Decomposed Mutual Information Optimization for Generalized Context in Meta-Reinforcement Learning
arxiv(2022)
摘要
Adapting to the changes in transition dynamics is essential in robotic applications. By learning a conditional policy with a compact context, context-aware meta-reinforcement learning provides a flexible way to adjust behavior according to dynamics changes. However, in real-world applications, the agent may encounter complex dynamics changes. Multiple confounders can influence the transition dynamics, making it challenging to infer accurate context for decision-making. This paper addresses such a challenge by Decomposed Mutual INformation Optimization (DOMINO) for context learning, which explicitly learns a disentangled context to maximize the mutual information between the context and historical trajectories, while minimizing the state transition prediction error. Our theoretical analysis shows that DOMINO can overcome the underestimation of the mutual information caused by multi-confounded challenges via learning disentangled context and reduce the demand for the number of samples collected in various environments. Extensive experiments show that the context learned by DOMINO benefits both model-based and model-free reinforcement learning algorithms for dynamics generalization in terms of sample efficiency and performance in unseen environments.
更多查看译文
关键词
Reinforcement Learning,Dynamics Generalization,Meta Reinforcement Learning
AI 理解论文
溯源树
样例
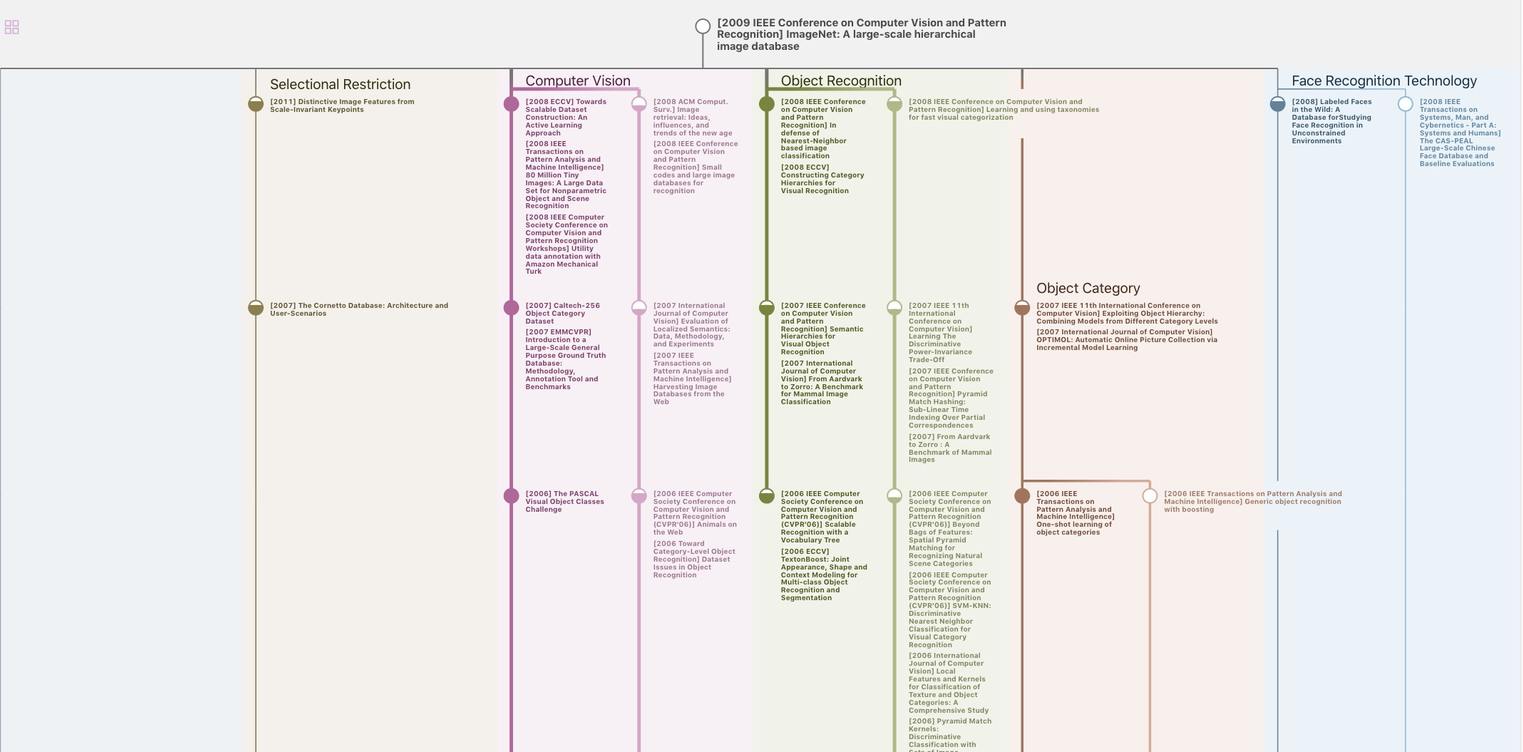
生成溯源树,研究论文发展脉络
Chat Paper
正在生成论文摘要