A new self-paced learning method for privilege-based positive and unlabeled learning
Information Sciences(2022)
摘要
Positive and unlabeled learning (PU learning) is a kind of problem whose goal is learning a two-classes classifier with little proportion of positive samples and numerous unlabeled samples. A series of studies focus on how to extract most likely negative samples from the unlabeled samples, and then train a classifier with the labeled samples as supervised learning. Previous PU learning methods always ignore the additional information called privileged information, which is just provided during the training process while unavailable during testing. In this paper, we propose a novel self-paced algorithm for PU learning with privileged information (SPUPI). The proposed SPUPI extracts some reliable negative samples from unlabeled samples at first, and then generates weights for the unlabeled samples according to the similarity with each class. After that, it builds a more accurate classifier based on privileged information and similarity weights by self-paced learning. By taking the self-paced learning into training, we can build the model with a few labeled samples from easy to complex. We also solve the problem by transforming the primal problem of the proposed model into its dual problem and achieving the PU classifier. Various experiments on the practical datasets indicate that the SPUPI has a better performance compared with previous methods.
更多查看译文
关键词
PU learning,Self-paced learning,Privileged information
AI 理解论文
溯源树
样例
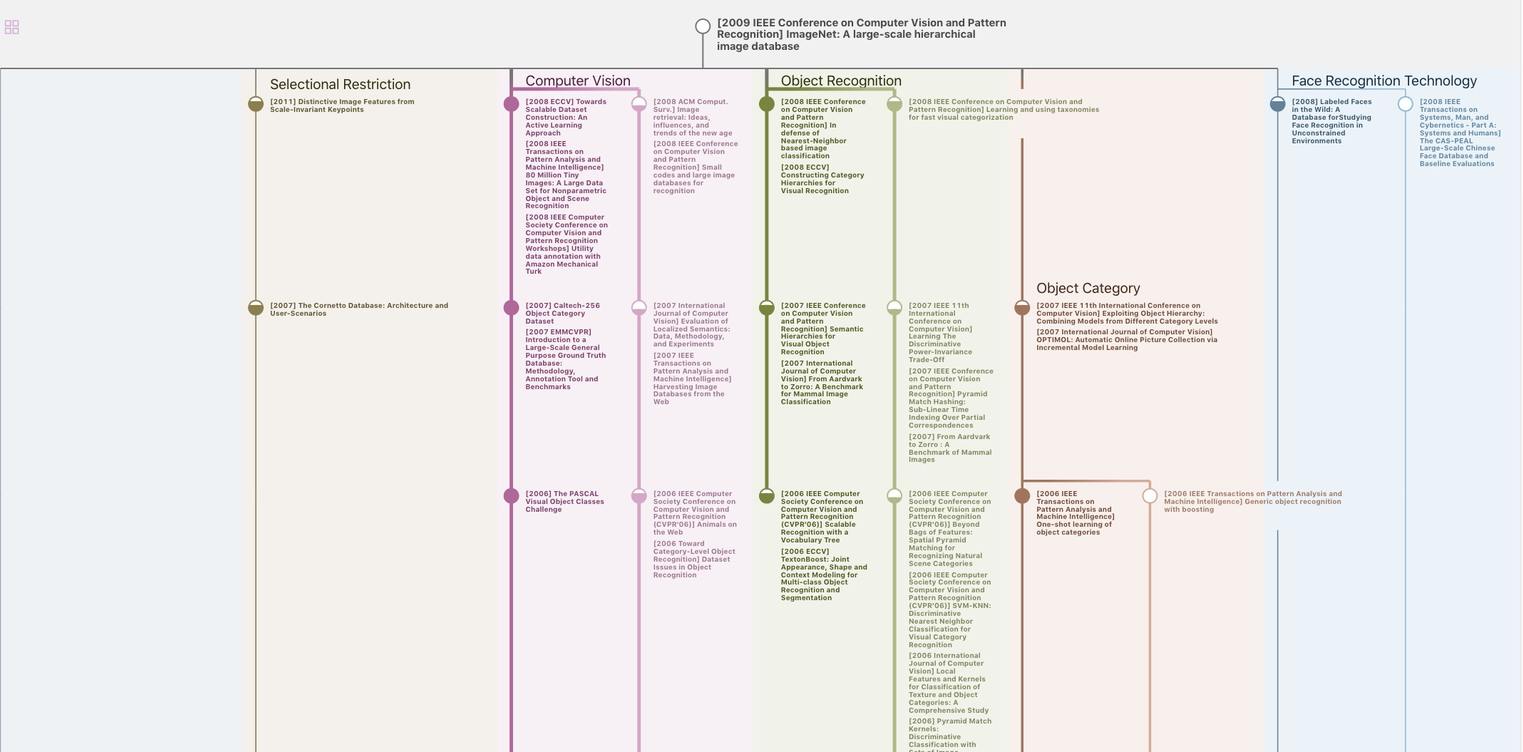
生成溯源树,研究论文发展脉络
Chat Paper
正在生成论文摘要