SampleHST: Efficient On-the-Fly Selection of Distributed Traces
arxiv(2022)
摘要
Since only a small number of traces generated from distributed tracing helps in troubleshooting, its storage requirement can be significantly reduced by biasing the selection towards anomalous traces. To aid in this scenario, we propose SampleHST, a novel approach to sample on-the-fly from a stream of traces in an unsupervised manner. SampleHST adjusts the storage quota of normal and anomalous traces depending on the size of its budget. Initially, it utilizes a forest of Half Space Trees (HSTs) for trace scoring. This is based on the distribution of the mass scores across the trees, which characterizes the probability of observing different traces. The mass distribution from HSTs is subsequently used to cluster the traces online leveraging a variant of the mean-shift algorithm. This trace-cluster association eventually drives the sampling decision. We have compared the performance of SampleHST with a recently suggested method using data from a cloud data center and demonstrated that SampleHST improves sampling performance up to by 9.5x.
更多查看译文
关键词
distributed traces,selection,on-the-fly
AI 理解论文
溯源树
样例
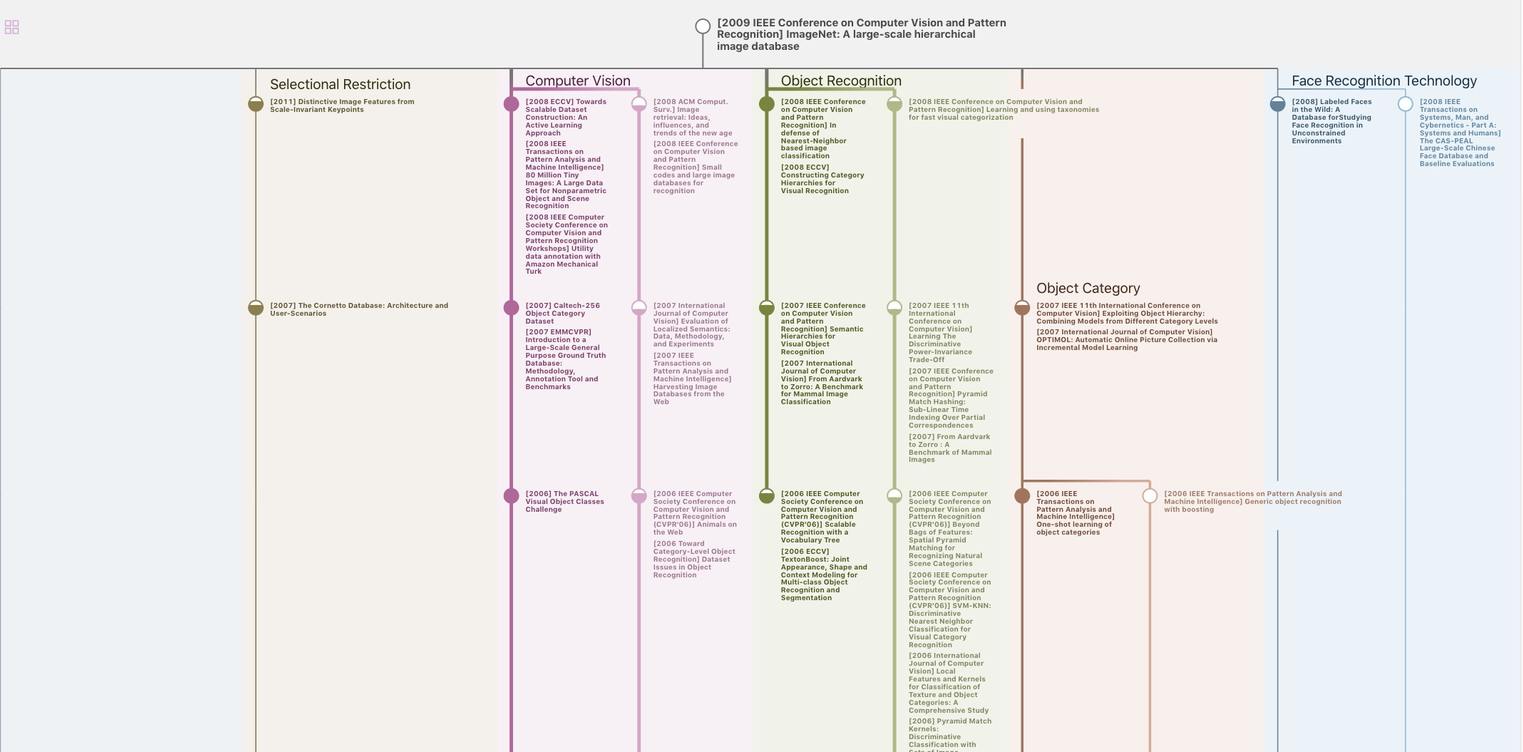
生成溯源树,研究论文发展脉络
Chat Paper
正在生成论文摘要