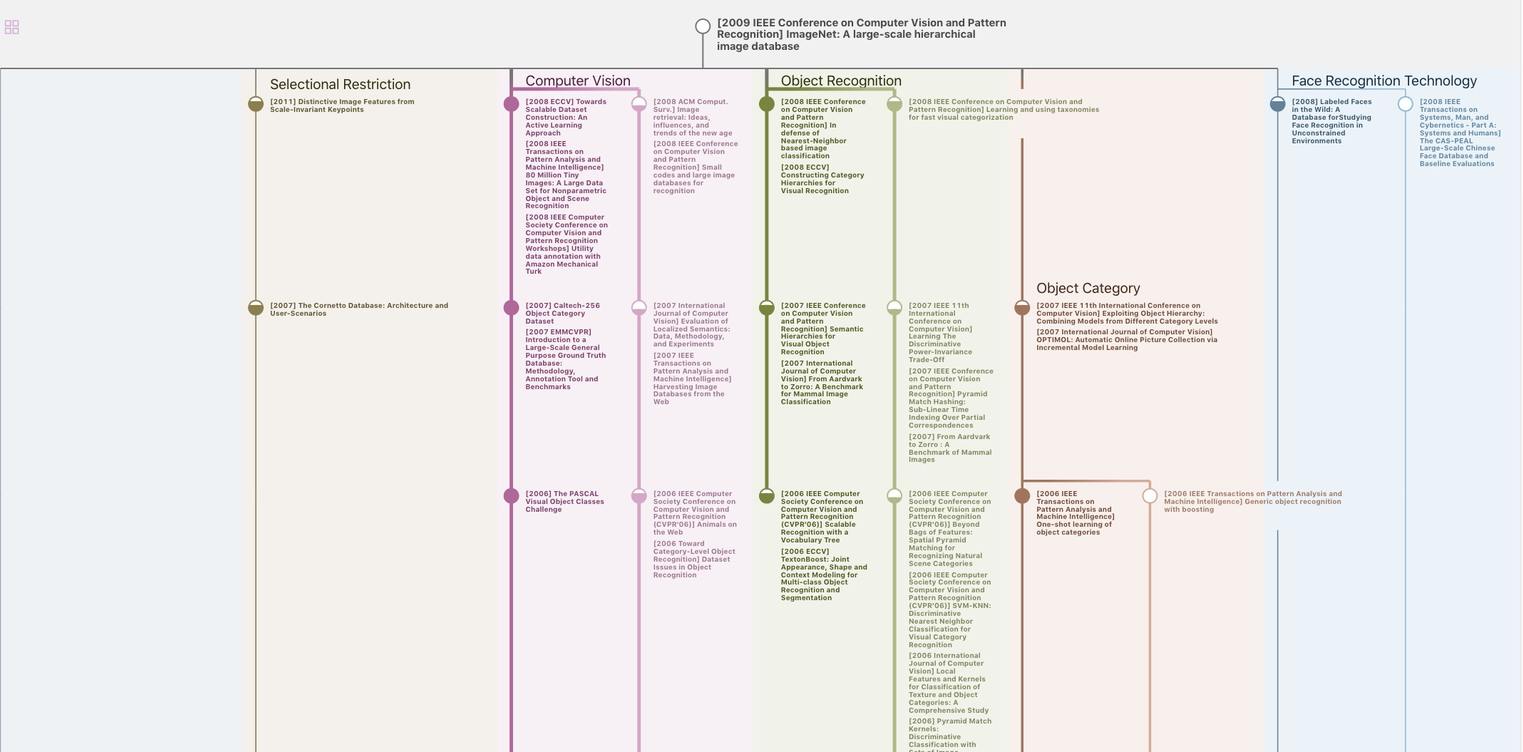
生成溯源树,研究论文发展脉络
Chat Paper
正在生成论文摘要