Neighbor Regularized Bayesian Optimization for Hyperparameter Optimization
arxiv(2022)
摘要
Bayesian Optimization (BO) is a common solution to search optimal hyperparameters based on sample observations of a machine learning model. Existing BO algorithms could converge slowly even collapse when the potential observation noise misdirects the optimization. In this paper, we propose a novel BO algorithm called Neighbor Regularized Bayesian Optimization (NRBO) to solve the problem. We first propose a neighbor-based regularization to smooth each sample observation, which could reduce the observation noise efficiently without any extra training cost. Since the neighbor regularization highly depends on the sample density of a neighbor area, we further design a density-based acquisition function to adjust the acquisition reward and obtain more stable statistics. In addition, we design a adjustment mechanism to ensure the framework maintains a reasonable regularization strength and density reward conditioned on remaining computation resources. We conduct experiments on the bayesmark benchmark and important computer vision benchmarks such as ImageNet and COCO. Extensive experiments demonstrate the effectiveness of NRBO and it consistently outperforms other state-of-the-art methods.
更多查看译文
关键词
bayesian optimization,hyperparameter
AI 理解论文
溯源树
样例
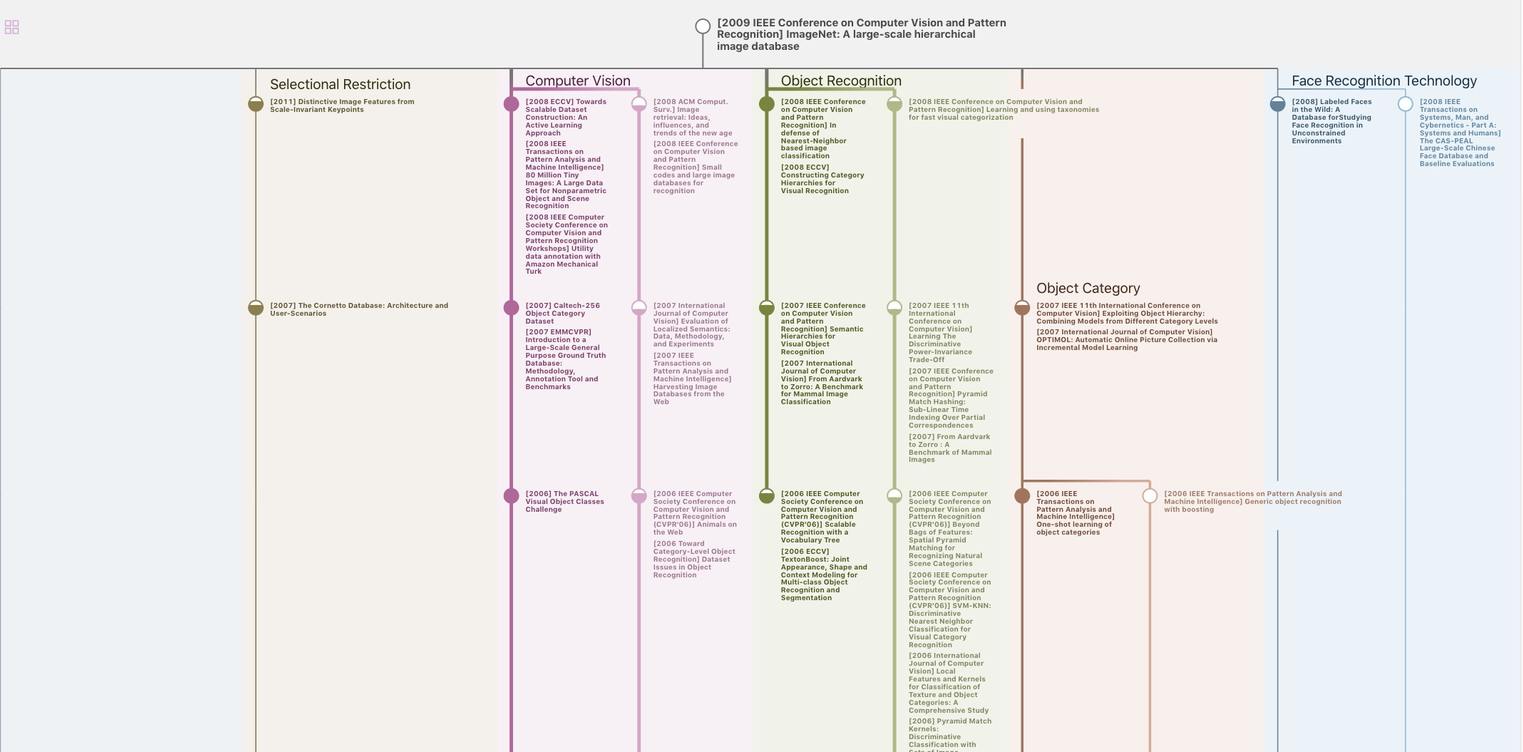
生成溯源树,研究论文发展脉络
Chat Paper
正在生成论文摘要