Structure-Inferred Bi-level Model for Underwater Image Enhancement
Proceedings of the 30th ACM International Conference on Multimedia(2022)
摘要
ABSTRACTVery recently, with the development of underwater robots, underwater image enhancement arising growing interests in the computer vision community. However, owing to light being scattered and absorbed while it traveling in water, underwater captured images often suffer from color cast and low visibility. Existing methods depend on specific prior knowledge and training data to enhance underwater images in the absence of structure information, which results in poor and unnatural performance. To this end, we propose a Structural-Inferred Bi-level Model (SIBM) that incorporates different modalities of knowledge (i.e., semantic domain, gradient-domain, and pixel domain) hierarchically enhancing underwater images. In particular, by introducing a semantic mask, we individually optimize the forehand branch that avoids unnecessary interference arising from the background region. We design a gradient-based high-frequency branch to exploit gradient-space guidance for preserving texture structures. Moreover, we construct a pixel-based branch by feeding semantic and gradient information to enhance underwater images. To exploit different modalities, we introduce a hyper-parameter optimization scheme to fuse the above domain information. Experimental results illustrate that the developed method not only outperforms the previous methods in quantitative scores but also generalizes well on real-world underwater datasets. Source code is available at \hrefhttps://github.com/IntegralCoCo/SIBM https://github.com/IntegralCoCo/SIBM.
更多查看译文
关键词
underwater image enhancement,structure-inferred,bi-level
AI 理解论文
溯源树
样例
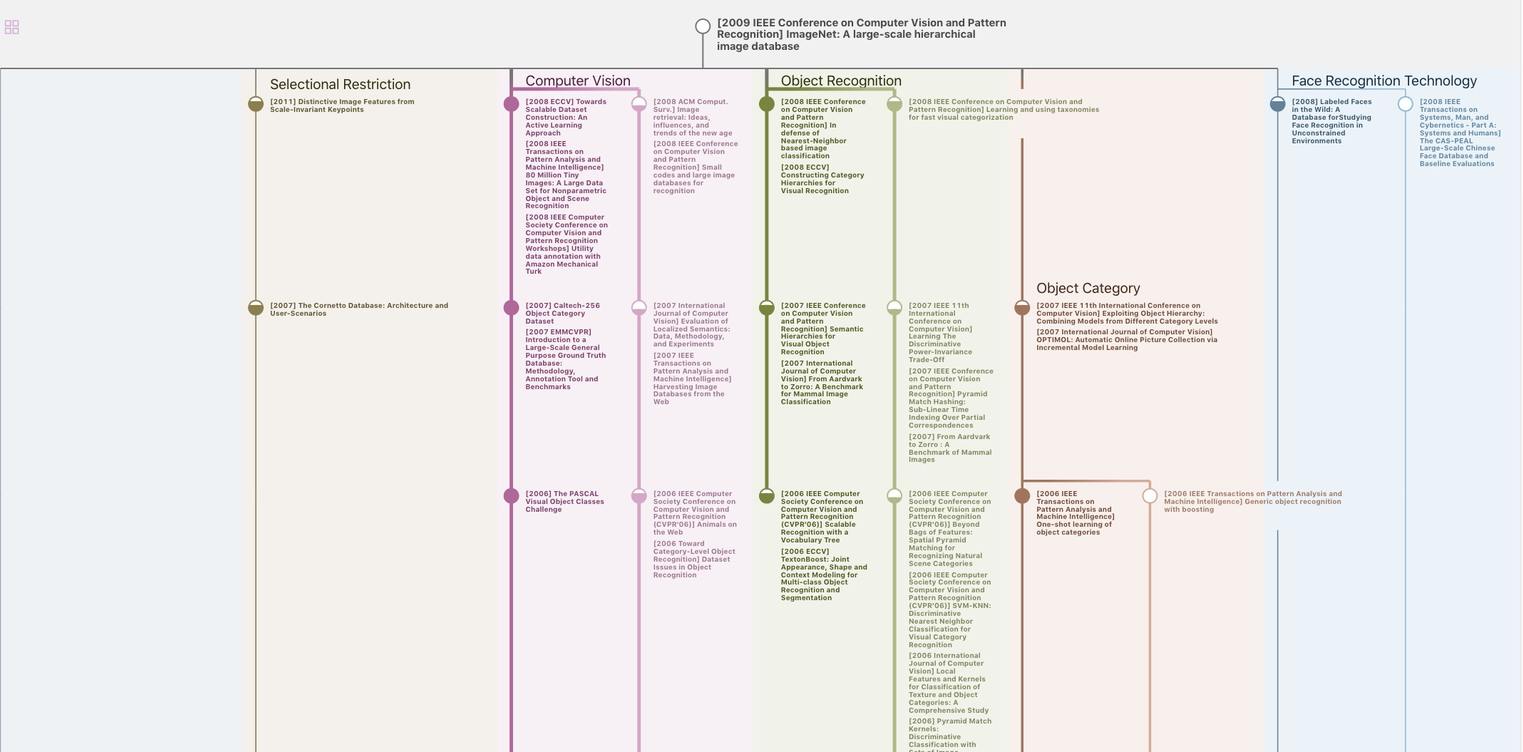
生成溯源树,研究论文发展脉络
Chat Paper
正在生成论文摘要