Tool wear prediction in edge trimming of carbon fiber reinforced polymer using machine learning with instantaneous parameters
Journal of Manufacturing Processes(2022)
摘要
Rapid tool wear occurs during the edge trimming of CFRP components due to the high strength and abrasive nature of the carbon fibers, leading to potential surface damage and delamination. This paper presents a hybrid approach by combining the machine learning technique and the physics-based kinematics model for tool wear prediction in edge trimming of CFRP. For edge trimming of unidirectional (UD) CFRP, the feature parameters of the machine learning models consist of the instantaneous fiber cutting angle, uncut chip thickness, and instantaneous radial force instead of the average force of a certain cutting period. The training data from UD CFRP is used to predict the overall radial force in edge trimming of multidirectional (MD) CFRP considering the nonuniform wear length distribution along the tool edge due to different fiber orientation angles of the UD layers. The machined surface, tool edge profile, and chip morphology were examined to demonstrate the nonuniform tool wear length and its effect on surface quality in edge trimming of MD CFRP. The tool wear monitoring in edge trimming of MD CFRPs with various lay-up configurations is achieved without the need for data training experiments on MD CFRP. The predictions from the machine learning methods are compared with experimental results in edge trimming of UD and MD CFRPs. As a result, the proposed method is able to reduce the experimental cost, considering the increased dimensionality if experimental data training of various fiber orientations of UD CFRP and various fiber lay-up configurations of MD CFRP is required.
更多查看译文
关键词
CFRP,GFRP,MRA,ANFIS,UD,DWT,ANN,AE,TOPSIS,CNN,SBULSTM,PCNN,MD,RF,FF,MSE,MAPE,R2,SEM
AI 理解论文
溯源树
样例
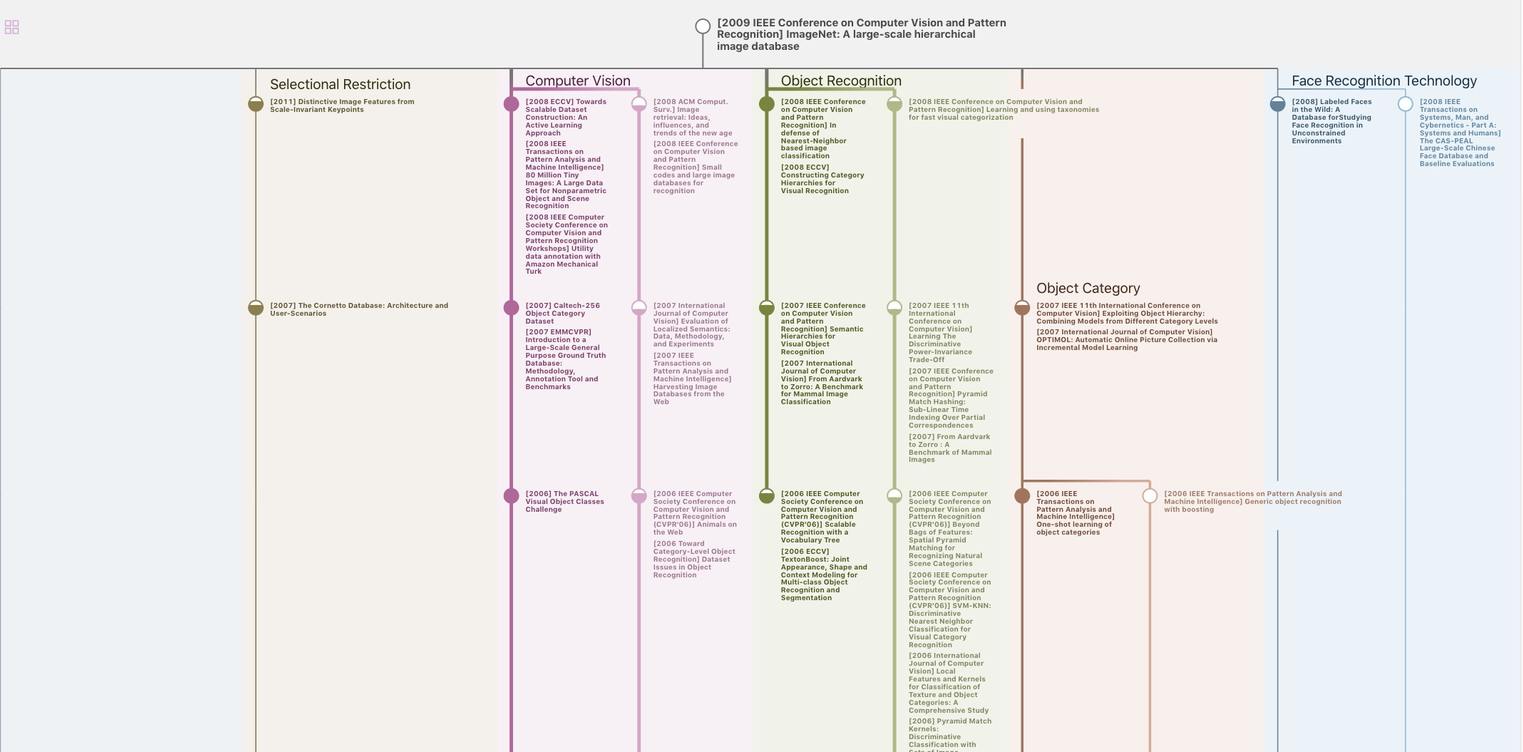
生成溯源树,研究论文发展脉络
Chat Paper
正在生成论文摘要