Mitigating viewpoint sensitivity of self-supervised one-class classifiers
Information Sciences(2022)
摘要
Recent studies on one-class classification have achieved a remarkable performance by employing the self-supervised classifier that predicts the type of pre-defined geometric transformations applied on in-class images. However, they cannot correctly identify in-class images as in-class at all when the input images have various viewpoints (e.g., translated or rotated images), because their classification-based in-class scores assume that in-class images always have a fixed viewpoint. Pointing out that humans can easily recognize such images having various viewpoints as the same class, in this work, we aim to propose a one-class classifier robust to geometrically-transformed inputs, named as GROC. To this end, we remark that in-class images match better with the in-class transformations than out-of-class images do. We introduce a conformity score indicating how strongly an input image agrees with one of the predefined in-class transformations, then utilize the conformity score with our proposed agreement measures for one-class classification. Our extensive experiments demonstrate that GROC is able to accurately distinguish in-class images from out-of-class images regardless of whether the inputs are geometrically-transformed or not, whereas the existing methods fail.
更多查看译文
关键词
One-class classification,Robustness,Viewpoint sensitivity,Geometrically-transformed images
AI 理解论文
溯源树
样例
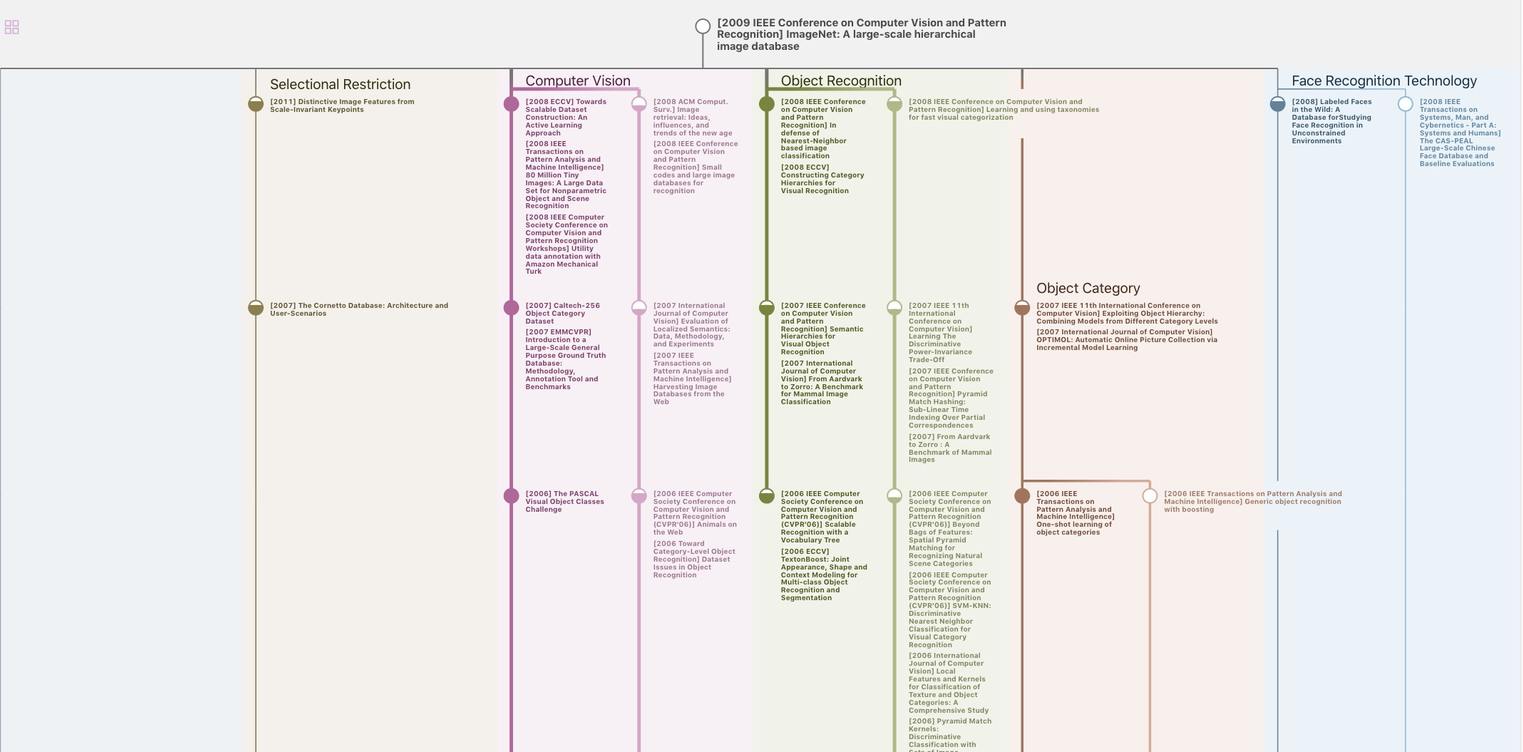
生成溯源树,研究论文发展脉络
Chat Paper
正在生成论文摘要