Improving Continual Relation Extraction through Prototypical Contrastive Learning.
CoRR(2022)
摘要
Continual relation extraction (CRE) aims to extract relations towards the continuous and iterative arrival of new data, of which the major challenge is the catastrophic forgetting of old tasks. In order to alleviate this critical problem for enhanced CRE performance, we propose a novel Continual Relation Extraction framework with Contrastive Learning, namely CRECL, which is built with a classification network and a prototypical contrastive network to achieve the incremental-class learning of CRE. Specifically, in the contrastive network a given instance is contrasted with the prototype of each candidate relations stored in the memory module. Such contrastive learning scheme ensures the data distributions of all tasks more distinguishable, so as to alleviate the catastrophic forgetting further. Our experiment results not only demonstrate our CRECL's advantage over the state-of-the-art baselines on two public datasets, but also verify the effectiveness of CRECL's contrastive learning on improving CRE performance.
更多查看译文
关键词
continual relation extraction,prototypical contrastive,learning
AI 理解论文
溯源树
样例
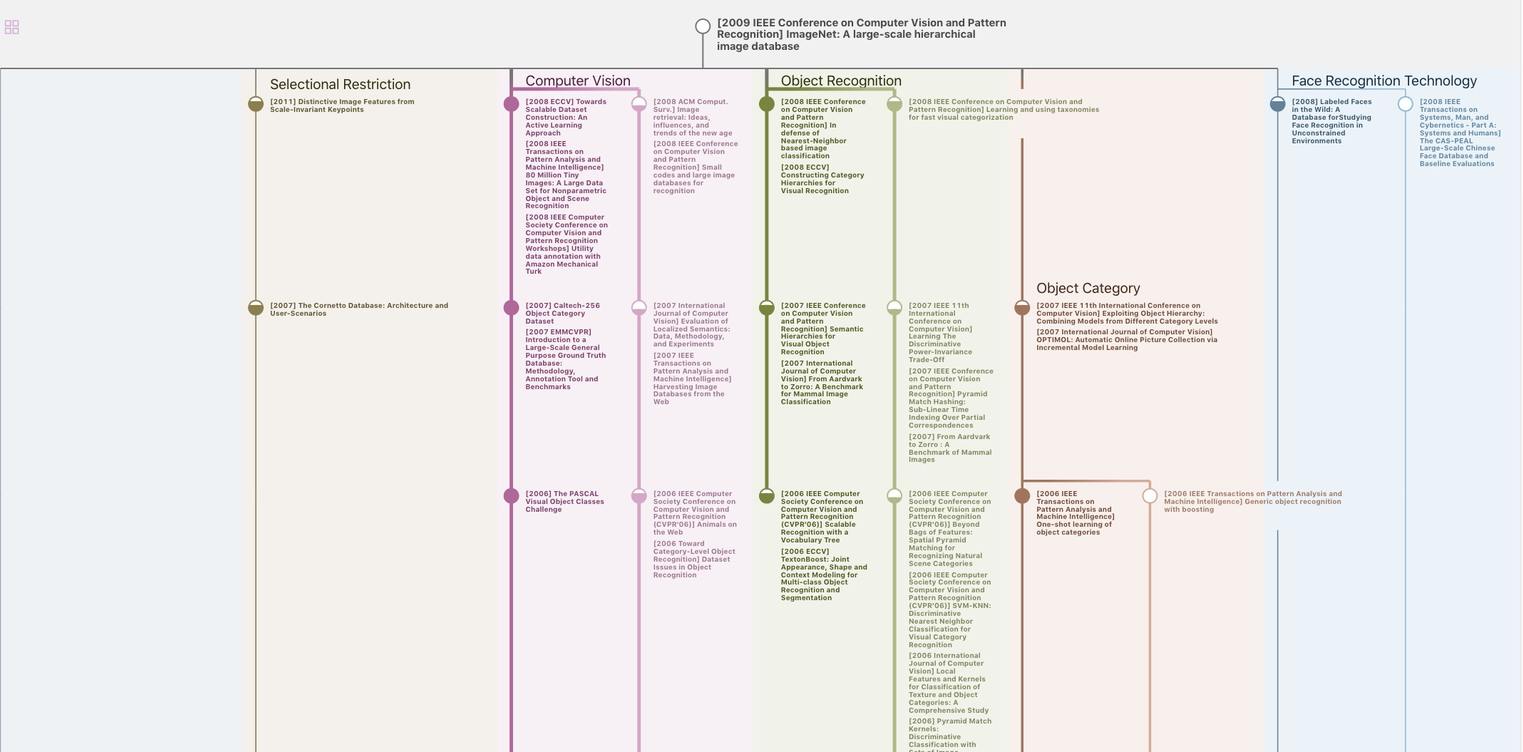
生成溯源树,研究论文发展脉络
Chat Paper
正在生成论文摘要