ParaDime: A Framework for Parametric Dimensionality Reduction.
CoRR(2022)
摘要
ParaDime is a framework for parametric dimensionality reduction (DR). In parametric DR, neural networks are trained to embed high-dimensional data items in a low-dimensional space while minimizing an objective function. ParaDime builds on the idea that the objective functions of several modern DR techniques result from transformed inter-item relationships. It provides a common interface to specify these relations and transformations and to define how they are used within the losses that govern the training process. Through this interface, ParaDime unifies parametric versions of DR techniques such as metric MDS, t-SNE, and UMAP. Furthermore, it allows users to fully customize each aspect of the DR process. We show how this ease of customization makes ParaDime suitable for experimenting with interesting techniques, such as hybrid classification/embedding models or supervised DR, which opens up new possibilities for visualizing high-dimensional data.
更多查看译文
关键词
paradime
AI 理解论文
溯源树
样例
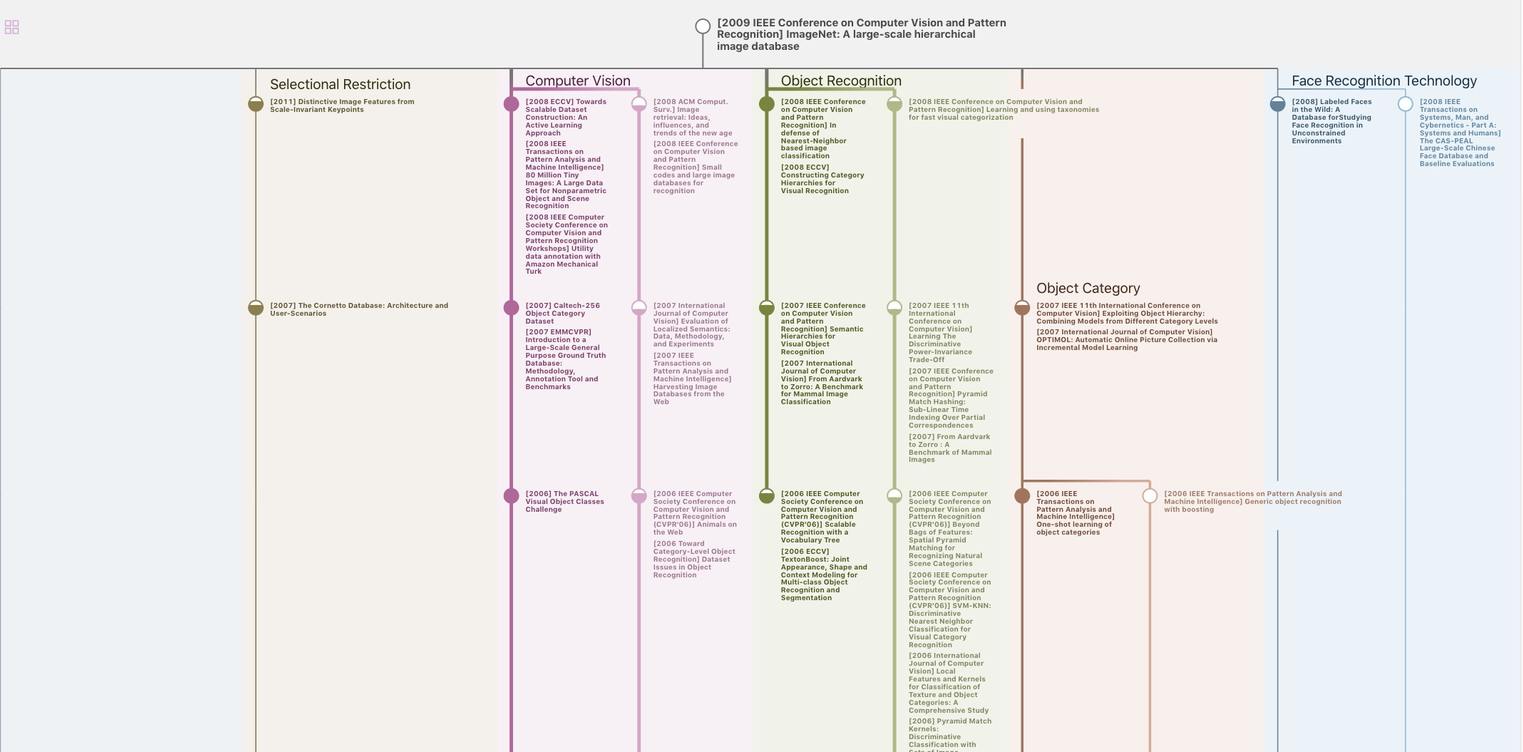
生成溯源树,研究论文发展脉络
Chat Paper
正在生成论文摘要