Detach and Attach: Stylized Image Captioning without Paired Stylized Dataset
Proceedings of the 30th ACM International Conference on Multimedia(2022)
摘要
ABSTRACTStylized Image Captioning aims to generate captions with accurate image content and stylized elements simultaneously. However, large-scaled image and stylized caption pairs cost lots of resources and are usually unavailable. Therefore, it's a challenge to generate stylized captions without paired stylized caption dataset. Previous work on controlling the style of generated captions in an unsupervised way can be divided into two ways: implicitly and explicitly. The former mainly relies on a well-trained language model to capture style knowledge, which is limited to a single style and hard to handle multi-style task. Thus, the latter uses extra style constraints such as outlined style labels or stylized words extracted from stylized sentences to control the style rather than the trained style-specific language model. However, certain styles, such as humorous and romance, are implied in the whole sentence, instead of in some words of a sentence. To address the problems above, we propose a two-step method based on Transformer: firstly detach style representations from large-scaled stylized text-only corpus to provide more holistic style supervision, and secondly attach the style representations to image content to generate stylized captions. We learn a shared image-text space to narrow the gap between the image and the text modality for better attachment. Due to the trade-off between semantics and style, we explore three injection methods of style representations to balance two requirements of image content preservation and stylization. Experiments show that our method outperforms the state-of-the-art systems in overall performance, especially on implied styles.
更多查看译文
关键词
stylized image captioning,stylized dataset,detach
AI 理解论文
溯源树
样例
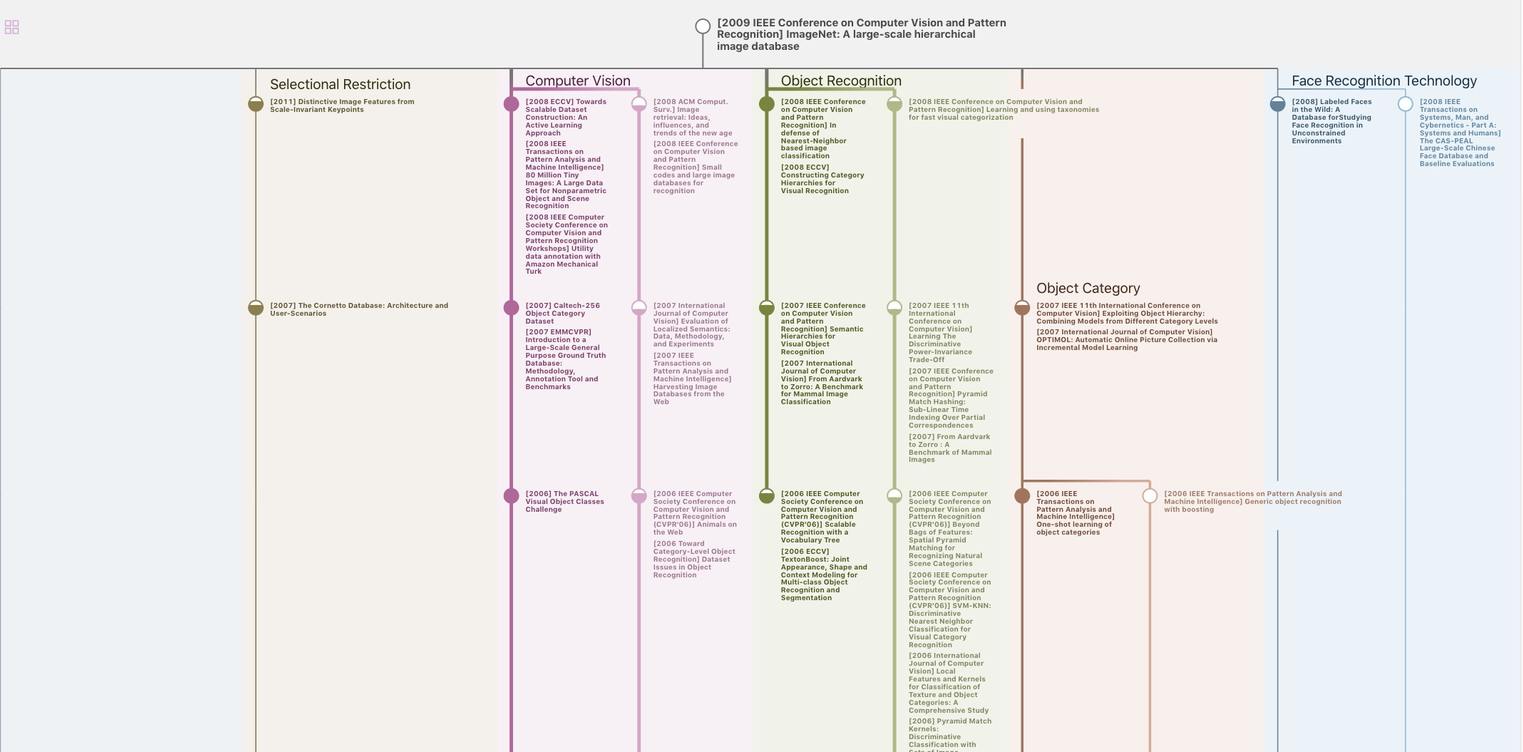
生成溯源树,研究论文发展脉络
Chat Paper
正在生成论文摘要