PairGNNs: Enabling Graph Neural Networks with Pair-Based View
Neural computing & applications(2022)
摘要
Graph neural network (GNN) is widely used in graph structure data and has achieved specific effects in downstream tasks such as node classification. Most existing GNN approaches are designed with a node-based view and homophily assumption during learning. They mainly preserve the similarity between a node and its surrounding context by intensifying low-frequency information in between. The main drawback of such node-view is its lack of support for expressing the compound relationships between nodes. The lack of discrimination of closed nodes makes it hard to handle tasks, e.g., edge classification that demands the high-frequency signals between connected nodes. To preserve the differences between connected nodes during aggregation, a new view, pairGNNs, is proposed, which replaces the node with a paired structure (two adjacent nodes) as the primary learning and information aggregation unit. Paired Nodes form neighbors based on the same node. Aiming at the “neighbor explosion” situation resulting from those designs, we propose a neighbor sampling strategy that significantly reduces the computational complexity by using k minimum degree paired nodes. We apply this model to three off-the-shelf GNNs: GCN, GAT, and GraphSage. Extensive experiments have been performed on nine different datasets and three different downstream tasks. Results prove that GNNs with a paired-view can significantly improve performance compared to their node-view peers and outperform seven strong compared baselines in major downstream tasks, especially on datasets with complex structures.
更多查看译文
关键词
Graph Neural Networks,Pair-based View,Homophily and heterophily network,Neighbor Sampling
AI 理解论文
溯源树
样例
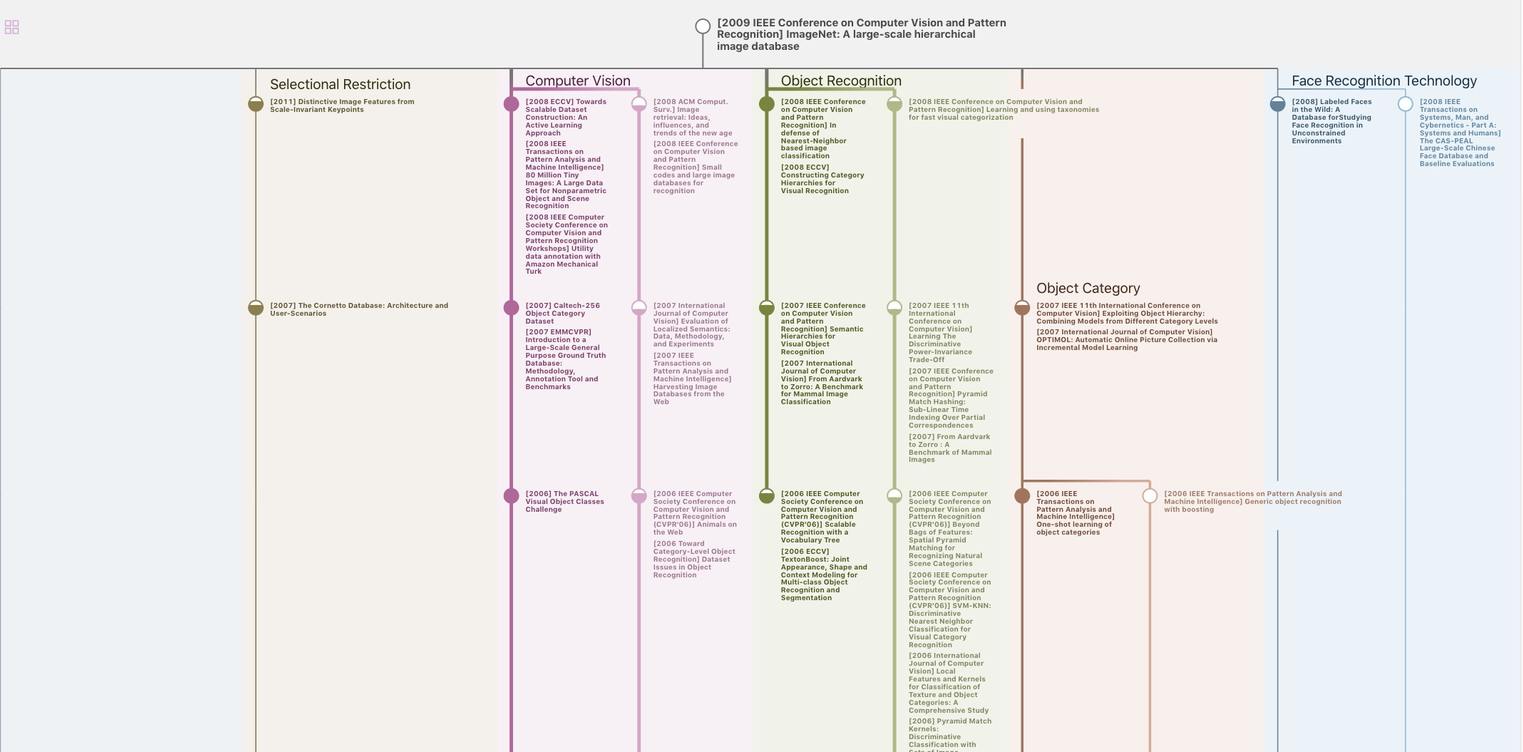
生成溯源树,研究论文发展脉络
Chat Paper
正在生成论文摘要