DP-RBAdaBound: A Differentially Private Randomized Block-Coordinate Adaptive Gradient Algorithm for Training Deep Neural Networks
Expert systems with applications(2023)
摘要
In order to rapidly train deep learning models, many adaptive gradient methods have been proposed in recent years, such as Adam and AMSGrad. However, the computation of the full gradient vectors in the above methods becomes expensive prohibitively at each iteration for handling high dimensional data. Moreover, the private information may be leaked in the process of training. For these reasons, we propose a differentially private randomized block-coordinate adaptive gradient algorithm, called DP-RBAdaBound, for training deep learning model. To reduce the computation of the full gradient vectors, we randomly choose a block-coordinate to update the model parameter at each iteration. Meanwhile, we add the Laplace noise to the block-coordinate of the gradient vector per iteration for preserving the privacy of users. Furthermore, we rigorously show that the proposed algorithm can preserve ϵ-differential privacy, where ϵ>0 denotes the privacy level. Moreover, we also rigorously prove that the square-root regret bound is also achieved in convex settings, i.e., O(T), where T is a time horizon. Besides, we offer a tradeoff between regret bound and privacy, i.e., the regret bound has order of O(1/ϵ4) by fixing other parameters when ϵ-differential privacy is achieved. Finally, we confirm the computational benefit by training DenseNet-121 and ResNet-34 models on CIFAR-10 dataset, respectively. Meanwhile, the effectiveness of DP-RBAdaBound is also validated through training the DenseNet-121 model on CIFAR-100 dataset and LSTM model on Penn TreeBank dataset, respectively.
更多查看译文
关键词
Adaptive gradient methods,Deep learning models,Differential privacy,Randomized block-coordinate
AI 理解论文
溯源树
样例
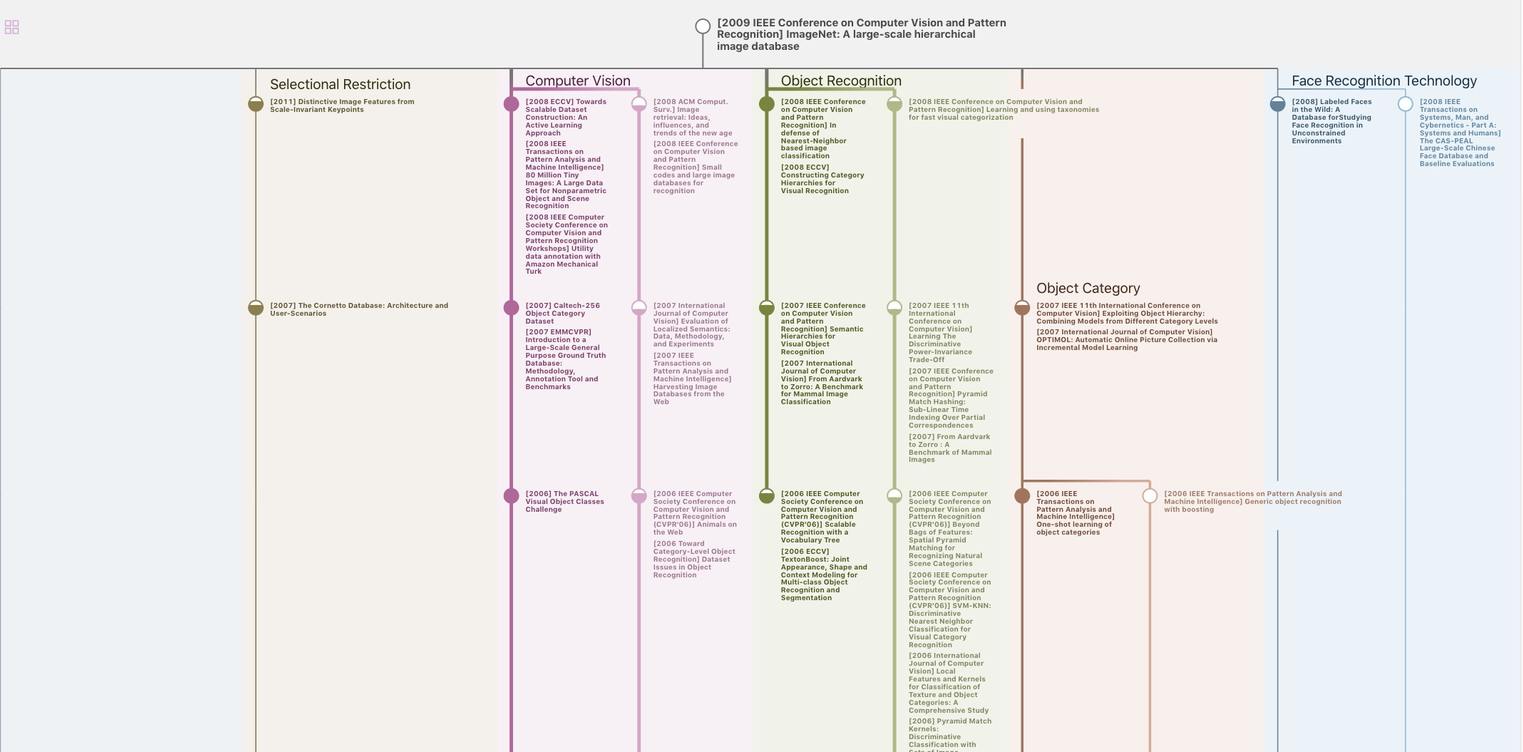
生成溯源树,研究论文发展脉络
Chat Paper
正在生成论文摘要