Is margin all you need? An extensive empirical study of active learning on tabular data
arxiv(2022)
摘要
Given a labeled training set and a collection of unlabeled data, the goal of active learning (AL) is to identify the best unlabeled points to label. In this comprehensive study, we analyze the performance of a variety of AL algorithms on deep neural networks trained on 69 real-world tabular classification datasets from the OpenML-CC18 benchmark. We consider different data regimes and the effect of self-supervised model pre-training. Surprisingly, we find that the classical margin sampling technique matches or outperforms all others, including current state-of-art, in a wide range of experimental settings. To researchers, we hope to encourage rigorous benchmarking against margin, and to practitioners facing tabular data labeling constraints that hyper-parameter-free margin may often be all they need.
更多查看译文
关键词
active learning
AI 理解论文
溯源树
样例
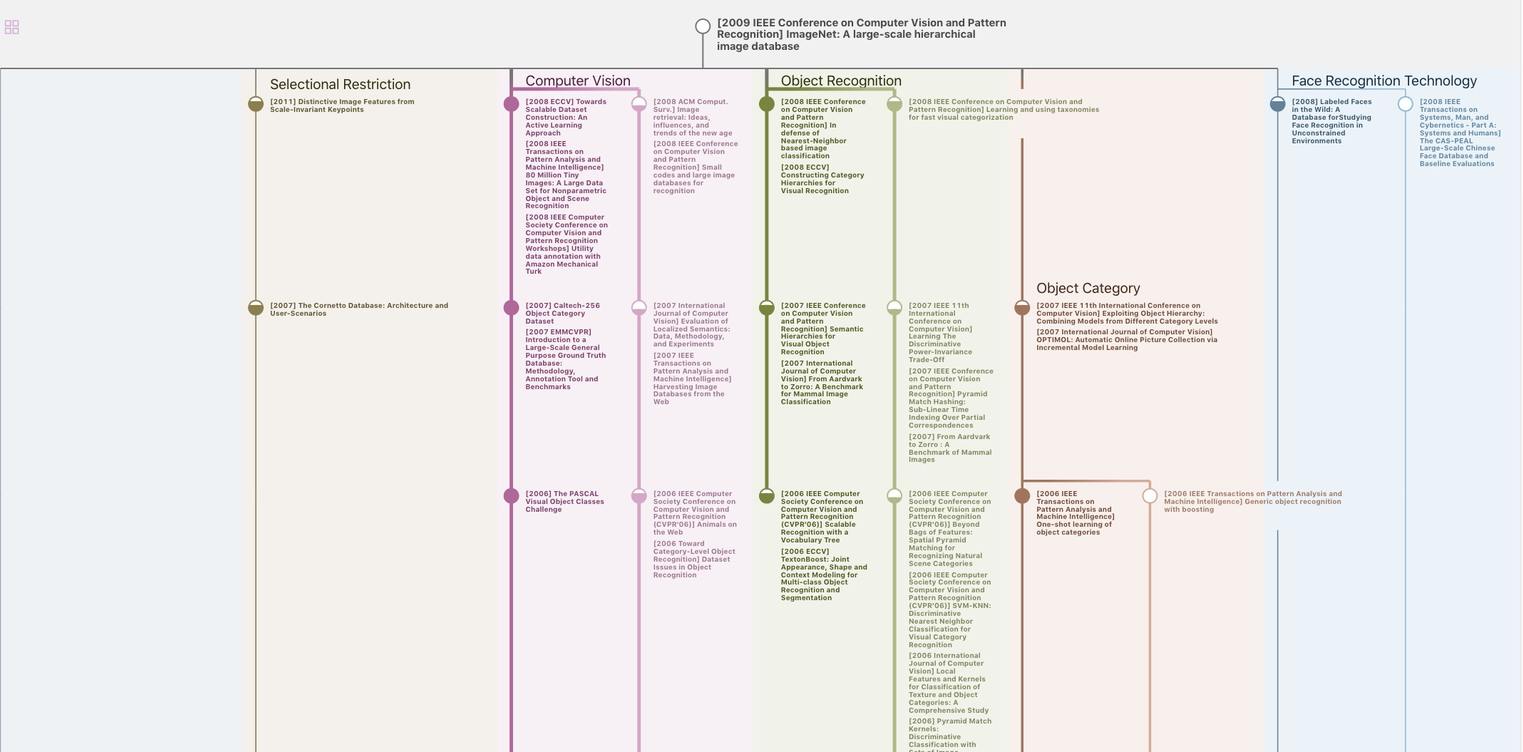
生成溯源树,研究论文发展脉络
Chat Paper
正在生成论文摘要