Cross-domain image translation with a novel style-guided diversity loss design
Knowledge-Based Systems(2022)
摘要
Cross-domain image-to-image translation has made remarkable progress in recent years. It aims to map the image from the original image domain to the target domains so that the image can appear in diverse styles. Currently, existing methods are mainly based on Generative Adversarial Networks (GAN). They often employ an auxiliary encoder to extract style features from noises or reference images for the generator to translate new images. However, these approaches are usually feasible for two-domain translation and present low diversity in multi-domain translation since the extracted style features are simply served as additional input to the generator rather than fully utilized. This paper proposes a style-guided image-to-image translation (SG-I2IT) with a novel diversity regularization term named style-guided diversity loss (SD loss), making the best of the extracted style features. In our model, style features not only serve as the generator’s input but also penalize the generator through the new SD loss, thus encouraging the model to capture the image styles better. The effectiveness of our method is demonstrated from two perspectives, noise-based and reference-based image translation. Qualitative and quantitative experiments validate our superiority of the proposed method against the state-of-the-art methods in terms of image quality and diversity. In addition, a user study demonstrates that the proposed method can better capture image styles and translate more realistic images.
更多查看译文
关键词
Cross-domain image-to-image (I2I) translation,Extracted style features,Generative Adversarial Networks (GAN),Multimodal,Multiple-domain
AI 理解论文
溯源树
样例
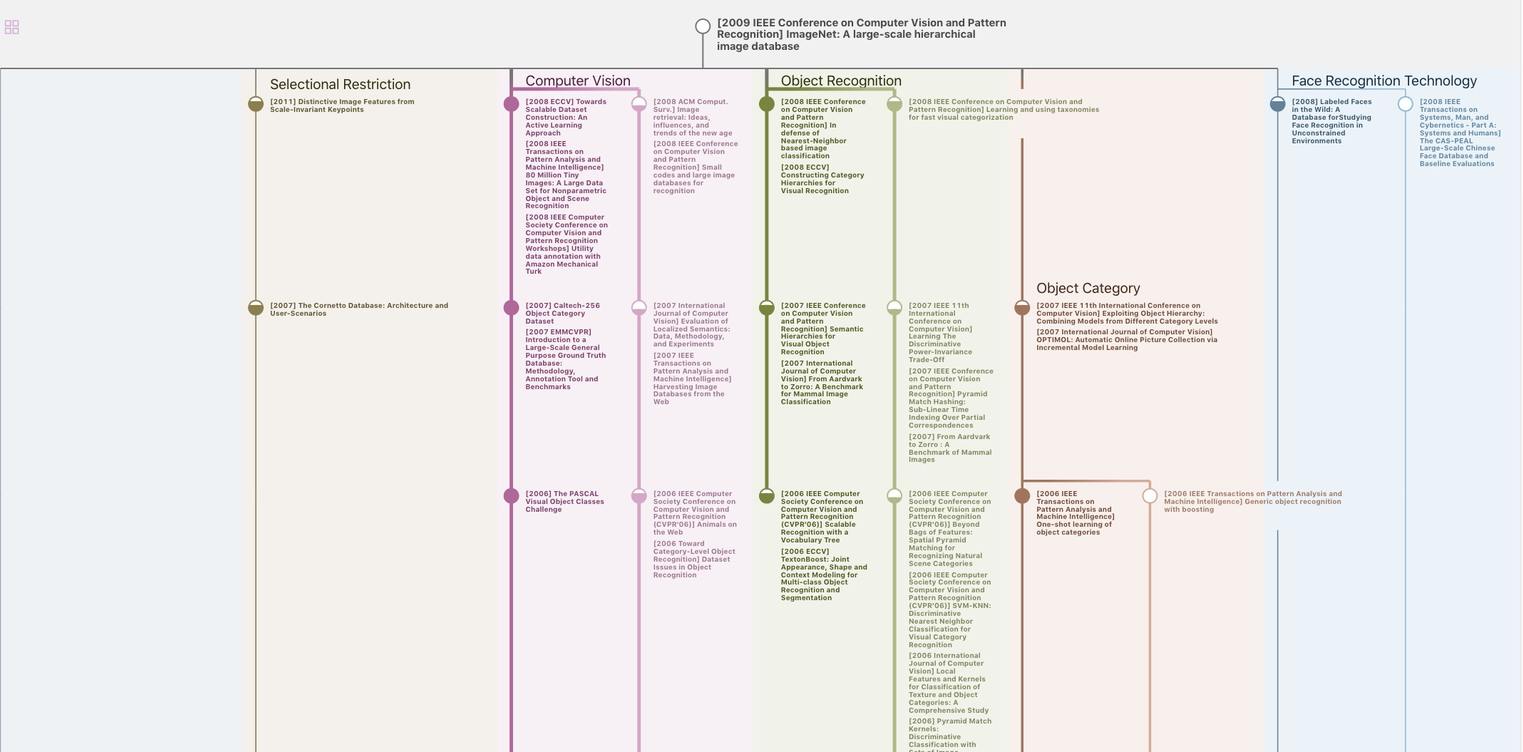
生成溯源树,研究论文发展脉络
Chat Paper
正在生成论文摘要