Modeling and Mining Multi-Aspect Graphs With Scalable Streaming Tensor Decomposition
arxiv(2022)
摘要
Graphs emerge in almost every real-world application domain, ranging from online social networks all the way to health data and movie viewership patterns. Typically, such real-world graphs are big and dynamic, in the sense that they evolve over time. Furthermore, graphs usually contain multi-aspect information i.e. in a social network, we can have the "means of communication" between nodes, such as who messages whom, who calls whom, and who comments on whose timeline and so on. How can we model and mine useful patterns, such as communities of nodes in that graph, from such multi-aspect graphs? How can we identify dynamic patterns in those graphs, and how can we deal with streaming data, when the volume of data to be processed is very large? In order to answer those questions, in this thesis, we propose novel tensor-based methods for mining static and dynamic multi-aspect graphs. In general, a tensor is a higher-order generalization of a matrix that can represent high-dimensional multi-aspect data such as time-evolving networks, collaboration networks, and spatio-temporal data like Electroencephalography (EEG) brain measurements. The thesis is organized in two synergistic thrusts: First, we focus on static multi-aspect graphs, where the goal is to identify coherent communities and patterns between nodes by leveraging the tensor structure in the data. Second, as our graphs evolve dynamically, we focus on handling such streaming updates in the data without having to re-compute the decomposition, but incrementally update the existing results.
更多查看译文
关键词
scalable streaming tensor
AI 理解论文
溯源树
样例
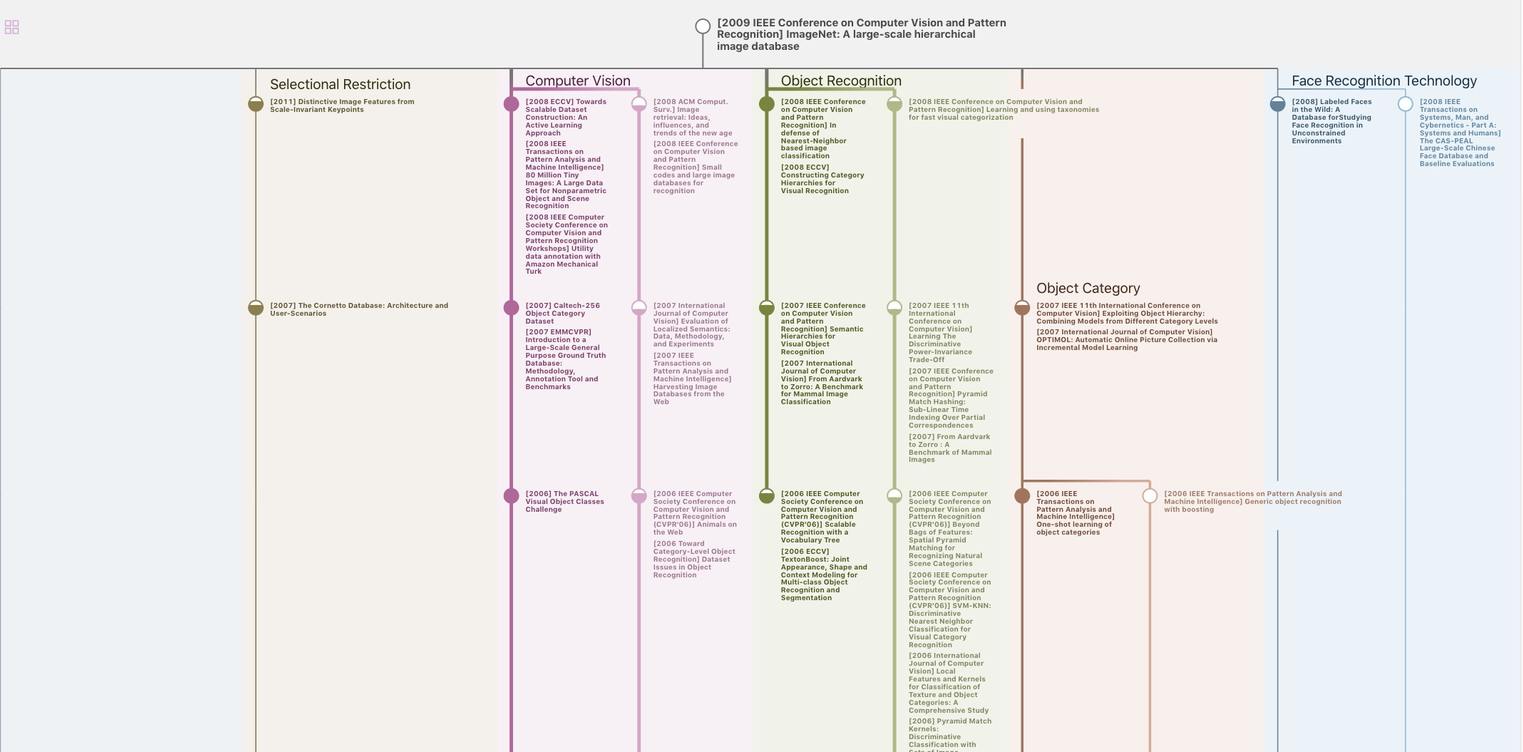
生成溯源树,研究论文发展脉络
Chat Paper
正在生成论文摘要